【Gemini 1.5 Flash】Geminiシリーズ最速のマルチモーダルLLM
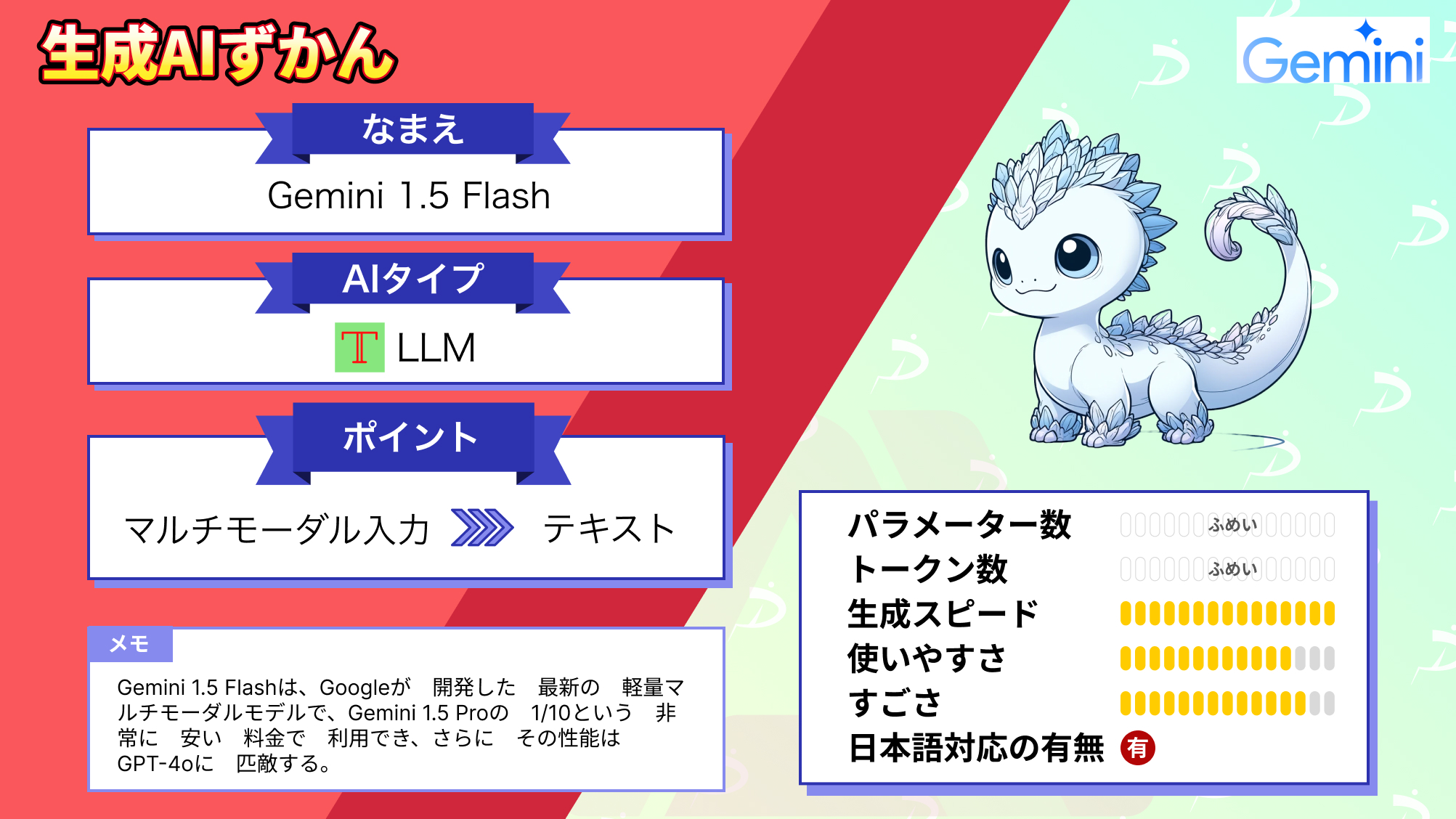
WEELメディア事業部LLMライターのゆうやです。
2024年5月15日のGoogleI/Oで、数多くのAIモデルや機能が公開され、Gemini 1.5 Flashもそのうちの一つです。
このモデルは、Geminiシリーズのなかで最も軽量で、高速な応答と高いコストパフォーマンスを実現しながら、コンテキストウィンドウは100万トークンを維持しているすごいモデルです。
Gemini 1.5 Proと同様にマルチモーダル推論が可能で、1時間のビデオや11時間のオーディオ入力に対応しています。
また、Gemini 1.5 Flashはその軽量さが売りですが、ベンチマークでGemini 1.0 Ultraと同等のスコアを獲得するほど十分な性能を有しています。
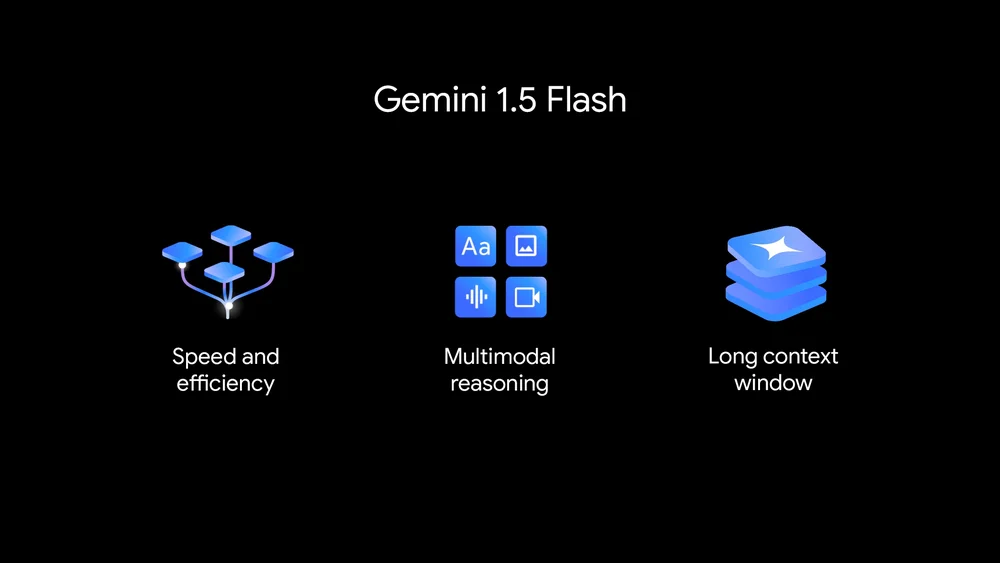
今回は、Gemini 1.5 Flashの概要や使ってみた感想をお伝えします。
是非最後までご覧ください!
\生成AIを活用して業務プロセスを自動化/
Gemini 1.5 Flashの概要
Gemini 1.5 Flashは、2024年5月15日に開催されたGoogle I/Oで発表された新しいAIモデルです。
このモデルは、Geminiシリーズのなかで最も軽量であり、応答の速さと非常に高いコスト効率を併せ持ちながら、100万トークンのコンテキストウィンドウを維持しています。
また、Gemini 1.5 Proと同様に動画や画像、音声などのマルチモーダル推論が可能で、1時間のビデオや11時間のオーディオ入力に対応しています。
ここで、Gemini 1.5 Flashの特徴をまとめます。
- スピードと効率性: Gemini 1.5 Flashは、低レイテンシーと高効率なタスク処理に特化しており、特にチャットアプリケーションやデータ抽出などの高頻度タスクに適しています。
- 大規模コンテキストウィンドウ: 最大100万トークンのコンテキストウィンドウを持ち、長文の要約、画像や動画のキャプション作成、長文ドキュメントやテーブルからのデータ抽出に優れています。
- マルチモーダル推論: テキスト、音声、画像、動画など、複数のモダリティを跨いだ推論が可能です。このため、多岐にわたる情報処理に対応できます。
- 軽量かつ強力: Proモデルのエッセンスを抽出し、軽量化されたにもかかわらず、Gemini 1.0 Ultraと同等の高度な性能を維持しています。
