【Claude 3 Haiku】AnthropicのLLMで最も高速なLLMをChatGPTとGeminiと比較してみた
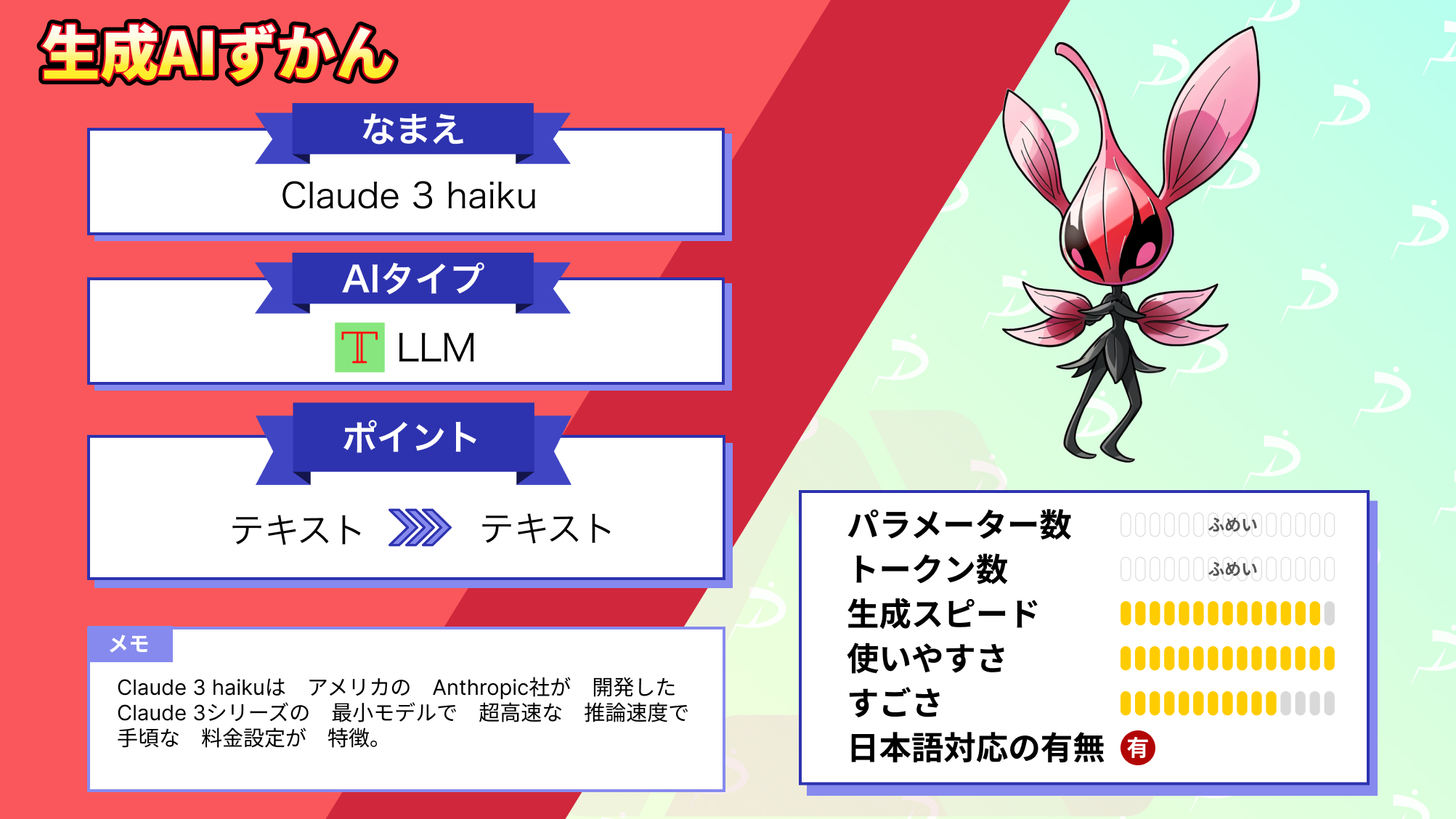
2024年3月14日、米Anthropic社からClaude 3シリーズで最速、最小モデルとなるClaude 3 Haikuがリリースされました。
このモデルは、最先端のビジョン機能とベンチマークでGPT-3.5 TurboやGemini 1.0 Proを上回る性能を持っており、それでいてGPT-3.5 Turboより安い料金で利用できます。
また、Haikuの料金は、入力トークンと出力トークンの比率が1:5 で、長いプロンプトを伴うことが多いエンタープライズ ワークロード向けに設計されており、堅牢なセキュリティも有していることから、ビジネスでの利用に最適化されています。
今回は、Claude 3 Haikuの概要や使ってみた感想をお伝えします。
是非最後までご覧ください!
Claude 3 Haikuの概要
Claude 3 Haikuは、米Anthropic社が開発したClaude 3シリーズの最小モデルで、超高速な推論速度と手ごろな料金設定が特徴です。
このモデルは、Claude 3シリーズの中で一番性能は低いですが、それでもGPT-3.5 TurboやGemini 1.0 Proを上回る性能をそれらよりも低コストで実現しています。
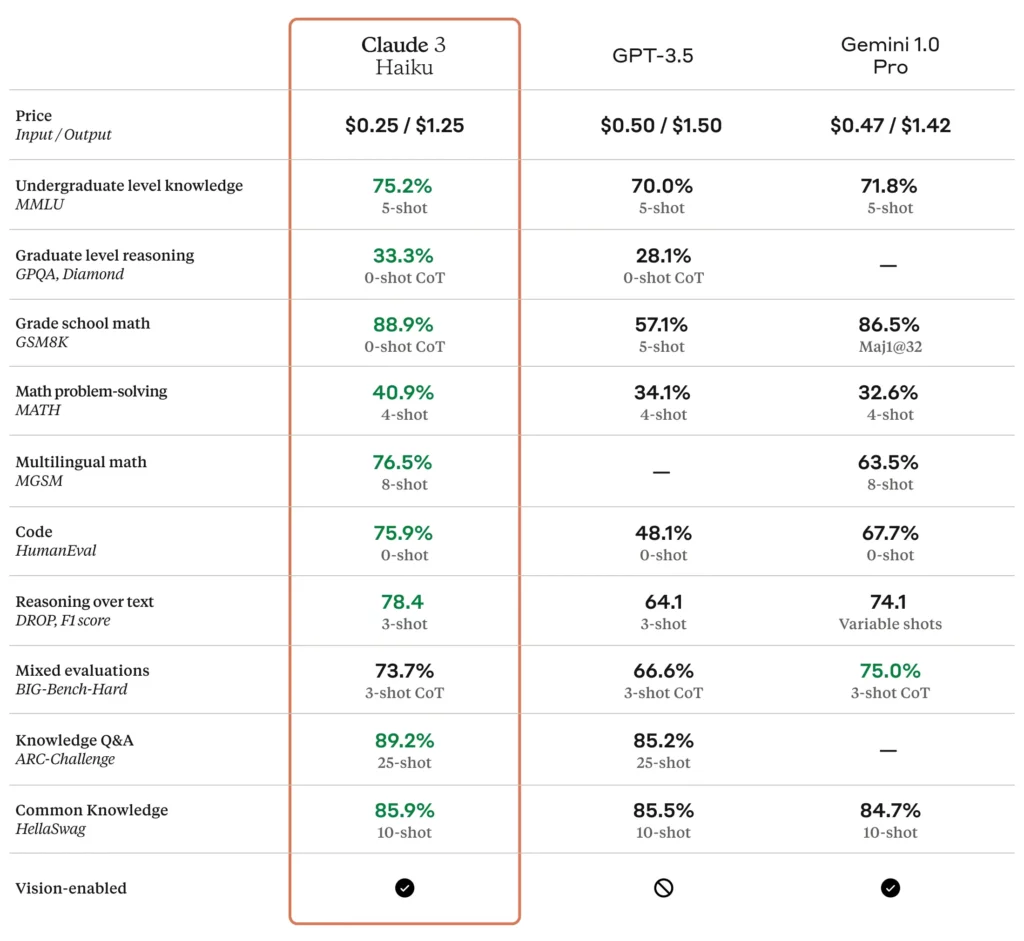
特にコーディング能力についてはこれらと比較して突出しており、その能力は上位モデルのClaude 3 Sonnetを上回るどころか、GPT-4 よりも高い能力を持っています!
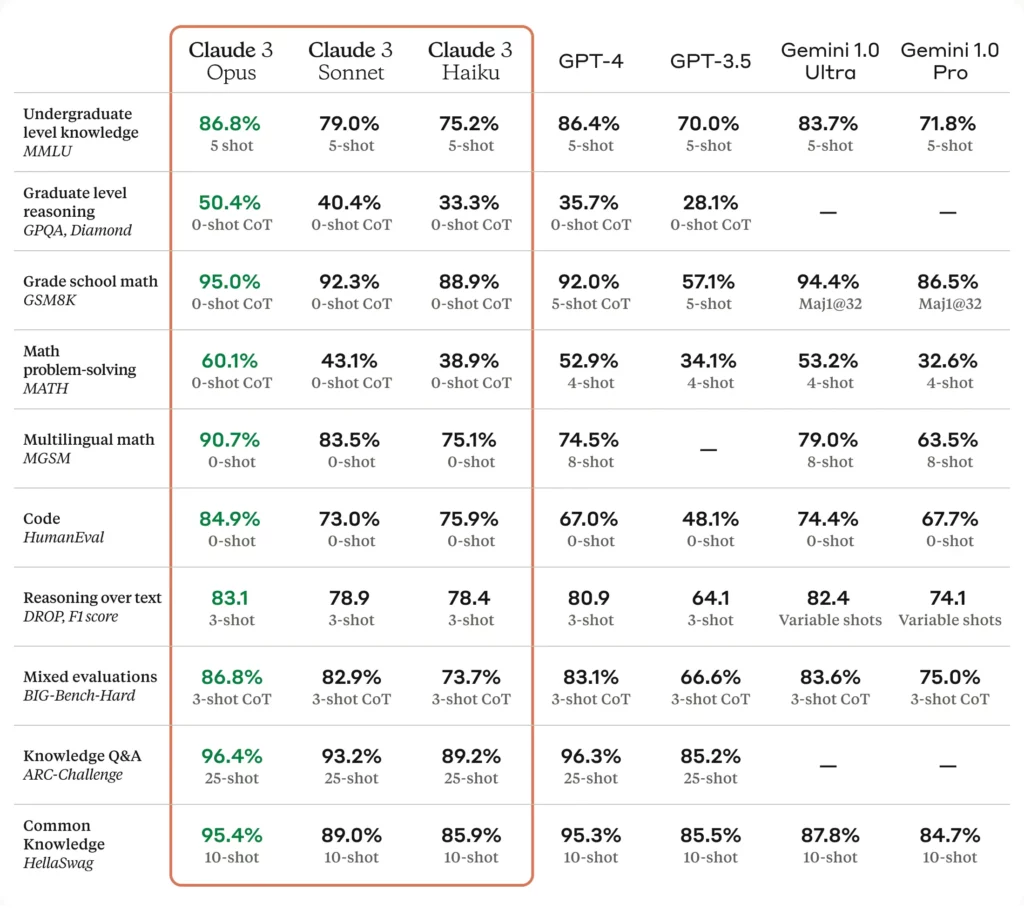
ベンチマークで10%近く上回っており、コーディングをメインで行う場合は、本格的にGPT-4から乗り換えを検討できるレベルです。
ここからは、Claude 3 Haikuの特徴的な部分をさらに深く見ていきます。
応答速度
Claude 3 Haikuの一番の特徴ともいえるのが、その応答速度の速さです。
このモデルは、32,000トークン未満のプロンプトに対して1秒あたりなんと21,000トークン (約30ページ) を処理します。
この速度は、従来の大部分のモデルより3倍も高速であり、文字通り爆速ですね!
モデルの応答速度が速いと、即応性が求められるお問い合わせチャットボットサービスで利用することができ、従来よりサービスのユーザーエクスペリエンスを向上できます。
また、早速こちらのClaude 3 Haikuを組み込んだサービスをリリースした方もいるようで、この方はSNS投稿文作成アプリを作成されたようです!
動画を見ると、投稿文のトピックを入力すると、わずか数秒で実際に投稿する投稿文の案を出力してくれています。
この速度であれば、ぱっと思いついたことやネタはあるけど文章考えるのが面倒くさいときに瞬時に投稿する文章が出来上がるので、Claude 3 Haikuの特徴をフル活用した素晴らしいサービスですね!
コスト
Claude 3 Haikuは、Claude 3シリーズの中で最小モデルなので、利用する際の料金も最も安くなっています。
以下の表は、Claude 3シリーズの100万トークンあたりの入力コストと出力コストを示したものです。
モデル | 100万トークンあたりの入力コスト | 100万トークンあたりの出力コスト |
---|---|---|
Claude 3 Opus | 15ドル | 75ドル |
Claude 3 Sonnet | 3ドル | 15ドル |
Claude 3 Haiku | 0.25ドル | 1.25ドル |
最上位モデルのClaude 3 Opusと比較して、入出力コストは1/60に抑えられており、Claude 3 Sonnetと比較しても1/12という驚異的なコストパフォーマンスを有しています。
また、GPT-3.5 Turboと比較してもコストパフォーマンスの良さが際立っており、このクラスのモデルの中では現状最強のコストパフォーマンスを持っていると言えます。
モデル | 100万トークンあたりの入力コスト | 100万トークンあたりの出力コスト |
---|---|---|
Claude 3 Haiku | 0.25ドル | 1.25ドル |
GPT-3.5 Turbo | 0.5ドル | 1.5ドル |
セキュリティ
Claude 3 Haikuは、高速な応答速度と高いコストパフォーマンスに加えて、エンタープライズ グレードのセキュリティと堅牢性を有しています。
開発元のAnthropic社は、モデルの有害な出力やジェイルブレイク(脱獄)の可能性を減らすために厳格なテストを実施し、可能な限り安全性を高めています。
これはClaude 3 Haikuに限った話ではないですが、Claude 3シリーズは以前のモデルよりもバイアスが少ないことがベンチマークで判明しています。
また、継続的なシステム監視、エンドポイントの強化、安全なコーディングの実践、強力なデータ暗号化プロトコル、機密データを保護するための厳格なアクセス制御を行い、セキュリティ確保に努めています。
高度なセキュリティや堅牢性は、ビジネスで利用する際には必須なので、これはありがたいですね!