注目すべきはその性能で、軽量化しながらGemini 1.0 Ultraと同等の性能を維持することに成功しています。
以下の表は、Geminiシリーズのベンチマーク結果を比較したものです。
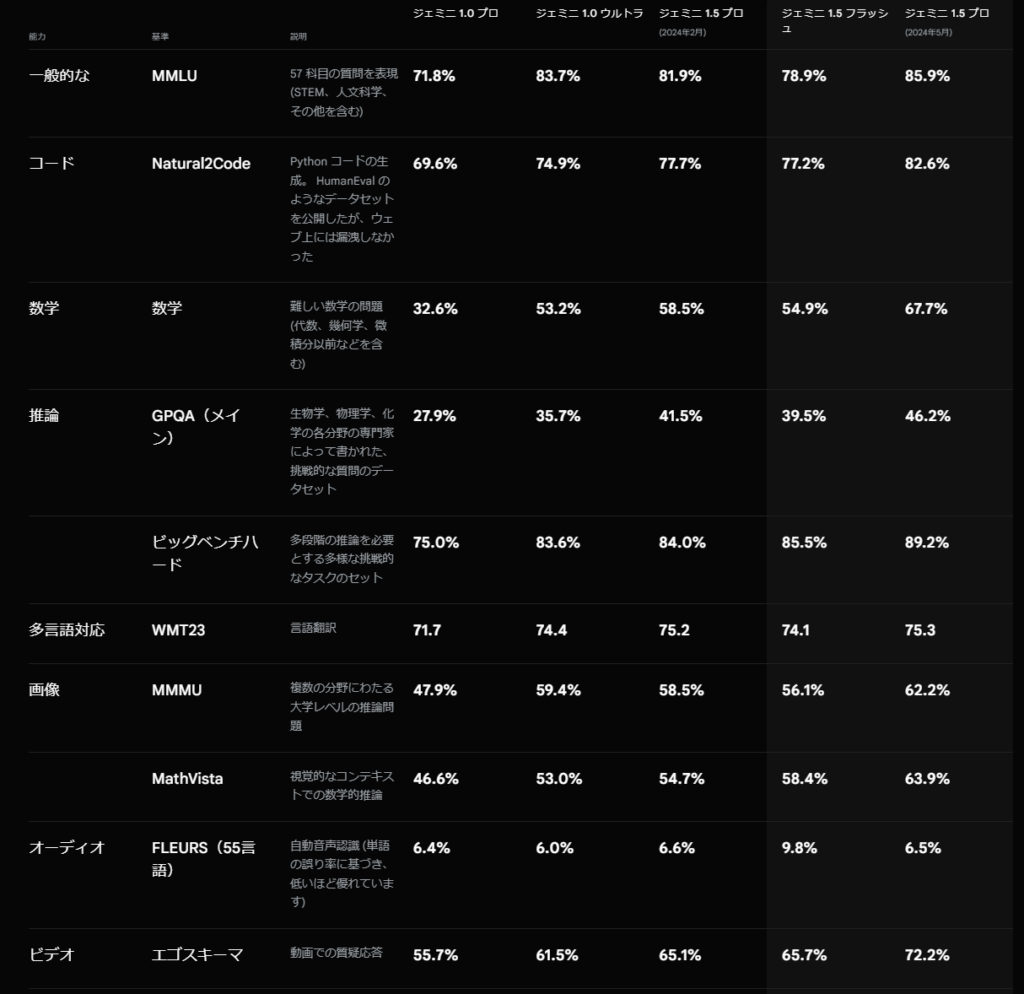
新しいGemini 1.5 Proのスコアの高さが目立ちますが、コストパフォーマンスで言えばGemini 1.5 Flashが圧倒的です。
続いて、気になるGemini 1.5 Flashの料金について紹介します。
Gemini 1.5 Flashの料金体系は、無料プランと従量課金制の2つに分かれています。
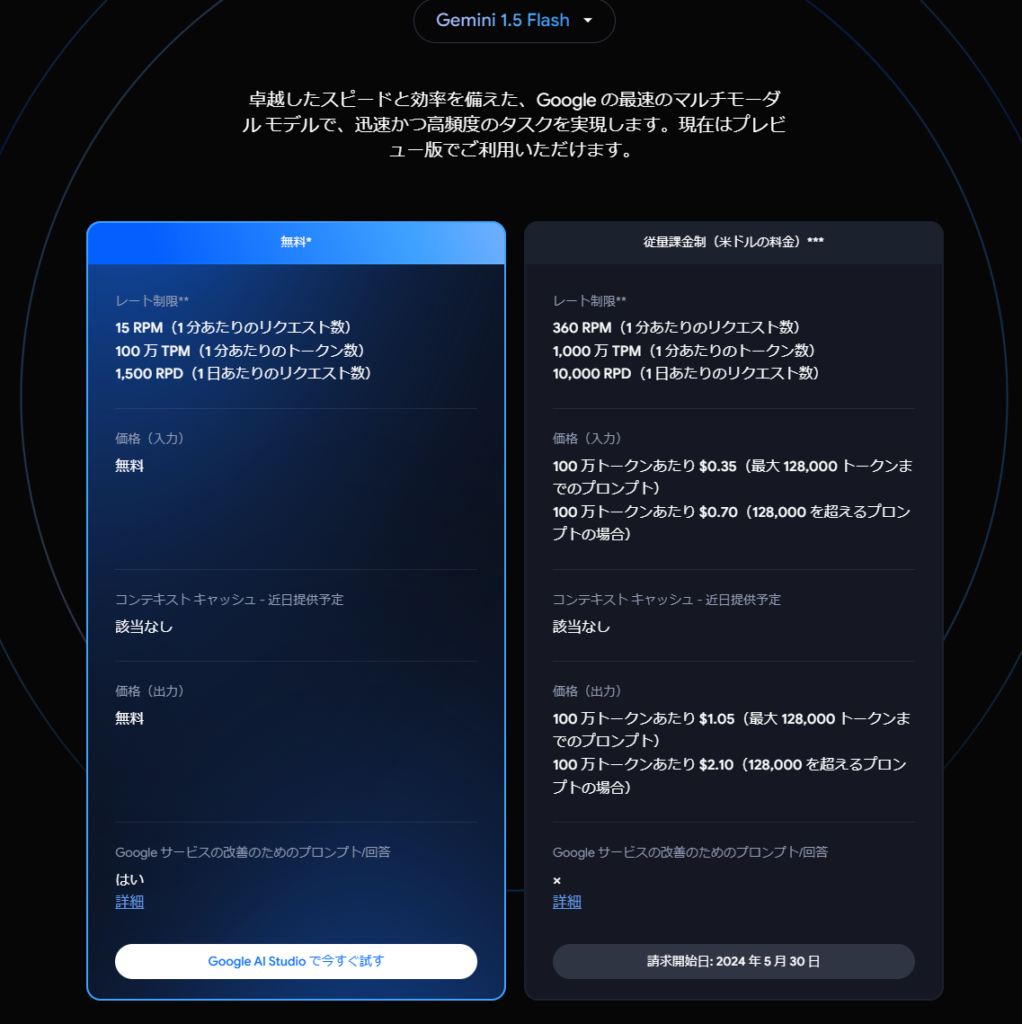
項目 | 無料プラン | 従量課金プラン(米ドルの料金) |
---|---|---|
レート制限 | – 15 RPM(1分あたりのリクエスト数) – 100万TPM(1分あたりのトークン数) – 1,500 RPD(1日あたりのリクエスト数) | – 360 RPM(1分あたりのリクエスト数) – 1,000万TPM(1分あたりのトークン数) – 10,000 RPD(1日あたりのリクエスト数) |
価格(入力) | 無料 | – 100万トークンあたり$0.35(最大128,000トークンまでのプロンプト) – 100万トークンあたり$0.70(128,000を超えるプロンプトの場合) |
コンテキストキャッシュ | 近日提供予定 | 近日提供予定 |
価格(出力) | 無料 | – 100万トークンあたり$1.05(最大128,000トークンまでのプロンプト) – 100万トークンあたり$2.10(128,000を超えるプロンプトの場合) |
無料プランもあり、本当に気軽に使えるモデルになっていますが、本格的に使う場合は従量課金プランを選択することをおすすめします。
Gemini 1.5 Flashのコスパの高さをもっと分かりやすくするために、Gemini 1.5 ProとOpen AIのGPTモデルとの料金比較表を以下に示します。
モデル | 入力料金 | 出力料金 |
---|---|---|
Gemini 1.5 Flash | $0.35 / 1Mトークン(128,000トークンまで) $0.70 / 1Mトークン(128,000を超える場合) | $1.05 / 1Mトークン(128,000トークンまで) $2.10 / 1Mトークン(128,000を超える場合) |
Gemini 1.5 Pro | $3.50 / 1Mトークン(128,000トークンまで) $7.00 / 1Mトークン(128,000を超える場合) | $10.50 / 1Mトークン(128,000トークンまで) $21.00 / 1Mトークン(128,000を超える場合) |