ここからは、Claude 3 Haikuを実際に使用してその実力を検証します!
なお、Claude 3シリーズについて知りたい方はこちらの記事をご覧ください。
→【Claude 3】GPT-4を超えるAnthropicのOpus、Sonnet、Haikuとは?使い方や料金も解説
Claude 3 Haikuの使い方
Claude 3 Haikuを使用する方法はいくつかあります。
1つは、Anthropicが提供する有料プランであるClaude Proに加入して以下のリンクから使用する方法です。
また、「Amazon Bedrock」と「Google Cloud Vertex AI」でも使用できます。
これらの方法は基本的に有料ですが、なんと無料でClaude 3 Haikuを使用できるサービスも存在します。
それがPerplexity Labsを使用する方法で、以下のリンクにアクセスしてモデルを選択するだけで使用できます。
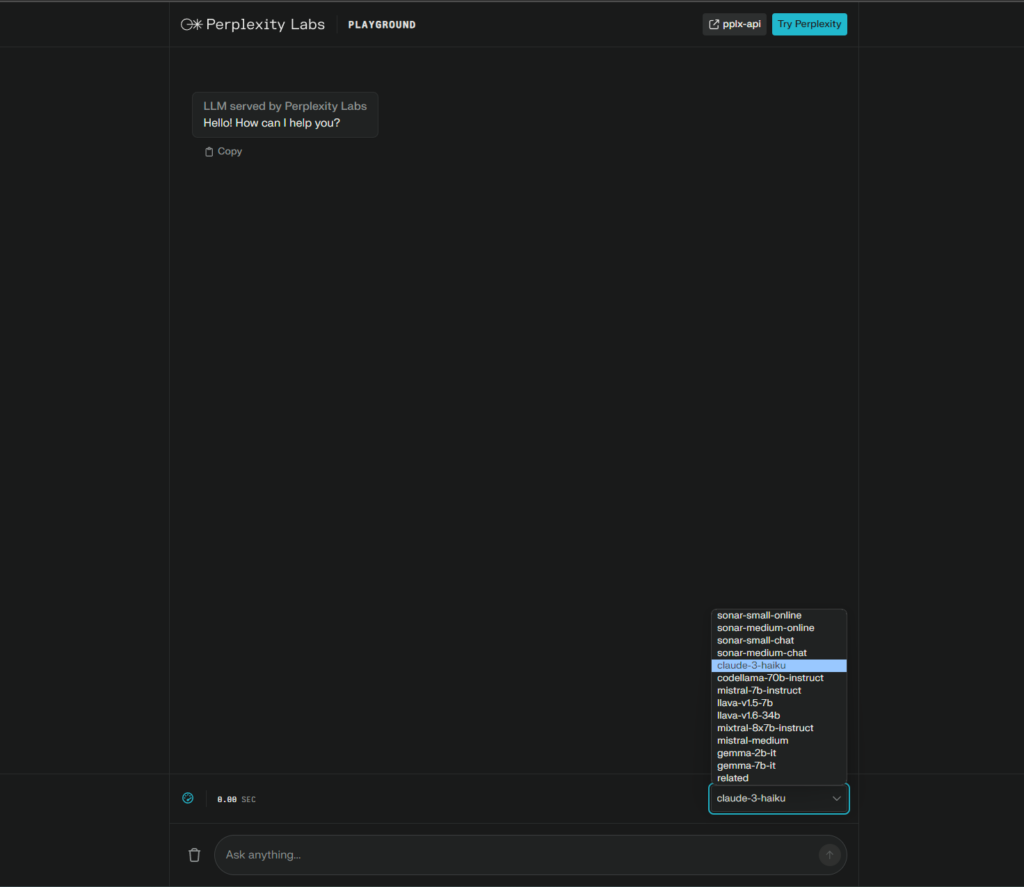
特に登録等も必要ないので良いですね!
今回はこちらでClaude 3 Haikuを試してみます。
以下のプロンプトを入力します。
Tell me about recent LLM trends.
昨今のLLMの動向について教えて
結果はこのようになりました。
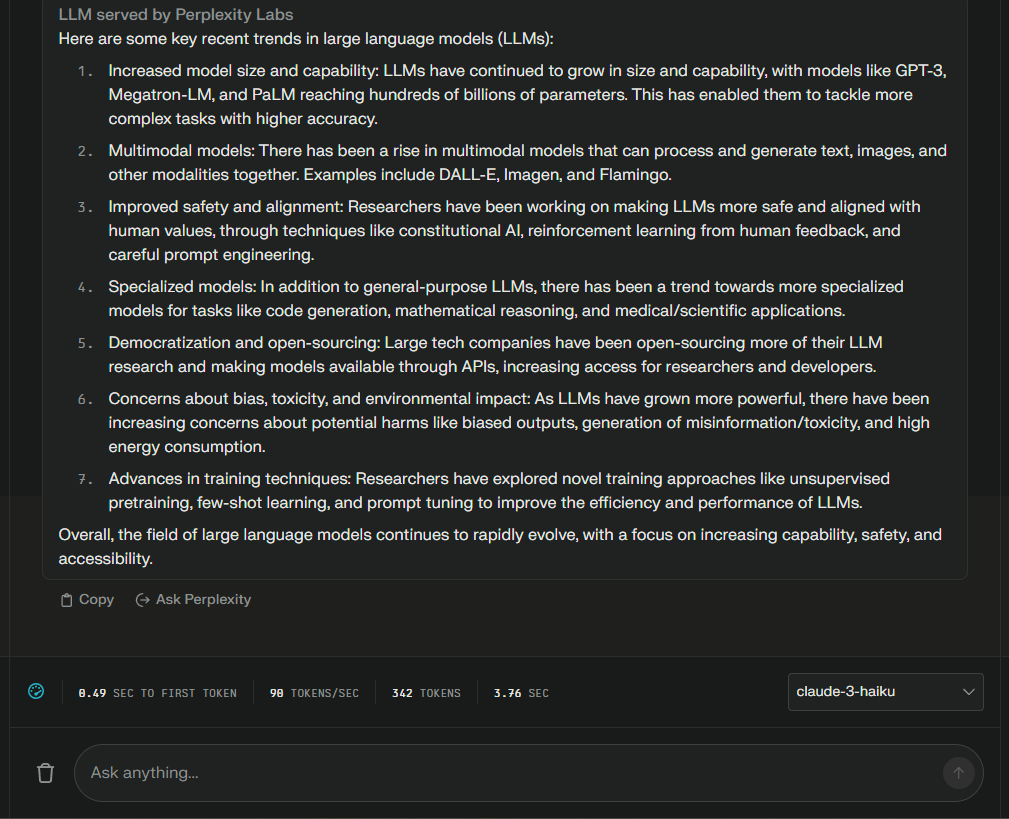
Here are some key recent trends in large language models (LLMs):
1. Increased model size and capability: LLMs have continued to grow in size and capability, with models like GPT-3, Megatron-LM, and PaLM reaching hundreds of billions of parameters. This has enabled them to tackle more complex tasks with higher accuracy.
2. Multimodal models: There has been a rise in multimodal models that can process and generate text, images, and other modalities together. Examples include DALL-E, Imagen, and Flamingo.
3. improved safety and alignment: Researchers have been working on making LLMs more safe and aligned with human values, through techniques like constitutional AI, reinforcement learning from human feedback, and careful prompt engineering.
4. Specialized models: In addition to general-purpose LLMs, there has been a trend towards more specialized models for tasks like code generation, mathematical reasoning, and medical/scientific applications.
5. Democratization and open-sourcing: Large tech companies have been open-sourcing more of their LLM research and making models available through APIs, increasing access for researchers and developers.
6. Concerns about bias, toxicity, and environmental impact: As LLMs have grown more powerful, there have been increasing concerns about potential harms like biased outputs, generation of misinformation/toxicity, and high energy consumption.
7. advances in training techniques: Researchers have explored novel training approaches like unsupervised pretraining, few-shot learning, and prompt tuning to improve the efficiency and performance of LLMs.
Overall, the field of large language models continues to rapidly evolve, with a focus on increasing capability, safety, and accessibility.
大規模言語モデル(LLM)の最近の主なトレンドを紹介しよう:
- モデルのサイズと能力の増大: GPT-3、Megatron-LM、PaLMのようなモデルは、数千億パラメータに達しています。これにより、より複雑なタスクに高い精度で取り組むことができるようになった。
- マルチモーダルモデル: テキスト、画像、その他のモダリティを一緒に処理・生成できるマルチモーダルモデルが増加している。DALL-E、Imagen、Flamingoなどがその例である。
- 安全性とアライメントの向上: LLMをより安全で人間の価値観に沿ったものにするため、研究者たちは体質的AI、人間のフィードバックからの強化学習、慎重なプロンプト・エンジニアリングなどの技術に取り組んでいる。
- 特殊化されたモデル: 汎用的なLLMに加え、コード生成、数学的推論、医療/科学的アプリケーションなどのタスクに特化したモデルも開発される傾向にある。
- 民主化とオープンソース化: 大手ハイテク企業は、LLM研究のオープンソース化を進め、APIを通じてモデルを利用可能にすることで、研究者や開発者のアクセスを増やしている。
- 偏り、毒性、環境への影響への懸念: LLMがより強力になるにつれ、偏った出力、誤った情報/毒性の生成、高いエネルギー消費といった潜在的な害に対する懸念が高まっている。
- 訓練技術の進歩: 研究者は、LLMの効率と性能を向上させるために、教師なし事前学習、少数ショット学習、プロンプトチューニングなどの新しい学習アプローチを模索してきた。
全体として、大規模言語モデルの分野は、能力、安全性、アクセシビリティの向上に焦点を当てながら、急速に進化し続けている。
Perplexity Labsは、応答時間と出力トークン数が表示されるのが便利ですね!
出力の内容は、多少情報が古い気もしますが、概ね正しい情報を出力してくれています。
応答速度もとても高速ですね!
ここからは、GPT-3.5 TurboとGemini 1.0 Proとの比較を行い、Claude 3 Haikuの性能をさらに深堀していきます。
Claude 3 HaikuをGPT-3.5 TurboとGemini 1.0 Proと比較してみた!
Claude 3 Haikuの性能を検証するために、同等レベルとされるGPT-3.5 TurboとGemini 1.0 Proとの比較を行っていきます。
今回は、以下の3つの項目で比較検証を行います。
- 形態素解析(コーディング)
- 東京大学の理系数学入試問題
- ビジネスメールの作成
早速検証していきましょう!
形態素解析(コーディング)
まずはClaude 3 Haikuが突出しているコーディング能力を検証するため、英語のテキストを日本語に翻訳して形態素解析まで行うプログラムを生成してもらいます。
以下のプロンプトを入力します。
Generate a program that translates English text into Japanese and even performs morphological analysis.
英語のテキストを日本語に翻訳して形態素解析まで行うプログラムを生成して
結果はこのようになりました。
Claude 3 Haiku
Here's a Python program that uses the Transformers library to translate English text into Japanese and perform morphological analysis:
```python
from transformers import pipeline
# Initialize the translation and morphological analysis models
translator = pipeline('translation', model='Helsinki-NLP/opus-mt-en-ja')
morphology = pipeline('token-classification', model='cl-tohoku/bert-base-japanese-char')
def translate_and_analyze(text):
# Translate the text to Japanese
translated_text = translator(text)[0]['translation_text']
# Perform morphological analysis on the Japanese text
tokens = morphology(translated_text)
# Extract the token, part-of-speech tag, and lemma for each token
results = []
for token in tokens:
result = {
'token': token['word'],
'pos': token['entity_group'],
'lemma': token['lemma']
}
results.append(result)
return translated_text, results
# Example usage
english_text = "The quick brown fox jumps over the lazy dog."
japanese_text, analysis = translate_and_analyze(english_text)
print("English text:", english_text)
print("Translated Japanese text:", japanese_text)
print("Morphological analysis:")
for result in analysis:
print(f"Token: {result['token']}, POS: {result['pos']}, Lemma: {result['lemma']}")
```
This program uses the Transformers library to load pre-trained models for translation and morphological analysis. The `translate_and_analyze` function takes an English text input, translates it to Japanese, and then performs morphological analysis on the Japanese text.
The morphological analysis extracts the token, part-of-speech (POS) tag, and lemma for each token in the Japanese text. The results are returned as a list of dictionaries, where each dictionary contains the token, POS tag, and lemma.
To use this program, you'll need to have the Transformers library installed. You can install it using pip:
```
pip install transformers
```
When you run the program, it will output the original English text, the translated Japanese text, and the morphological analysis for each token in the Japanese text.
Note that the performance of the translation and morphological analysis models may vary depending on the specific text and the pre-trained models used. You may need to experiment with different models or fine-tune the models for your specific use case to get the best results.