gpt-4o(-2024-05-13) | $5.00 / 1Mトークン | $15.00 / 1Mトークン |
gpt-4-turbo(-2024-04-09) | $10.00 / 1Mトークン | $30.00 / 1Mトークン |
gpt-3.5-turbo(-0125) | $0.50 / 1Mトークン | $1.50 / 1Mトークン |
Gemini 1.5 Flashは、Gemini 1.5 Proの1/10の料金で利用でき、Open AIのGPTモデルと比較するとさらに高いコスト効率を有していることが分かります。
ここからは、Gemini 1.5 Flashを実際に使用して、その性能を確かめていきます。
なお、Gemini 1.5 Proについて知りたい方はこちらの記事をご覧ください。
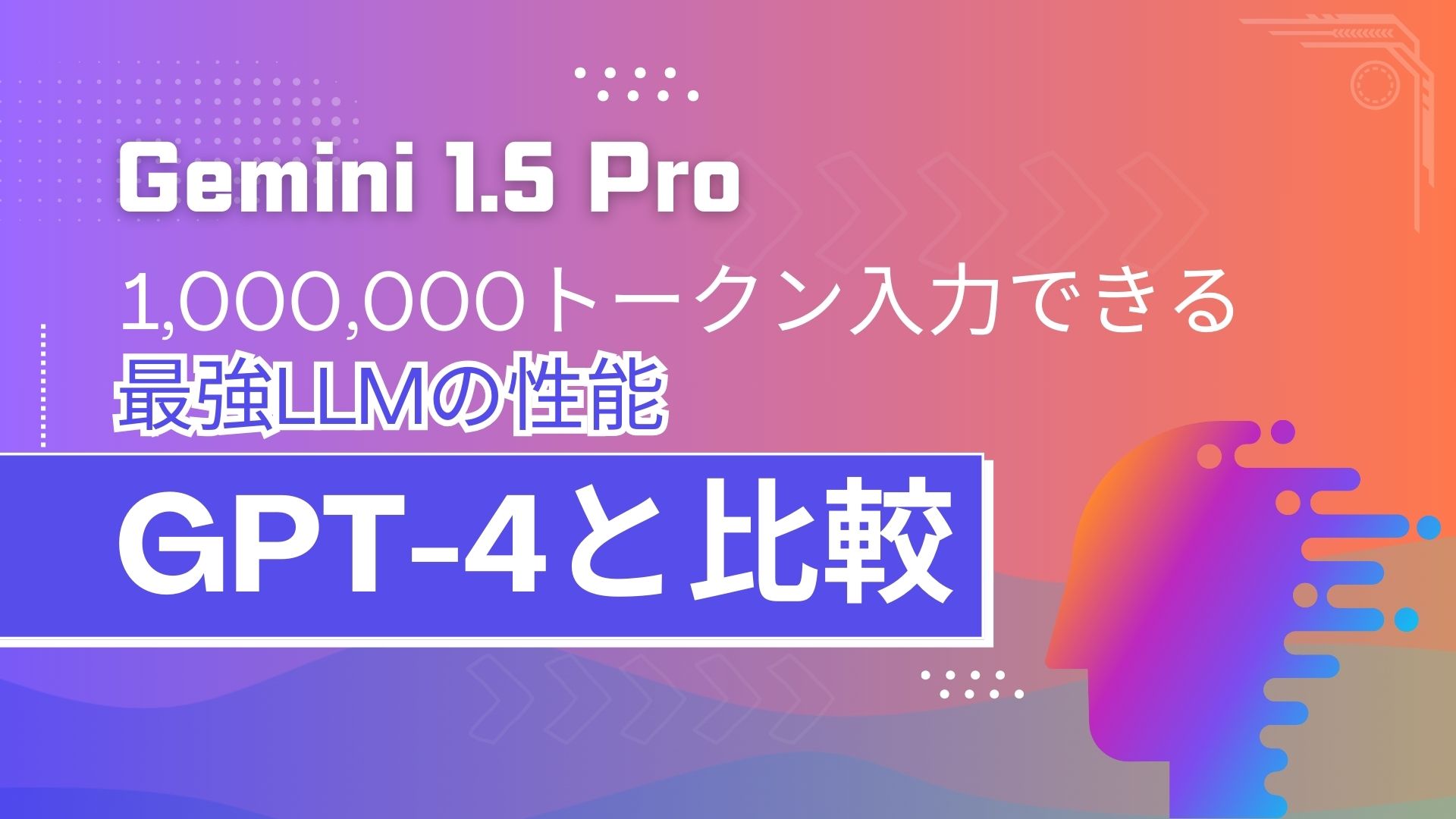
Gemini 1.5 Flashの使い方
Gemini 1.5 Flashは、現在Google AI StudioとVertex AIで利用可能になっています。
それぞれの使い方を説明していきます。
Google AI Studio
Google AI Studioを利用するには、以下のリンクにアクセスします。
ログインや利用規約の同意を済ませると、以下のような画面になるので、右上のボックスの部分でGemini 1.5 Flashを選択することで使用できます。
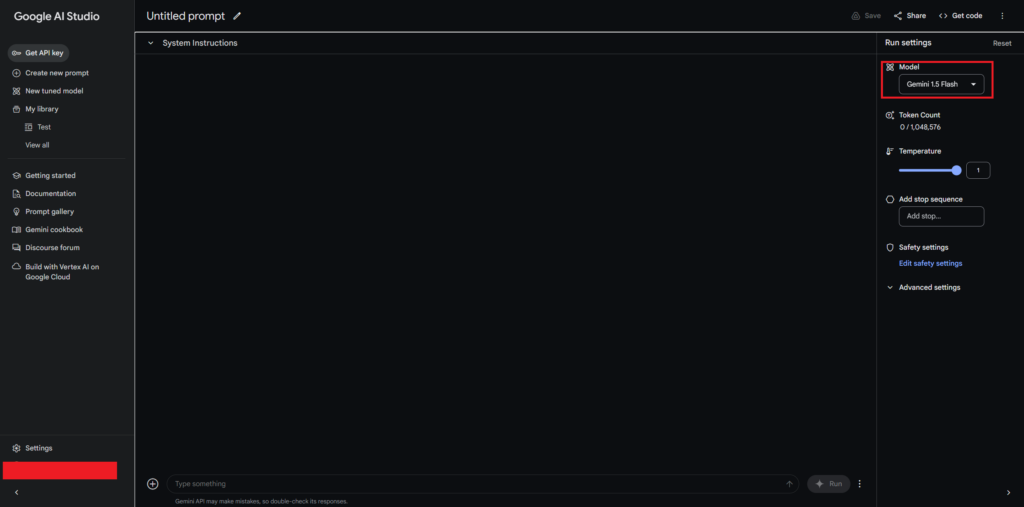
これで完了です。
Vertex AI
まず、Vertex AIのコンソールにアクセスします。
ログインなどの諸々の設定を済ませると以下のような画面になるので、Vertex AI Studioをクリックします。
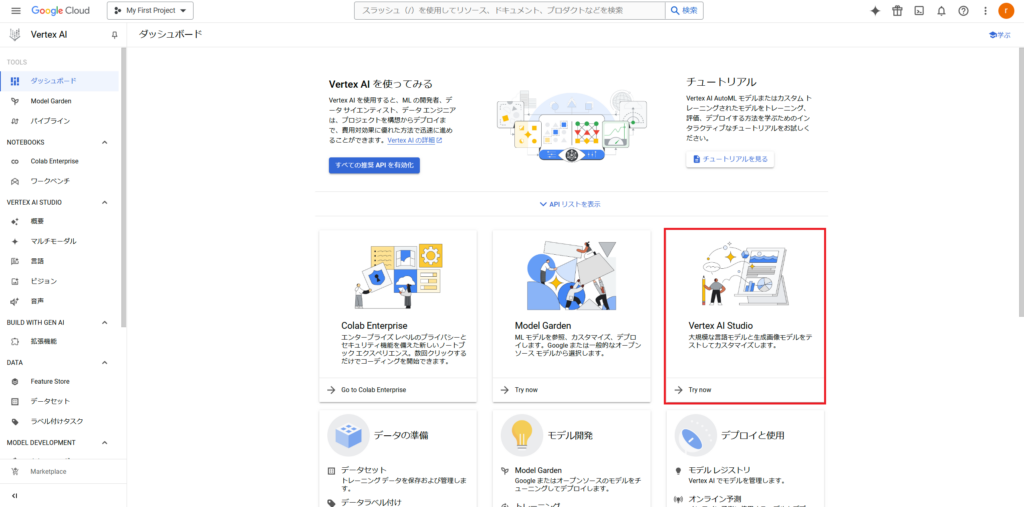
クリックすると、このような画面になるので、「GEMINIを試す」をクリックします。
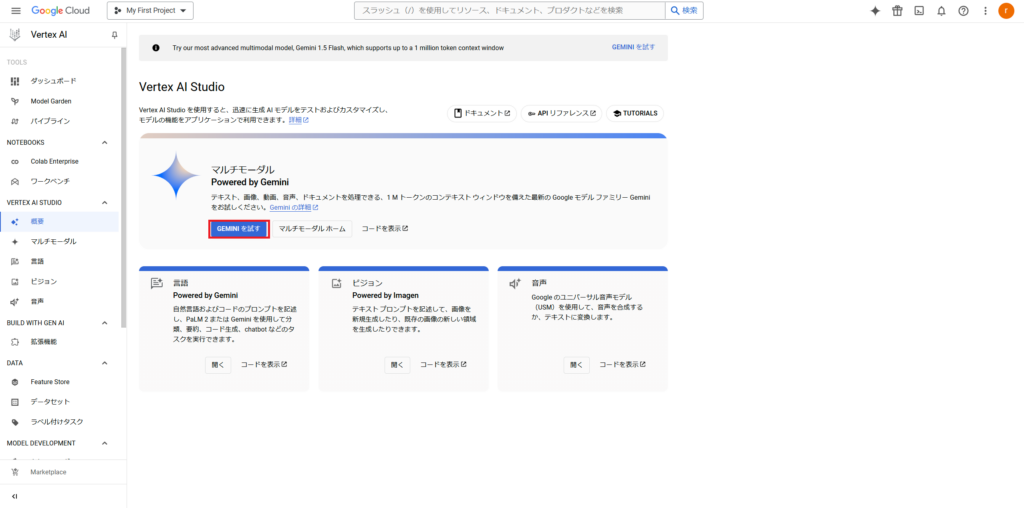
すると、このような画面になるので、右上のモデルからGemini 1.5 Flashを選択することで利用可能になります。
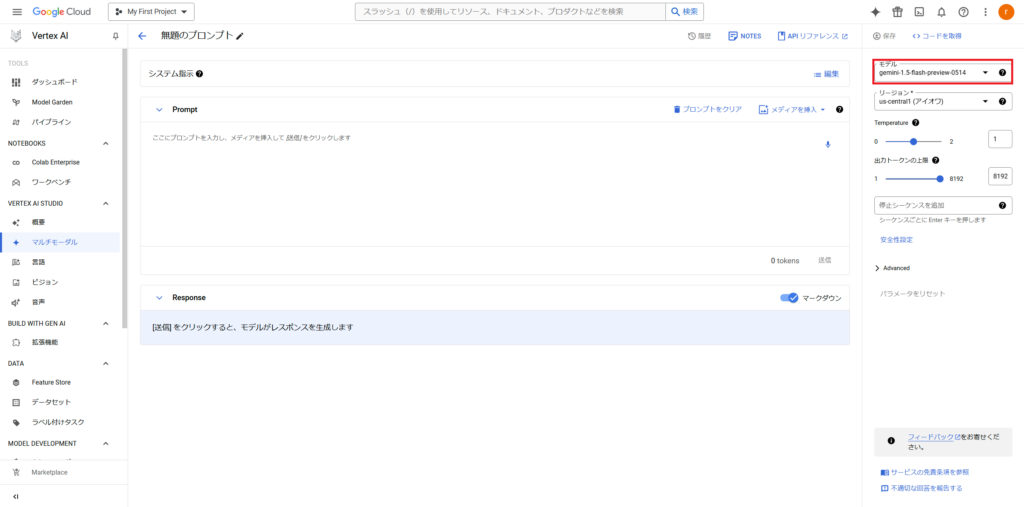
Vertex AIは、初回ログイン時に$300のフリークレジットがプレゼントされるので、初めてVertex AIを使う方はお得にGemini 1.5 Flashを利用できます。
さて、それでは実際にGemini 1.5 Flashを使用してみましょう!