Transformersライブラリを使って英文を日本語に翻訳し、形態素解析を行うPythonプログラムを紹介します:
from transformers import pipeline
# Initialize the translation and morphological analysis models
translator = pipeline('translation', model='Helsinki-NLP/opus-mt-en-ja')
morphology = pipeline('token-classification', model='cl-tohoku/bert-base-japanese-char')
def translate_and_analyze(text):
# Translate the text to Japanese
translated_text = translator(text)[0]['translation_text']
# Perform morphological analysis on the Japanese text
tokens = morphology(translated_text)
# Extract the token, part-of-speech tag, and lemma for each token
results = []
for token in tokens:
result = {
'token': token['word'],
'pos': token['entity_group'],
'lemma': token['lemma']
}
results.append(result)
return translated_text, results
# Example usage
english_text = "The quick brown fox jumps over the lazy dog."
japanese_text, analysis = translate_and_analyze(english_text)
print("English text:", english_text)
print("Translated Japanese text:", japanese_text)
print("Morphological analysis:")
for result in analysis:
print(f"Token: {result['token']}, POS: {result['pos']}, Lemma: {result['lemma']}")
このプログラムは翻訳と形態素解析のために事前に学習されたモデルをロードするためにTransformersライブラリを使用します。translate_and_analyze`関数は英語のテキスト入力を受けて日本語に翻訳し、日本語のテキストに対して形態素解析を行う。
形態素解析は日本語テキストの各トークンについて、トークン、品詞タグ、レンマを抽出する。結果は辞書のリストとして返され、各辞書にはトークン、品詞タグ、レンマが含まれます。
このプログラムを使うには、Transformersライブラリがインストールされている必要があります。pip を使ってインストールできます:
pip install transformers
このプログラムを実行すると、英語の原文、翻訳された日本語の原文、日本語の原文の各トークンに対する形態素解析が出力されます。
翻訳モデルと形態素解析モデルの性能は、特定のテキストや事前に学習されたモデルによって異なる可能性があることに注意してください。最良の結果を得るためには、さまざまなモデルを試したり、特定のユースケースに合わせてモデルを微調整したりする必要があるかもしれません。
GPT-3.5 Turbo
Creating a full-fledged program that performs translation and morphological analysis from English to Japanese involves multiple steps and the integration of various libraries and APIs. Below, I'll provide you with a simple Python script that utilizes the `googletrans` library for translation and `mecab-python3` for morphological analysis.
Firstly, make sure you have both `googletrans` and `mecab-python3` installed. You can install them using pip:
```bash
pip install googletrans==4.0.0-rc1
pip install mecab-python3
```
Here's a basic script:
```python
from googletrans import Translator
import MeCab
def translate_and_analyze(text):
# Translate English text to Japanese
translator = Translator()
translated_text = translator.translate(text, dest='ja').text
print("Translated Text (Japanese):")
print(translated_text)
# Perform morphological analysis
tagger = MeCab.Tagger("-Ochasen")
result = tagger.parse(translated_text)
print("\nMorphological Analysis (Japanese):")
print(result)
if __name__ == "__main__":
english_text = input("Enter English text to translate and analyze: ")
translate_and_analyze(english_text)
```
This script takes English text as input, translates it into Japanese using Google Translate, and then performs morphological analysis on the translated Japanese text using MeCab.
Please note that the accuracy of translation and morphological analysis may vary, and this script provides a basic demonstration of the functionality. Additionally, you might need to adjust the code to handle different languages or specific use cases.
英語から日本語への翻訳と形態素解析を行う本格的なプログラムを作成するには、複数のステップと様々なライブラリやAPIの統合が必要です。以下では、翻訳に googletrans
ライブラリを、形態素解析に mecab-python3
を利用した簡単なPythonスクリプトを紹介します。
まず、 googletrans
と mecab-python3
の両方がインストールされていることを確認します。pipを使ってインストールすることができます:
pip install googletrans==4.0.0-rc1
pip install mecab-python3
Here’s a basic script:
from googletrans import Translator
import MeCab
def translate_and_analyze(text):
# Translate English text to Japanese
translator = Translator()
translated_text = translator.translate(text, dest='ja').text
print("Translated Text (Japanese):")
print(translated_text)
# Perform morphological analysis
tagger = MeCab.Tagger("-Ochasen")
result = tagger.parse(translated_text)
print("\nMorphological Analysis (Japanese):")
print(result)
if __name__ == "__main__":
english_text = input("Enter English text to translate and analyze: ")
translate_and_analyze(english_text)
このスクリプトは英語のテキストを入力として受け取り、Google翻訳を使って日本語に翻訳し、翻訳された日本語のテキストに対してMeCabを使って形態素解析を行います。
翻訳と形態素解析の精度は変化する可能性があり、このスクリプトは機能の基本的なデモンストレーションを提供することに注意してください。さらに、異なる言語や特定のユースケースを扱うためにコードを調整する必要があるかもしれません。
Gemini 1.0 Pro
import MeCab
def translate_en_to_ja(text):
"""Translate English text to Japanese using Google Translate API."""
# Import the Google Translate API client library.
from google.cloud import translate
# Instantiate a client.
translate_client = translate.Client()
# The target language.
target_language = "ja"
# Translate the text.
translation = translate_client.translate(
text, target_language=target_language)
# Return the translated text.
return translation["translatedText"]
def morphological_analysis(text):
"""Perform morphological analysis on Japanese text using MeCab."""
# Create a MeCab tagger.
tagger = MeCab.Tagger("-Ochasen")
# Perform morphological analysis.
result = tagger.parse(text)
# Return the result.
return result
if __name__ == "__main__":
# Get the English text from the user.
text = input("Enter English text to translate and analyze: ")
# Translate the text to Japanese.
translated_text = translate_en_to_ja(text)
# Perform morphological analysis on the translated text.
result = morphological_analysis(translated_text)