Gemini 1.5 Flashを実際に使ってみた
以下のプロンプトを入力します。
Analyze the following information on the major combat and strategic records during World War II. In particular, provide a summary based on the key phases of the war, the strategies of the leaders, the outcome of the battles, and how they affected the flow and outcome of the war.
Key phases of the war and background
Key leaders and their strategies
The outcome of each battle and its impact
The overall flow and outcome of the war
「第二次世界大戦中の主要な戦闘および戦略に関する記録について、以下の内容を分析してください。特に、戦争の重要な局面、指導者の戦略、戦闘の結果に基づいた総括を行い、戦争の流れと結果にどのように影響を与えたかを説明してください。」
戦争の主要な局面と背景
主要な指導者とその戦略
各戦闘の結果とその影響
戦争全体の流れと結末
結果はこのようになりました。
結果はこちら
## Analyzing World War II: A Clash of Ideologies and Strategies
World War II was a global conflict unlike any other, spanning six years and engulfing nearly every corner of the world. Its legacy remains deeply etched in global politics, the international order, and the human psyche. This analysis explores the key phases, strategic approaches, and decisive battles that ultimately led to the Allied victory and reshaped the world.
**1. Key Phases of the War and Background:**
* **1939 - 1941: The Early Years and Axis Dominance:** The war began with Germany's invasion of Poland, prompting Britain and France to declare war. Germany, under the leadership of Adolf Hitler, employed the Blitzkrieg, a strategy of swift, overwhelming attacks that led to the rapid conquest of Poland, Denmark, Norway, the Netherlands, Belgium, and France.
* **1941 - 1943: The Turning Point:** 1941 marked a crucial shift. Germany's invasion of the Soviet Union, a strategic blunder, led to a protracted and bloody struggle on the Eastern Front. In the Pacific, Japan's attack on Pearl Harbor dragged the United States into the war. This period saw significant Allied victories, including the Battle of Midway, a decisive naval defeat for Japan, and the Battle of Stalingrad, a crushing blow to the German war machine.
* **1944 - 1945: The Allied Offensive and the Final Collapse:** The Allied forces, bolstered by the U.S. entry, launched major offensives on multiple fronts. The D-Day landings in Normandy in 1944, followed by the liberation of Western Europe, severely strained German resources. In the East, the Red Army relentlessly pushed westward, eventually capturing Berlin in 1945. The dropping of atomic bombs on Hiroshima and Nagasaki forced Japan's surrender in August 1945, bringing the war to an end.
**2. Key Leaders and Their Strategies:**
* **Adolf Hitler (Germany):** Hitler's strategy relied on aggressive expansionism, fueled by Nazi ideology. The Blitzkrieg was his primary tool, aiming for swift victories and demoralizing the enemy. His goals included conquering Europe and creating a "Greater German Reich." However, his underestimation of Soviet resistance and the strategic errors of Operation Barbarossa proved fatal.
* **Winston Churchill (Great Britain):** Churchill, the British Prime Minister, championed a defiant stance against Nazi Germany. He emphasized the importance of resisting Nazi aggression and rallied the British people through his inspiring speeches. He was a strong advocate for collaboration with the United States and was instrumental in securing American support.
* **Franklin D. Roosevelt (United States):** Initially pursuing a policy of neutrality, Roosevelt shifted the U.S. to an interventionist stance after Pearl Harbor. His strategy focused on defeating both Germany and Japan by deploying vast military and industrial resources, and by building strong alliances with Britain and the Soviet Union.
* **Joseph Stalin (Soviet Union):** Stalin's strategy was marked by unwavering determination and a willingness to endure immense casualties. The Soviet Union's strategy was primarily defensive, but they eventually mounted a powerful offensive that drove back the Germans. His post-war ambitions contributed to the Cold War.
**3. The Outcome of Each Battle and Its Impact:**
* **Battle of Britain (1940):** The Luftwaffe's failed attempt to gain air superiority over Britain marked a significant turning point, demonstrating the resilience of the British and the inadequacy of the German air force against a well-defended adversary.
* **Battle of Midway (1942):** The U.S. Navy's decisive victory over the Japanese fleet in the Pacific, crippled Japanese naval power and swung the tide of the war in the Pacific.
* **Battle of Stalingrad (1942-1943):** The grueling and devastating battle resulted in the complete defeat of the German Sixth Army. This defeat marked a turning point in the Eastern Front, highlighting the Soviet Union's resilience and the limitations of Germany's blitzkrieg tactics.
* **D-Day Landings (1944):** The successful Normandy landings opened a second front in Europe, putting immense pressure on the German war machine. It allowed the Allies to liberate France and eventually push into Germany.
* **Battle of Berlin (1945):** The Soviet capture of Berlin marked the end of Nazi Germany. It also marked the beginning of the Cold War as the Soviet Union solidified its control over Eastern Europe.
**4. The Overall Flow and Outcome of the War:**
World War II was a complex and brutal conflict driven by political and ideological differences. The early years saw a rapid expansion of Axis power, but the tide turned with the Soviet Union's relentless resistance and the United States' entry into the war. The Allied victory was achieved through a combination of military might, strategic planning, and the unwavering resolve of the Allied nations. The war's conclusion left a profound impact on the global landscape, leading to the rise of superpowers, the emergence of the Cold War, and a renewed emphasis on international cooperation.
**Conclusion:**
World War II was a watershed moment in human history, a conflict that reshaped the world order, redefined international relations, and left an indelible mark on human consciousness. By examining the key phases, the strategies of the leaders, the outcome of the battles, and the overall flow of the war, we can better understand the complex tapestry of events that led to its conclusion and appreciate the enduring consequences of this pivotal moment in history.
第二次世界大戦を分析する:イデオロギーと戦略の衝突
第二次世界大戦は、6年の歳月をかけ、世界のほぼ全域を巻き込んだ、他に類を見ない世界規模の紛争でした。その遺産は、世界政治、国際秩序、そして人間の精神に深く刻まれています。この分析では、最終的に連合軍を勝利に導き、世界を再形成した重要な局面、戦略的アプローチ、決定的な戦いを探ります。
- 戦争の主要な局面と背景:。
1939 – 1941: 戦争初期と枢軸国の支配: ドイツのポーランド侵攻で始まった戦争は、イギリスとフランスに宣戦布告を促しました。アドルフ・ヒトラー率いるドイツは、ポーランド、デンマーク、ノルウェー、オランダ、ベルギー、フランスの急速な征服につながった迅速かつ圧倒的な攻撃の戦略である電撃戦を採用しました。
1941 – 1943: ターニングポイント: 1941年、決定的な変化が起こりました。ドイツのソ連侵攻は戦略的な失策であり、東部戦線では長期にわたる血なまぐさい戦いが続きました。太平洋戦争では、日本の真珠湾攻撃が米国を戦争に引きずり込みました。この時期には、ミッドウェー海戦での日本の決定的な敗北や、スターリングラード海戦でのドイツ軍への大打撃など、連合軍の大きな勝利がありました。
1944 – 1945: 連合軍の攻勢と最終的な崩壊:連合軍は、米国の参戦によって強化され、複数の戦線で大規模な攻勢を開始しました。1944年のノルマンディー上陸作戦とそれに続く西ヨーロッパの解放は、ドイツの資源を著しく疲弊させました。東部では、赤軍が執拗に西へと押し進め、最終的に1945年にベルリンを占領。広島と長崎への原爆投下により、日本は1945年8月に降伏し、戦争は終結しました。
- 主な指導者とその戦略:。
アドルフ・ヒトラー(ドイツ):** ヒトラーの戦略は、ナチスのイデオロギーに煽られた攻撃的な拡張主義に依拠していました。電撃戦は彼の主要な手段であり、迅速な勝利と敵の戦意喪失を目指しました。彼の目標はヨーロッパの征服と “大ドイツ帝国 “の建設。しかし、ソ連の抵抗を過小評価し、バルバロッサ作戦の戦略ミスが致命傷となりました。
ウィンストン・チャーチル(イギリス):**イギリスの首相であったチャーチルは、ナチス・ドイツに対して反抗的な姿勢を唱えました。ナチスの侵略に抵抗することの重要性を強調し、鼓舞的な演説で英国民を結集。アメリカとの協調を強く主張し、アメリカの支持を取り付けることに尽力。
フランクリン・D・ルーズベルト(アメリカ合衆国): **当初は中立政策を追求していたルーズベルトは、真珠湾攻撃後、アメリカを介入主義的な姿勢に転換。ルーズベルトの戦略は、膨大な軍事・産業資源を投入し、イギリスやソ連と強固な同盟関係を築くことで、ドイツと日本の両方を打ち負かすことに重点を置いていました。
ヨシフ・スターリン(ソビエト連邦):** スターリンの戦略は、揺るぎない決意と甚大な犠牲を厭わない姿勢によって特徴付けられました。ソ連の戦略は主に防御的でしたが、最終的にはドイツ軍を追い返す強力な攻撃を展開しました。彼の戦後の野望は冷戦の一因となりました。
- 各戦闘の結果とその影響:。
** バトル・オブ・ブリテン(1940年):** ドイツ空軍がイギリス上空で制空権を得ようとして失敗したことは、イギリス軍の回復力とドイツ空軍の防備の固い敵に対する不十分さを示し、重要な転機となりました。
ミッドウェー海戦(1942年):**太平洋における日本艦隊に対するアメリカ海軍の決定的な勝利は、日本の海軍力を麻痺させ、太平洋における戦争の流れを変えました。
スターリングラードの戦い(1942-1943年):**過酷で壊滅的な戦いは、ドイツ第6軍の完全な敗北をもたらしました。この敗北は東部戦線の転換点となり、ソ連の回復力とドイツの電撃戦術の限界を浮き彫りにしました。
ノルマンディー上陸作戦(1944年):成功したノルマンディー上陸作戦は、ヨーロッパに第二戦線を開き、ドイツの戦争マシーンに絶大な圧力をかけました。その結果、連合軍はフランスを解放し、最終的にはドイツに攻め入ることができました。
ベルリンの戦い(1945年):ソ連によるベルリンの占領は、ナチス・ドイツの終焉を示しました。また、ソビエト連邦が東ヨーロッパに対する支配を強固にしたため、冷戦の始まりともなりました。
- 戦争の全体的な流れと結果:*。
第二次世界大戦は、政治的、イデオロギー的な相違によって引き起こされた複雑で残忍な紛争でした。初期には枢軸国の勢力が急速に拡大しましたが、ソ連の執拗な抵抗と米国の参戦によって流れが変わりました。連合国の勝利は、軍事力、戦略計画、そして連合国の揺るぎない決意の組み合わせによって達成されました。戦争終結は世界の情勢に大きな影響を残し、超大国の台頭、冷戦の勃興、国際協力の再重要化につながりました。
**結論
第二次世界大戦は人類史の分岐点であり、世界秩序を再構築し、国際関係を再定義し、人類の意識に消えない足跡を残した紛争でした。重要な局面、指導者たちの戦略、戦闘の結果、そして戦争の全体的な流れを検証することで、私たちは終結に至った複雑な出来事のタペストリーをよりよく理解し、この歴史上極めて重要な瞬間の永続的な結果を理解することができます。
与えられた項目に対して、非常に分かりやすい分析及び史実の解説をしてくれただけでなく、その後の世界に与えた影響の部分も回答してくれました。
さすがGemini 1.0 Ultraと同等の性能なだけありますね!
同じ質問を日本語でも入力してみました。
「第二次世界大戦中の主要な戦闘および戦略に関する記録について、以下の内容を分析してください。特に、戦争の重要な局面、指導者の戦略、戦闘の結果に基づいた総括を行い、戦争の流れと結果にどのように影響を与えたかを説明してください。」
戦争の主要な局面と背景
主要な指導者とその戦略
各戦闘の結果とその影響
戦争全体の流れと結末
結果は日本語でも問題なく、英語のプロンプトの際と同等の結果が返ってきました。
結果はこちら
第二次世界大戦: 戦争の流れと結末を決定した主要な局面と戦略
第二次世界大戦は、人類史上最も破壊的な戦争であり、世界中に多大な影響を与えました。この戦争は、数多くの重要な局面と戦略によって特徴付けられ、その結果、世界の勢力図は大きく変化しました。以下に、主要な局面と指導者の戦略、戦闘の結果、そして戦争全体の流れと結末に与えた影響について詳しく解説します。
1. 戦争の主要な局面と背景
1.1. ヨーロッパでの戦争勃発 (1939年): ナチスドイツによるポーランド侵攻が戦争の始まりを告げました。イギリスとフランスはドイツに宣戦布告し、第二次世界大戦が本格的に始まりました。
1.2. ドイツの電撃戦とヨーロッパ制圧 (1939-1941年): ドイツは、ポーランド、ノルウェー、デンマーク、オランダ、ベルギー、フランスを次々と制圧し、ヨーロッパ大陸の大部分を支配下に置きました。
1.3. 東部戦線とソ連の抵抗 (1941-1945年): ドイツは1941年6月、ソ連に侵攻しました。この東部戦線は、両陣営にとって最も過酷な戦場となり、数百万人の死者を出し、ソ連の抵抗はドイツの戦略を大きく阻害しました。
1.4. 太平洋戦争とアメリカの参戦 (1941年): 日本は1941年12月、真珠湾攻撃によってアメリカに宣戦布告し、太平洋戦争が勃発しました。アメリカの参戦は、戦争の様相を一変させ、連合国にとって決定的な転換点となりました。
1.5. 戦争の転換期 (1942-1943年): 1942年のミッドウェー海戦とスターリングラードの戦いでのドイツ軍の敗北は、戦争の潮目が変わり始めたことを示しました。連合国は徐々に戦局を有利に進めるようになりました。
1.6. 連合国の勝利と終戦 (1944-1945年): 1944年のノルマンディー上陸作戦と1945年のベルリン攻防戦は、連合国の勝利を決定づけました。1945年8月、日本が降伏し、第二次世界大戦は終結しました。
2. 主要な指導者とその戦略
2.1. アドルフ・ヒトラー (ドイツ): ナチス・ドイツの指導者であるヒトラーは、世界支配を目標に掲げ、電撃戦を用いてヨーロッパを制圧しようとした。彼の戦略は、初期には成功を収めたものの、ソ連との長期戦に突入するにつれて破綻し始めました。
2.2. ウィンストン・チャーチル (イギリス): イギリスの首相であるチャーチルは、ドイツに対する断固たる抵抗を主張し、連合国の勝利に大きく貢献しました。彼は、ナチス・ドイツと戦うためにアメリカとの同盟を重視し、アメリカの参戦を促しました。
2.3. フランクリン・ルーズベルト (アメリカ): アメリカの大統領であるルーズベルトは、当初は中立を保っていましたが、真珠湾攻撃を受けて日本に宣戦布告し、戦争に参戦しました。彼は、連合国を率いてドイツと日本を打ち負かし、世界平和を実現することに貢献しました。
2.4. ジョセフ・スターリン (ソ連): ソ連の最高指導者であるスターリンは、ドイツの侵攻に抵抗し、東部戦線で大きな犠牲を払ってドイツ軍を撃退しました。彼は、戦後の世界におけるソ連の影響力を拡大しようと試みました。
3. 各戦闘の結果とその影響
3.1. ノルマンディー上陸作戦 (1944年): 西部戦線における連合国の大規模な作戦であり、ドイツ軍をフランスから駆逐し、ヨーロッパ解放の道を切り開きました。
3.2. スターリングラードの戦い (1942-1943年): 東部戦線における決定的な戦闘であり、ドイツ軍の敗北は、東部戦線におけるドイツの優位性を失わせました。
3.3. ミッドウェー海戦 (1942年): 太平洋戦争における重要な海戦であり、アメリカ海軍が日本海軍に勝利し、太平洋における日本の優位性を失わせました。
3.4. 真珠湾攻撃 (1941年): 日本によるアメリカへの奇襲攻撃であり、アメリカの参戦を招き、戦争の様相を一変させました。
3.5. ベルリン攻防戦 (1945年): ソ連軍によるベルリン占領は、ナチス・ドイツの敗北を決定づけました。
4. 戦争全体の流れと結末
第二次世界大戦は、ドイツ、日本、イタリアを中心とする枢軸国とイギリス、アメリカ、ソ連を中心とする連合国の対立によって展開されました。戦争初期は、ドイツの電撃戦によって枢軸国が優勢でしたが、スターリングラードの戦い、ミッドウェー海戦、ノルマンディー上陸作戦などの重要な戦闘で連合国が勝利し、徐々に戦局を有利に進めました。最終的には、1945年に日本が降伏し、連合国の勝利によって戦争は終結しました。
5. 戦争の結果
第二次世界大戦は、世界中に壊滅的な被害をもたらしました。数千万人の民間人が犠牲となり、都市は破壊され、経済は崩壊しました。また、戦争の結果、世界の勢力図は大きく変化しました。アメリカとソ連は、世界を二分する超大国として台頭し、冷戦が始まりました。さらに、戦争は、ナチス・ドイツによるホロコーストなど、多くの悲劇をもたらし、人権や平和に対する意識を高める契機となりました。
結論
第二次世界大戦は、人類史上最も重要な出来事の一つであり、その影響は今日まで続いています。戦争の主要な局面と戦略、戦闘の結果、そして戦争全体の流れと結末を理解することで、現代社会における国際関係や平和構築について深く考えることができます。
ここからは、Gemini 1.5 Flashの性能やマルチモーダル推論能力を、GPT-4oと比較していきます。
Gemini 1.5 Flashの性能やマルチモーダル推論能力を、GPT-4oと比較してみた
ここでは、Gemini 1.5 Flashの性能を測るため、最新のマルチモーダルモデルであるGPT-4oとの比較を行っていきます。
以下の項目を比較します。
- 論文の分析(Gemmaのテクニカルレポート)
- 17分の動画の文字起こし(Google I/O 2024のGoogle DeepMindパート)
- コーディング能力(形態素解析)
早速比較検証していきましょう!