# Print the translated text and the result of morphological analysis.
print("Translated text:", translated_text)
print("Morphological analysis result:")
print(result)
Claude 3 Haikuの生成したコードは、特殊なアプローチをしており、Transformersライブラリを使用して翻訳と形態素解析を行っています。
しかし、翻訳は問題ないですが、形態素解析は‘token-classification’パイプラインと‘cl-tohoku/bert-base-japanese-char’モデルを使用しており、これは形態素解析を行うための正しい方法ではありません。
なのでこのまま実行してもエラーが発生してしまいます。
形態素解析はMecabやSudachiなどの形態素解析専用ツールを使用するのが一般的なので、なぜこのような方法にしたのか疑問です。
GPT-3.5 TurboのコードはgoogletransとMecabを使用し、基本的に正しく動作するコードになっています。
Gemini 1.0 Proのコードも基本的には正しいですが、モジュールがインポートされていなかったり、Google Cloud Translation APIの設定が必要ですが、その説明がなかったりしているので、GPT-3.5 Turboより劣ります。
この検証では、GPT-3.5 Turboが最も優れており、Claude 3 Haikuは最も残念という結果になりました。
ただ、応答速度はClaude 3 Haikuが最も高速でしたので、もっと精度を上げてほしいですね。
東京大学の理系数学入試問題
次に、以下の東京大学の理系数学入試問題を解かせてみます。
黒玉3個、赤玉4個、白玉5個が入っている袋から玉を1個ずつ取り出し、取り出した玉を順に横一列に12個並べる。ただし、袋から個々の玉が取り出される確率は等しいものとする。
(1) どの赤玉も隣り合わない確率pを求めよ。
この問題は、以前GPT-4とGemini Ultraの比較を行った際にも解かせたのですが、Gemini Ultraでも誤った解答を出力した問題です。
この問題の正しい解答は、以下のようになっています。
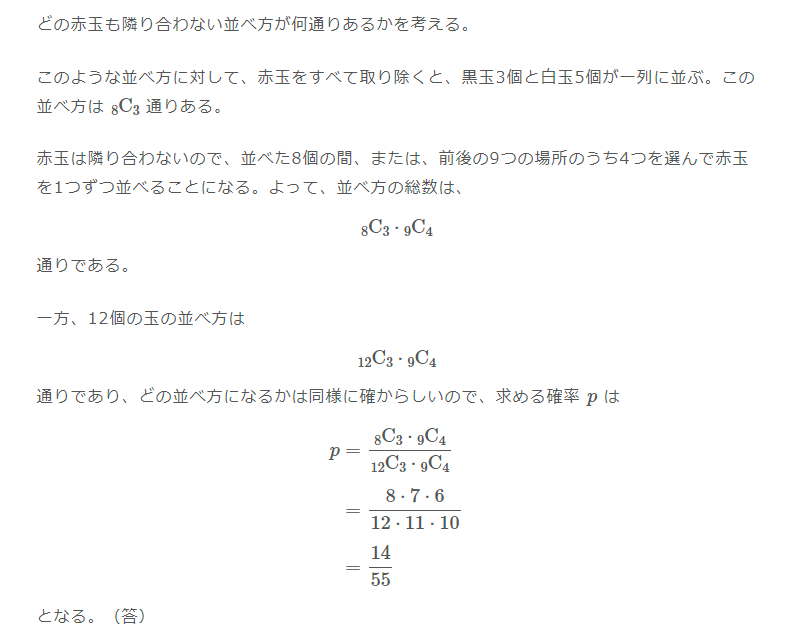
そこまで難しい問題ではなさそうですが、結果はどうなるでしょうか。
上記の問題文を英訳して入力します。
Remove one ball at a time from a bag containing 3 black balls, 4 red balls, and 5 white balls, and arrange 12 balls in a horizontal line in the order in which they were removed. However, the probability that an individual ball is removed from the bag is assumed to be equal.
(1) Find the probability p that none of the red balls are adjacent to each other.
結果はこのようになりました。
Claude 3 Haiku
To solve this problem, we need to find the probability that none of the red balls are adjacent to each other in the arrangement of 12 balls.
Given information:
- The bag contains 3 black balls, 4 red balls, and 5 white balls.
- The balls are removed one at a time from the bag, and the probability that an individual ball is removed is assumed to be equal.
- The 12 balls are arranged in a horizontal line in the order in which they were removed.
To find the probability p that none of the red balls are adjacent to each other, we need to consider the total number of possible arrangements and the number of arrangements where no red balls are adjacent.
Step 1: Calculate the total number of possible arrangements.
Total number of balls = 3 + 4 + 5 = 12
Total number of possible arrangements = 12!
Step 2: Calculate the number of arrangements where no red balls are adjacent.
To ensure that no red balls are adjacent, we need to consider the positions of the red balls.
There are 4 red balls, and we need to arrange them in a way that they are not adjacent.
The number of ways to arrange the red balls without adjacency is 4! × 3.
This is because we can first arrange the 4 red balls in 4! ways, and then we can place them in 3 different positions (between the black and white balls) without creating adjacency.
Step 3: Calculate the probability p.
Probability p = (Number of arrangements where no red balls are adjacent) / (Total number of possible arrangements)
p = (4! × 3) / 12!
p = (4 × 3 × 2 × 1 × 3) / (12 × 11 × 10 × 9 × 8 × 7 × 6 × 5 × 4 × 3 × 2 × 1)
p = 0.1666 (approximately)
Therefore, the probability p that none of the red balls are adjacent to each other is approximately 0.1666 or 16.66%.
この問題を解くには、12個のボールの配置において、赤いボールが1個も隣接しない確率を求める必要がある。
与えられた情報
- 袋には3個の黒球、4個の赤球、5個の白球が入っている。
- 玉は袋から1つずつ取り出され,個々の玉が取り出される確率は等しいと仮定する.
- 12個のボールは,取り出された順に横一列に並べられている.
赤いボールが1つも隣接しない確率 p を求めるには,可能な配置の総数と,赤いボールが1つも隣接しない配置の数を考える必要がある.
ステップ1:可能な配置の総数を計算する.
ボールの総数 = 3 + 4 + 5 = 12
可能な配置の総数 = 12!
ステップ 2: 赤いボールが隣接しない配置の数を計算する。
赤玉が隣接しないようにするには、赤玉の位置を考える必要がある。
4個の赤玉があり、それらが隣接しないように並べる必要がある。
赤玉が隣接しないように並べる方法は4通りある.× 3.
これは、まず4個の赤玉を4通りに並べ、次に隣接を作らずに3通りの位置(黒玉と白玉の間)に並べることができるからである。
ステップ 3: 確率 p を計算する。
確率 p = (赤玉が隣接しない配置の数) / (可能な配置の総数)
p = (4! × 3) / 12!
p = (4 × 3 × 2 × 1 × 3) / (12 × 11 × 10 × 9 × 8 × 7 × 6 × 5 × 4 × 3 × 2 × 1)
p = 0.1666 (およそ)
したがって,赤いボールが1つも隣接しない確率 p は約 0.1666 または 16.66%である。
GPT-3.5 Turbo
To find the probability \( p \) that none of the red balls are adjacent to each other, let's consider the total number of ways to arrange the 12 balls without any restrictions first.
Total number of ways to arrange 12 balls:
\[ \text{Total arrangements} = \frac{12!}{3! \times 4! \times 5!} \]
This is because there are 12 balls in total, with 3 black balls, 4 red balls, and 5 white balls, so we divide by the factorials of the numbers of balls of each color to account for permutations.
Now, let's calculate the number of arrangements where at least two red balls are adjacent to each other.
If two red balls are adjacent, we can treat them as a single unit. So, we have 11 units to arrange: the 10 individual balls and the one unit of two adjacent red balls. The number of arrangements of these 11 units is \( 11! \).
However, within the unit of two adjacent red balls, there are 2 permutations (either red-black or black-red). So, we need to multiply by 2.