論文の分析(Gemmaのテクニカルレポート)
まずは、Gemmaのテクニカルレポートを入力して、その内容の分析を行います。
以下のプロンプトを入力します。
This is the Gemma technical report. Please analyze the report and provide a detailed description of Gemma.
これはGemmaのテクニカルレポートです。レポートを分析して、Gemmaについて詳細な説明をしてください。
結果はこのようになりました。
Gemini 1.5 Flashの結果はこちら
## Gemma: Open Models Based on Gemini Research and Technology
This technical report introduces Gemma, a family of lightweight, open-source language models (LLMs) built using research and technology from Google's Gemini models. Gemma aims to bridge the gap between powerful, closed-source LLMs and accessible, open-source models, enabling wider research and development.
Here's a detailed description of Gemma:
**Key Features:**
* **Lightweight and Efficient:** Gemma comes in two sizes: 2 billion parameters for CPU/on-device applications and 7 billion parameters for efficient deployment on GPUs/TPUs.
* **Open Source:** Both pretrained and fine-tuned checkpoints are released alongside an open-source codebase for inference and serving.
* **Strong Performance:** Gemma outperforms similarly sized open models on various academic benchmarks in language understanding, reasoning, and safety, including question answering, commonsense reasoning, math/science, and coding.
* **Gemini-Inspired:** The architecture, training data, and techniques used to train Gemma are inspired by Google's Gemini models, demonstrating strong generalist capabilities and state-of-the-art performance at scale.
**Model Architecture:**
* Based on the Transformer decoder architecture.
* Utilizes several improvements:
* Multi-Query Attention (for 2B model) and Multi-Head Attention (for 7B model).
* RoPE Embeddings (rotary positional embeddings) for efficient positional information encoding.
* GeGLU Activations for enhanced non-linearity.
* RMSNorm for training stability.
**Training Infrastructure:**
* Trained on TPUv5e, leveraging a single controller paradigm for simplified development.
* Utilizes GSPMD partitioner for training step computation and MegaScale XLA compiler.
* Estimated carbon emissions for training are ∼ 131 𝑡𝐶𝑂2𝑒𝑞, with Google data centers operating carbon neutrally.
**Pretraining:**
* Trained on 3T and 6T tokens of primarily English data from web documents, mathematics, and code.
* Employs a subset of the SentencePiece tokenizer used in Gemini.
* Dataset filtered to minimize harmful content and sensitive data using both heuristics and model-based classifiers.
**Instruction Tuning:**
* Fine-tuned with supervised fine-tuning (SFT) on a mix of synthetic and human-generated prompt-response pairs.
* Further fine-tuned with Reinforcement Learning from Human Feedback (RLHF) for improved instruction following, helpfulness, and safety.
* A specific formatter is used to annotate instruction-tuning examples for better model performance during inference.
**Evaluation:**
* Thoroughly evaluated across a wide range of quantitative and qualitative benchmarks, including both standard academic benchmarks and human preference evaluations.
* Gemma outperforms comparable open models on 11 out of 18 text-based tasks.
* Demonstrates strong performance on mathematics and coding benchmarks.
* Evaluated for memorization and personal data retention using established methodologies.
**Responsible Deployment:**
* Adheres to a structured approach to responsible development and deployment.
* Implements various safety measures including:
* Dataset filtering for harmful and sensitive content.
* Standardized safety benchmark testing.
* Internal red teaming to assess risks associated with external use.
* Rigorous ethics and safety evaluations.
**Benefits:**
* Encourages research and development in the AI community by providing access to state-of-the-art, open-source models.
* Facilitates innovation in areas such as science, education, and the arts.
* Reduces economic and technical barriers for developers to incorporate these technologies into their workstreams.
**Risks:**
* Potential misuse by bad actors for harmful purposes.
* Challenges in mitigating unintended model behaviors like bias, hallucinations, and personal data leakage.
**Mitigations:**
* Extensive filtering and measurement of bias in pre-training data.
* Comprehensive safety assessments using standardized benchmarks and internal red teaming.
* Rigorous ethics and safety evaluations.
* Publication of a detailed model card and Generative AI Responsible Toolkit to aid developers in building AI responsibly.
**Assessment:**
* OpenAI believes the benefits of Gemma to the AI community outweigh the risks.
**Going Forward:**
* Google DeepMind remains committed to responsible open model development, with plans to explore extended testing, staggered releases, and alternative access mechanisms to ensure safety.
* Encourages the wider AI community to adopt a nuanced, collaborative approach to AI risks and benefits, focusing on research in areas like AI literacy, economic and social resilience, and anticipatory governance.
**Overall, Gemma represents a significant step towards greater accessibility and transparency in the field of LLMs. Its strong performance, open-source nature, and focus on responsible deployment make it a valuable resource for advancing research, innovation, and safe development in the field of AI.**
Gemma: Gemini の研究とテクノロジーに基づくオープン モデル
この技術レポートでは、Google の Gemini モデルの研究とテクノロジーを使用して構築された軽量のオープンソース言語モデル (LLM) ファミリーである Gemma を紹介します。 Gemma は、強力なクローズドソース LLM とアクセス可能なオープンソース モデルの間のギャップを埋め、より広範な研究開発を可能にすることを目指しています。
Gemma の詳細な説明は次のとおりです。
主な機能:
- 軽量かつ効率的: Gemma には 2 つのサイズがあります。CPU/オンデバイス アプリケーション用の 20 億パラメータと、GPU/TPU での効率的な展開用の 70 億パラメータです。
- オープンソース: 事前トレーニングされたチェックポイントと微調整されたチェックポイントの両方が、推論と提供のためにオープンソースのコードベースとともにリリースされます。
- 優れたパフォーマンス: Gemma は、質問応答、常識的推論、数学/科学、コーディングなどの言語理解、推論、安全性におけるさまざまな学術ベンチマークにおいて、同様のサイズのオープン モデルよりも優れたパフォーマンスを発揮します。
- Gemini からインスピレーションを得た: Gemma のトレーニングに使用されるアーキテクチャ、トレーニング データ、テクニックは Google の Gemini モデルからインスピレーションを受けており、強力なジェネラリスト機能と最先端のパフォーマンスを大規模に実証しています。
モデル アーキテクチャ:
- Transformer デコーダ アーキテクチャに基づいています。
- いくつかの改善を利用します:
- マルチクエリ アテンション (2B モデルの場合) およびマルチヘッド アテンション (7B モデルの場合)。
- 効率的な位置情報エンコーディングのための RoPE エンベディング (回転位置エンベディング)。
- GeGLU アクティベーションによる非線形性の強化。
- トレーニングの安定性のための RMSNorm。
トレーニング インフラストラクチャ:
- TPUv5e でトレーニングされ、単一コントローラー パラダイムを活用して開発を簡素化します。
- トレーニング ステップ計算および MegaScale XLA コンパイラに GSPMD パーティショナーを利用します。
- Google データセンターはカーボン ニュートラルで運用されており、トレーニングによる推定二酸化炭素排出量は約 131 𝑡𝐶𝑂2𝑒𝑞です。
事前トレーニング:
- Web ドキュメント、数学、コードからの主に英語データの 3T および 6T トークンでトレーニングされました。
- Gemini で使用される SentencePiece トークナイザーのサブセットを採用します。
- ヒューリスティックとモデルベースの分類器の両方を使用して、有害なコンテンツと機密データを最小限に抑えるためにデータセットがフィルタリングされます。
命令チューニング:
- 合成および人間が生成したプロンプト応答ペアを組み合わせた教師付き微調整 (SFT) で微調整されます。
- ヒューマン フィードバックからの強化学習 (RLHF) でさらに微調整され、指示への追従性、有用性、安全性が向上しました。
- 推論中のモデルのパフォーマンスを向上させるために、特定のフォーマッタを使用して命令チューニングの例に注釈を付けます。
評価:
- 標準的な学術ベンチマークと人間の好みの評価の両方を含む、幅広い定量的および定性的ベンチマークにわたって徹底的に評価されます。
- Gemma は、18 個のテキストベースのタスクのうち 11 個で、同等のオープン モデルよりも優れたパフォーマンスを発揮します。
- 数学およびコーディングのベンチマークで優れたパフォーマンスを示します。
- 確立された方法論を使用して、記憶と個人データの保持について評価されます。
責任ある展開:
- 責任ある開発と導入に対する構造化されたアプローチを遵守します。 ※以下のようなさまざまな安全対策を実施しています。
- 有害で機密性の高いコンテンツに対するデータセットのフィルタリング。
- 標準化された安全ベンチマークテスト。
- 外部使用に関連するリスクを評価するための内部レッドチーム。
*厳格な倫理と安全性の評価。
利点:
- 最先端のオープンソース モデルへのアクセスを提供することで、AI コミュニティでの研究開発を促進します。
- 科学、教育、芸術などの分野におけるイノベーションを促進します。
- 開発者がこれらのテクノロジーをワークストリームに組み込む際の経済的および技術的障壁を軽減します。
リスク:
- 悪意のある者による有害な目的での悪用の可能性。
- 偏見、幻覚、個人データの漏洩など、モデルの意図しない動作を軽減するという課題。
緩和策:
- 事前トレーニングデータの広範なフィルタリングとバイアスの測定。
- 標準化されたベンチマークと内部のレッドチームを使用した包括的な安全性評価。
*厳格な倫理と安全性の評価。 - 開発者が責任を持って AI を構築するのを支援する、詳細なモデル カードと Generative AI Responsible Toolkit の公開。
評価:
- OpenAI は、AI コミュニティに対する Gemma の利点がリスクを上回ると信じています。
今後:
- Google DeepMind は引き続き責任あるオープン モデルの開発に取り組んでおり、安全性を確保するためのテストの延長、段階的なリリース、代替アクセス メカニズムを検討する予定です。
- AI リテラシー、経済的および社会的回復力、予期的なガバナンスなどの分野の研究に焦点を当て、AI のリスクと利点に対して微妙な協力的アプローチを採用することを広範な AI コミュニティに奨励します。
全体として、Gemma は LLM 分野におけるアクセシビリティと透明性の向上に向けた重要な一歩を表しています。 その強力なパフォーマンス、オープンソースの性質、責任ある導入への重点により、AI 分野での研究、イノベーション、安全な開発を推進するための貴重なリソースとなっています。
GPT-4oの結果はこちら
The technical report on Gemma provides a comprehensive overview of this advanced language model developed by Google DeepMind. Here's a detailed description of Gemma:
### Overview
- **Gemma** is a series of state-of-the-art, lightweight, open models derived from the technology and research used in the Gemini models.
- The models are designed to be open and are available in two sizes: 2 billion and 7 billion parameters, catering to different computational needs and environments (GPU, TPU, CPU, and on-device applications).
- Gemma has demonstrated superior performance across a range of academic benchmarks, especially in language understanding, reasoning, and safety.
### Model Architecture and Training
- Gemma models are based on the transformer architecture, specifically the transformer decoder, and include several innovative technologies such as Multi-Query Attention, RoPE Embeddings, and GeGLU activations.
- Training was conducted on up to 6 trillion tokens from text-based datasets, using a pretraining and fine-tuning approach.
- The models are equipped with safety and helpfulness tuning, designed to enhance performance while minimizing potential harms.
### Performance
- Gemma models outperform similar open models in 11 out of 18 text-based tasks.
- The models are tested thoroughly against quantitative and qualitative benchmarks, demonstrating robust capabilities in reasoning, math, science, and coding.
### Safety and Ethical Considerations
- The development and release of Gemma models consider the importance of safety and ethics in AI, with detailed assessments on their responsible use.
- Precautions are taken to minimize risks associated with deployment, including extensive safety evaluations and the application of filters to reduce the risk of harmful outputs.
### Deployment and Applications
- Gemma models are available in pre-trained and fine-tuned formats, along with an open-source codebase for inference and serving.
- They are intended for a wide range of applications, from academic research to real-world problem solving in various domains.
### Limitations and Further Work
- Despite their advanced capabilities, Gemma models share common limitations of AI models, such as potential for biased outputs and the challenge of fully understanding complex human contexts.
- The report emphasizes ongoing efforts to address these limitations, with continued research and updates expected to enhance model performance and safety.
### Conclusion
- Gemma represents a significant advancement in open model availability, combining high performance with a strong focus on safety and ethical AI development.
- Its release supports the broader AI community by providing tools that lower barriers to innovation and facilitate more secure and robust AI applications.
This report highlights Google DeepMind's commitment to advancing AI technology while addressing the critical need for responsible AI development and deployment.
Gemma の技術レポートでは、Google DeepMind によって開発されたこの高度な言語モデルの包括的な概要が提供されます。 Gemma の詳細な説明は次のとおりです。
### 概要
- Gemma は、Gemini モデルで使用されたテクノロジーと研究から派生した、最先端の軽量オープン モデルのシリーズです。
- モデルはオープンになるように設計されており、20 億パラメーターと 70 億パラメーターの 2 つのサイズで利用でき、さまざまな計算ニーズと環境 (GPU、TPU、CPU、オンデバイス アプリケーション) に対応します。
- Gemma は、特に言語理解、推論、安全性において、さまざまな学術ベンチマークにわたって優れたパフォーマンスを示しています。
###モデルのアーキテクチャとトレーニング
- Gemma モデルは、トランス アーキテクチャ、特にトランス デコーダに基づいており、マルチクエリ アテンション、RoPE エンベディング、GeGLU アクティベーションなどのいくつかの革新的なテクノロジが含まれています。
- 事前トレーニングと微調整アプローチを使用して、テキストベースのデータセットから最大 6 兆のトークンに対してトレーニングが実施されました。
- モデルには安全性と有用性のチューニングが装備されており、潜在的な危害を最小限に抑えながらパフォーマンスを向上させるように設計されています。
### パフォーマンス
- Gemma モデルは、18 個のテキストベースのタスクのうち 11 個で、同様のオープン モデルよりも優れたパフォーマンスを発揮します。
- モデルは定量的および定性的なベンチマークに対して徹底的にテストされ、推論、数学、科学、コーディングにおける堅牢な機能を実証しています。
###安全性と倫理的配慮
- Gemma モデルの開発とリリースでは、責任ある使用に関する詳細な評価とともに、AI における安全性と倫理の重要性が考慮されています。
- 広範な安全性評価や有害な出力のリスクを軽減するためのフィルターの適用など、導入に関連するリスクを最小限に抑えるための予防措置が講じられています。
###導入とアプリケーション
- Gemma モデルは、推論と提供のためのオープンソース コードベースとともに、事前トレーニングおよび微調整された形式で利用できます。
- 学術研究からさまざまな分野における現実世界の問題解決まで、幅広い用途を対象としています。
###制限とさらなる作業
- Gemma モデルは高度な機能にもかかわらず、偏った出力の可能性や複雑な人間のコンテキストを完全に理解するという課題など、AI モデルに共通する制限を共有しています。
- このレポートでは、モデルのパフォーマンスと安全性を向上させるために継続的な研究と更新が期待されており、これらの制限に対処するための継続的な取り組みが強調されています。
### 結論
- Gemma は、安全性と倫理的な AI 開発に重点を置きながら、高いパフォーマンスを組み合わせた、オープン モデルの可用性における大幅な進歩を表しています。
- そのリリースは、イノベーションへの障壁を低くし、より安全で堅牢な AI アプリケーションを促進するツールを提供することで、より広範な AI コミュニティをサポートします。
このレポートは、責任ある AI の開発と導入という重要なニーズに対処しながら、AI テクノロジーを進化させる Google DeepMind の取り組みを強調しています。
結果は、Gemini 1.5 FlashのほうがGPT-4oより詳細な回答を生成してくれました。
Gemini 1.5 Flashは、技術的な内容だけでなく、利点やリスク、緩和策を分かりやすくまとめています。
GPT-4oの回答も要点がまとめられており、大変わかりやすいものだと感じますが、個人的にはGemini 1.5 Flashの回答のほうが優れていると感じました。
17分の動画の文字起こし(Google I/O 2024のGoogle DeepMindパート)
続いて、Google I/O 2024のGoogle DeepMindパートの約17分の動画を入力して、文字起こしをしてもらいます。
結果はこのようになりました。
Gemini 1.5 Flashの結果はこちら
Thanks Sundar. It’s so great to be here. Ever since I was a kid playing chess for the England Junior team I’ve been thinking about the nature of intelligence. I was captivated by the idea of a computer that could think like a person. It’s ultimately why I became a programmer and studied neuroscience. I co-founded DeepMind in 2010 with the goal of one day building AGI. Artificial General Intelligence. A system that has human level cognitive capabilities. I’ve always believed that if we could build this technology responsibly it’s impact would be truly profound and it could benefit humanity in incredible ways. Last year we reached a milestone on that path when we formed Google DeepMind combining AI talent from across the company into one super unit. Since then we’ve built AI systems that can do an amazing range of things from turning language and vision into action for robots, navigating complex virtual 3D environments, solving Olympiad level math problems, and even discovering thousands of new materials. Just last week, we announced our next generation AlphaFold model. It can predict the structure and interactions of nearly all of life’s molecules including how proteins interact with strands of DNA and RNA. This will accelerate vitally important biological and medical research from disease understanding to drug discovery. And all of this was made possible with the best infrastructure for the AI era, including our highly optimized tensor processing units. At the center of our efforts is our Gemini model. It’s built up from the ground up to be natively multimodal because that’s how we interact with and understand the world around us. We’ve built a variety of models for different use cases. You’ve seen how powerful Gemini 1.5 Pro is but we also know from user feedback that some applications need lower latency and a lower cost to serve. So, today we’re introducing Gemini 1.5 Flash. Flash! Flash is a lightweight model compared to Pro. It’s designed to be fast and cost efficient to serve at scale, while still featuring multimodal reasoning capabilities, and breakthrough long context. Flash is optimized for tasks where low latency and efficiency matter most. Starting today, you can use 1.5 Flash and 1.5 Pro with up to 1 million tokens in Google AI Studio and Vertex AI and developers can sign up to try to million tokens. We’re so excited to see what all of you will create with it and you’ll hear a little more about Flash later on from Josh. We’re very excited by the progress we made so far with our family of Gemini models, but we’re always striving to push the state of the art even further. At any one time we have many different models in training and we use our very large and powerful ones to help teach and train our production-ready models. Together with user feedback, this cutting edge research will help us to build amazing new products for billions of people. For example, in December, we shared a glimpse into the future of how people would interact with multimodal AI. And this would How this would end up powering a new set of transformative experiences. Today we have some exciting new progress to share about the future of AI assistants that we’re calling Project Astra. For a long time, we’ve wanted to build a universal AI agent that can be truly helpful in everyday life. Our work making this vision a reality goes back many years. It’s why we made Gemini multimodal from the very beginning. An agent like this has to understand and respond to our complex and dynamic world just like we do. It would need to take in and remember what it sees so we can understand context and take action and it would have to be proactive, teachable and personal. So, you can talk to it naturally without lag or delay. While we’ve made some great strides in developing AI systems that can understand multimodal information getting response time down to something conversational is a difficult engineering challenge. Building on our Gemini model we’ve developed agents that can process information faster, by continuously encoding video frames combining the video and speech input into a timeline of events, and caching this for efficient recall. We’ve also enhanced how they sound with a wider range of intonations. These agents better understand the context you’re in, and can respond quickly in conversation making the pace and quality of interaction feel much more natural. Here’s a video of our prototype, which we’ll see has two parts. Each part was captured in a single take in real time. Okay. Let’s do some tests. Tell me when you see something that makes sound. I see a speaker, which makes sound. What is that part of the speaker called? That is the tweeter. It produces high-frequency sounds. Give me a creative alliteration about these. Creative crayons color cheerfully. They certainly craft colorful creations. What does that part of the code do? This code defines encryption and decryption functions. It seems to use AES-CBC encryption to encode and decode data based on a key and an initialization vector IV. That’s right. What neighborhood do you think I’m in? This appears to be the King’s Cross area of London. It is known for its railway station and transportation connections. Do you remember where you saw my glasses? Yes, I do. Your glasses were on the desk near a red apple. That’s right, this is the King’s Cross area of London. It is known for its railway station and transportation connections. Do you remember where you saw my glasses? Yes, I do. Your glasses were on the desk near a red apple.