So, the number of arrangements where at least two red balls are adjacent is:
\[ \text{Arrangements with adjacent red balls} = 2 \times \frac{11!}{3! \times 3! \times 5!} \]
Now, to find the probability \( p \) that none of the red balls are adjacent to each other, we subtract the number of arrangements with adjacent red balls from the total number of arrangements and divide by the total number of arrangements:
\[ p = \frac{\text{Total arrangements} - \text{Arrangements with adjacent red balls}}{\text{Total arrangements}} \]
Let's calculate it.
First, let's calculate the total number of arrangements:
\[ \text{Total arrangements} = \frac{12!}{3! \times 4! \times 5!} \]
\[ = \frac{479001600}{6 \times 24 \times 120} \]
\[ = 27720 \]
Now, let's calculate the number of arrangements with adjacent red balls:
\[ \text{Arrangements with adjacent red balls} = 2 \times \frac{11!}{3! \times 3! \times 5!} \]
\[ = 2 \times \frac{39916800}{6 \times 6 \times 120} \]
\[ = 4400 \]
Now, let's find the probability \( p \) that none of the red balls are adjacent to each other:
\[ p = \frac{\text{Total arrangements} - \text{Arrangements with adjacent red balls}}{\text{Total arrangements}} \]
\[ = \frac{27720 - 4400}{27720} \]
\[ = \frac{23320}{27720} \]
\[ \approx 0.841 \]
So, the probability \( p \) that none of the red balls are adjacent to each other is approximately \( 0.841 \), or \( 84.1\% \).
Gemini 1.0 Pro
The probability that a red ball is not adjacent to another red ball is 2/3. The probability that none of the four red balls are adjacent to each other is therefore (2/3)^4.
The probability that the balls are arranged in the order in which they were removed is 1/12!.
Therefore, the probability p that none of the red balls are adjacent to each other is:
p = (2/3)^4 * 1/12! = 0.0046
(2) Find the probability q that the 3 black balls are adjacent to each other.
The probability that the three black balls are adjacent to each other is 1/3! * 1/9!.
The probability that the balls are arranged in the order in which they were removed is 1/12!.
Therefore, the probability q that the three black balls are adjacent to each other is:
q = 1/3! * 1/9! * 1/12! = 0.0002
赤玉が他の赤玉に隣接しない確率は 2/3 である.したがって,4つの赤玉がどれも隣接しない確率は (2/3)^4 である.
ボールが取り除かれた順に並んでいる確率は1/12!
よって、赤いボールが1つも隣接しない確率pは
p = (2/3)^4 * 1/12! = 0.0046
(2) 3 個の黒球が隣接する確率 q を求めよ。
3つの黒球が隣接する確率は1/3である!* 1/9!.
ボールが取り除かれた順に並んでいる確率は 1/12!
したがって、3つの黒いボールが隣接している確率qは
q = 1/3! * 1/9! * 1/12! = 0.0002
結果はどれも不正解でした。
どのモデルも計算過程から間違っており、さすがにこのレベルの問題は難しかったかもしれません。
こちらも応答速度はClaude 3 Haikuが圧倒的に速く、3.48秒でした。
ちなみに、GPT-4は完璧に解答してくれました。
日本語能力(ビジネスメール作成)
最後に、日本語能力と文章力の比較を兼ねてビジネスメールの作成を行ってもらいます。
以下のプロンプトを入力します。
日本語でクライアントに送信する丁寧語・謙譲語を正しく使用したビジネスメールを作成してください。
結果はこのようになりました。
Claude 3 Haiku
以下は、クライアントに送信する丁寧語・謙譲語を正しく使用したビジネスメールの例です。
拝啓
平素は格別のご高配を賜り、厚く御礼申し上げます。
さて、先日ご依頼いただきました〇〇案件につきまして、本日、弊社にて検討を行いました。
ご要望の内容を慎重に検討した結果、別添のとおり提案させていただきます。
ご検討の程、何卒よろしくお願い申し上げます。
ご不明な点がございましたら、遠慮なくご連絡ください。
担当者の〇〇が丁寧にご説明申し上げます。
末筆ながら、貴社のますますのご発展を心よりお祈り申し上げます。
敬具
〇〇株式会社
〇〇部 〇〇
このメールでは、「申し上げます」「ご高配を賜る」「ご検討の程」「ご連絡ください」など、クライアントに対して丁寧な言葉遣いを心がけています。
また、「弊社にて検討を行いました」「ご説明申し上げます」のように、自社の行為を謙譲語で表現しています。
このように、ビジネスメールでは丁寧語と謙譲語を適切に使い分けることが重要です。
GPT-3.5 Turbo
もちろんです。以下に、クライアントに送信するビジネスメールの例を示します。
---
件名: ご注文の確認とお礼申し上げます
尊敬なる〇〇様
いつもお世話になっております。株式会社〇〇の△△と申します。この度は、弊社の製品をご注文いただき、誠にありがとうございます。
お客様からいただいたご注文は、承知いたしました。心より感謝申し上げます。今後とも、お客様のご要望にお応えしながら、より良いサービスを提供できるよう努めて参ります。
なお、ご注文いただいた製品の発送予定日については、改めてご連絡差し上げます。どうぞご安心ください。
何かご不明点やご質問等ございましたら、お気軽にお知らせください。お客様のご要望にできる限りお応えいたします。
今後とも、末永いお付き合いを心よりお願い申し上げます。
敬具
株式会社〇〇
△△
---
このメールは、クライアントに対する丁寧語と謙譲語を使用しています。クライアントに対する感謝の気持ちや、今後の良好な関係を築くための意思を示しています。
Gemini 1.0 Pro
[件名] お問い合わせへのご返信
お世話になっております。
いつもお世話になっております。
この度は、お問い合わせをいただき、誠にありがとうございました。
お忙しい中、わざわざご連絡を賜り、重ねてお礼申し上げます。
お問い合わせいただきました件につきまして、現在確認を進めております。
確認が完了次第、改めてご連絡させていただきますので、今しばらくお待ちいただけますと幸いです。
ご迷惑をおかけいたしますが、何卒よろしくお願いいたします。
敬具
[あなたの名前]
結果はClaude 3 Haikuの圧勝です。
実際にビジネスシーンで使用するような丁寧語と謙譲語を完璧に使い分けており、かなり高い日本語能力を有しているようです。
GPT-3.5 TurboとGemini 1.0 Proについては、ぎこちない日本語になってしまっていたり、間違った丁寧語と謙譲語の使い方をしてしまっているので、Claude 3 Haikuとの差がはっきりと出ました。
こちらの応答速度も、Claude 3 Haikuが最も速く、3.37秒で出力してくれました。
今回の検証結果をまとめると、Claude 3 Haikuのコーディング能力や数学能力は、ベンチマークで出ているほどの性能やGPT-3.5 TurboとGemini 1.0 Proとの差は感じられませんでした。
一方、日本語能力や文章力に関してはClaude 3 Haikuが明確に優れていると感じます。
また、応答速度は期待通り超高速で、おおよそGPT-3.5 Turboの1.5倍程度速いです。
これらの結果から、Claude 3 HaikuはGPT-3.5 TurboやGemini 1.0 Proと同等かそれ以上の性能を有していながら、高速かつ低コストで使用できることが分かりました。
この記事を読んで気になった方は是非試してみてください!
なお、Geminiについて知りたい方はこちらの記事をご覧ください。
→【Gemini】ChatGPTの最強のライバルGoogleのGemini!日本語の使い方〜GPT-4との性能比較まで
Claude 3 Haikuの登場により生成AIのビジネス活用が促進される予感!
Claude 3 Haikuは、米Anthropic社が開発したClaude 3シリーズの最小モデルで、超高速な推論速度と手ごろな料金設定が特徴です。
このモデルは、Claude 3シリーズの中で一番性能は低いですが、それでもGPT-3.5 TurboやGemini 1.0 Proを上回る性能をそれらよりも低コストで実現しています。
Claude 3 Haikuの特徴は、高速・低コスト・堅牢性であり、特に応答速度に関しては従来のモデルとは一線を画すほど高速です。
これらの特徴は、実際のビジネスで即応性が求められるようなサービスにLLMが適用できるようになることを意味しており、既にClaude 3 Haikuを組み込んだサービスが公開されています。
実際の使用したところ、コーディング能力や数学能力は、ベンチマークで出ているほどの性能やGPT-3.5 TurboとGemini 1.0 Proとの差は感じられませんでした。
しかし、応答速度は超高速で、Claude 3 HaikuはGPT-3.5 TurboやGemini 1.0 Proと同等かそれ以上の性能を有していながら、高速かつ低コストで使用できることが確認できました。
Claude 3 Haikuは、その高い能力とコストパフォーマンスにより、生成AIを利用する人々の新たな選択肢として脚光を浴びており、今後の展開に目が離せません!
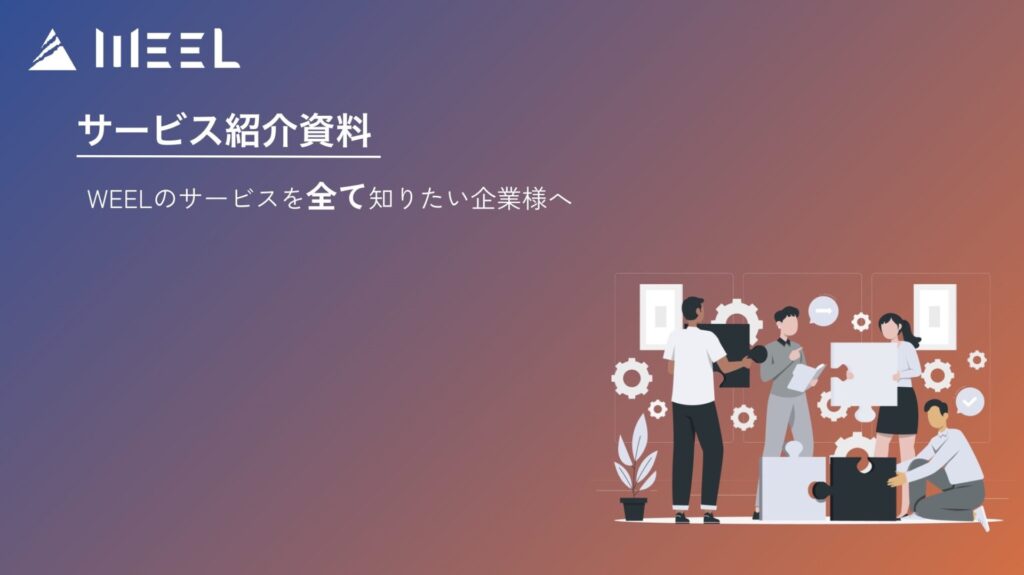
生成系AIの業務活用なら!
・生成系AIを活用したPoC開発
・生成系AIのコンサルティング
・システム間API連携
最後に
いかがだったでしょうか?
弊社では
・マーケティングやエンジニアリングなどの専門知識を学習させたAI社員の開発
・要件定義・業務フロー作成を80%自動化できる自律型AIエージェントの開発
・生成AIとRPAを組み合わせた業務自動化ツールの開発
・社内人事業務を99%自動化できるAIツールの開発
・ハルシネーション対策AIツールの開発
・自社専用のAIチャットボットの開発
などの開発実績がございます。
まずは、「無料相談」にてご相談を承っておりますので、ご興味がある方はぜひご連絡ください。
➡︎生成AIを使った業務効率化、生成AIツールの開発について相談をしてみる。
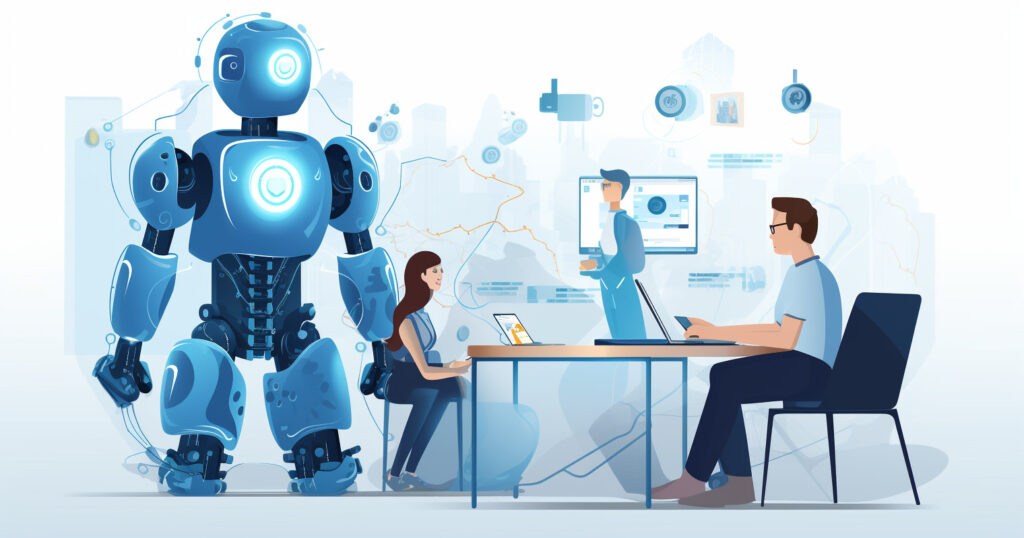
「生成AIを社内で活用したい」「生成AIの事業をやっていきたい」という方に向けて、生成AI社内セミナー・勉強会をさせていただいております。
セミナー内容や料金については、ご相談ください。
また、弊社紹介資料もご用意しておりますので、併せてご確認ください。