GPT-4oの結果はこちら
It seems that I am unable to run the `whisper` transcription tool directly in this environment. As an alternative, I can process the audio from the video file using available tools in the current environment. Here’s how we can proceed:
1. Extract the audio from the video file.
2. Use an audio transcription tool available in this environment to transcribe the audio.
Let's start by extracting the audio from the video file.
The audio has been successfully extracted from the video. The next step is to transcribe the audio file. I'll use an audio transcription tool available in this environment to transcribe the extracted audio.
It appears that the `speech_recognition` library is not available in this environment. As an alternative, I can use another method to transcribe the audio. Let's try using a different tool that is available here.
I'll use the `pydub` library to handle audio processing and check if we have any other transcription tools available. Let me first confirm the libraries and tools available in this environment.
It seems there was an error in checking the installed packages. Let me try a different approach to list the available libraries.
The environment has several installed libraries, including `pydub` and `gtts` (Google Text-to-Speech). However, a direct transcription library like `speech_recognition` is not available.
I will proceed with an alternative approach by converting the audio to text using `gtts`. This method isn't ideal for transcription but can serve to confirm the audio processing capabilities.
Since direct transcription isn't feasible here, you might consider uploading the audio to a service that supports transcription, such as Google Cloud Speech-to-Text, or using local tools if available on your machine.
If you want me to generate a rough text representation using `gtts` for the extracted audio, please confirm, and I'll proceed with that approach. Alternatively, you can download the extracted audio and use external transcription tools.
この環境では whisper
テープ起こしツールを直接実行することができないようです。別の方法として、現在の環境で利用可能なツールを使って、ビデオファイルの音声を処理することができます。以下はその方法です:
- ビデオファイルから音声を抽出します。
- この環境で利用可能な音声書き起こしツールを使って、音声を書き起こします。
まず、動画ファイルから音声を抽出します。
動画から音声が抽出されました。次は音声ファイルを書き起こします。この環境で利用できる音声書き起こしツールを使って、取り出した音声を書き起こしてみます。
この環境では speech_recognition
ライブラリが利用できないようです。代替案として、別の方法で音声を書き起こすことができます。ここで利用できる別のツールを使ってみましょう。
ここでは pydub
ライブラリを使って音声処理を行い、他に利用可能な書き起こしツールがないか確認してみます。まず、この環境で利用できるライブラリとツールを確認してみます。
インストールされているパッケージのチェックにエラーがあったようです。別のアプローチで利用可能なライブラリをリストアップしてみます。
この環境には pydub
や gtts
(Google Text-to-Speech) などのライブラリがインストールされています。しかし、speech_recognition
のような直接書き起こしライブラリはありません。
別の方法として、gts
を使って音声をテキストに変換することにします。この方法は文字起こしには理想的ではありませんが、音声処理能力を確認するのに役立ちます。
ここでは直接テープ起こしはできないので、Google Cloud Speech-to-Textのようなテープ起こしをサポートするサービスに音声をアップロードするか、あなたのマシンで利用可能であればローカルツールを使うことを検討してください。
抽出した音声に対して gtts
を使って大まかなテキスト表現を生成してほしい場合は、確認してください。また、抽出した音声をダウンロードし、外部の書き起こしツールを使用することもできます。
Gemini 1.5 Flashは、途中で出力が止まり、冒頭から約7分間のみ文字起こしをしてくれました。
ただ、出力された文字起こしは正確で、ほとんど間違いのないものだったので、その性能の高さが伺えます。
一方のGPT-4oは、様々な手段で文字起こしを使用としてくれていますが、どれも失敗し、最終的には他のツールで文字起こしをするように促しています。
この比較でも、Gemini 1.5 Flashのほうが優秀な結果になりました。
コーディング能力(形態素解析)
最後に、コーディング能力を検証するため、英語のテキストを日本語に翻訳して形態素解析まで行うプログラムを生成してもらいます。
以下のプロンプトを入力します。
Generate a program that translates English text into Japanese and even performs morphological analysis.
英語のテキストを日本語に翻訳して形態素解析まで行うプログラムを生成して
結果はこのようになりました。
Gemini 1.5 Flashの結果はこちら
```python
import goslate
import MeCab
def translate_and_analyze(text):
"""
Translates English text to Japanese and performs morphological analysis.
Args:
text: The English text to translate.
Returns:
A dictionary containing the Japanese translation and morphological analysis.
"""
gs = goslate.Goslate()
japanese_text = gs.translate(text, 'ja')
# Morphological analysis using MeCab
tagger = MeCab.Tagger()
node = tagger.parseToNode(japanese_text)
analysis = []
while node:
analysis.append({
'surface': node.surface,
'feature': node.feature
})
node = node.next
return {
'translation': japanese_text,
'analysis': analysis
}
if __name__ == '__main__':
text = input("Enter English text: ")
result = translate_and_analyze(text)
print("Japanese Translation:", result['translation'])
print("Morphological Analysis:")
for entry in result['analysis']:
print(f" {entry['surface']} ({entry['feature']})")
```
**Explanation:**
1. **Import Libraries:**
- `goslate`: Used for English to Japanese translation.
- `MeCab`: Used for Japanese morphological analysis.
2. **`translate_and_analyze` Function:**
- Takes English text (`text`) as input.
- **Translation:**
- Initializes a `goslate.Goslate()` object.
- Translates the input text to Japanese using `gs.translate(text, 'ja')`.
- **Morphological Analysis:**
- Creates a `MeCab.Tagger()` object for analyzing Japanese text.
- Uses `tagger.parseToNode(japanese_text)` to parse the Japanese translation and get a node object.
- Iterates through the nodes:
- Extracts the surface form (`node.surface`) – the actual word.
- Extracts the feature information (`node.feature`) – details about the word's part of speech, inflection, etc.
- Stores the surface and feature in a dictionary.
- Adds the dictionary to the `analysis` list.
- Returns a dictionary containing the Japanese translation and the analysis list.
3. **Main Execution (`if __name__ == '__main__':`):**
- Prompts the user to enter English text.
- Calls `translate_and_analyze` to get the translation and analysis.
- Prints the Japanese translation.
- Prints the morphological analysis:
- Iterates through the `analysis` list.
- For each entry, prints the surface form and the feature information in a formatted way.
**Before Running:**
1. **Install `goslate`:** `pip install goslate`
2. **Install `MeCab`:** Follow the installation instructions for your system (typically involves downloading and compiling the source code).
3. **Install `mecab-python3`:** `pip install mecab-python3`
**How to Run:**
1. Save the code as a Python file (e.g., `translator.py`).
2. Open your terminal or command prompt.
3. Navigate to the directory where you saved the file.
4. Run the script: `python translator.py`
**Example Usage:**
```
Enter English text: I love to eat Japanese food.
Japanese Translation: 私は日本の食べ物が大好きです。
Morphological Analysis:
私は (私-名詞-一般, は-助詞-格助詞-主格)
日本 (日本-名詞-固有名詞)
の (の-助詞-連体化)
食べ物 (食べ物-名詞-一般)
が (が-助詞-格助詞-主格)
大好き (大好き-形容詞-自立)
です (です-助動詞-判定)
。 (。-記号-句点)
```
import goslate
import MeCab
def translate_and_analyze(text):
"""
Translates English text to Japanese and performs morphological analysis.
Args:
text: The English text to translate.
Returns:
A dictionary containing the Japanese translation and morphological analysis.
"""
gs = goslate.Goslate()
japanese_text = gs.translate(text, 'ja')
# Morphological analysis using MeCab
tagger = MeCab.Tagger()
node = tagger.parseToNode(japanese_text)
analysis = []
while node:
analysis.append({
'surface': node.surface,
'feature': node.feature
})
node = node.next
return {
'translation': japanese_text,
'analysis': analysis
}
if __name__ == '__main__':
text = input("Enter English text: ")
result = translate_and_analyze(text)
print("Japanese Translation:", result['translation'])
print("Morphological Analysis:")
for entry in result['analysis']:
print(f" {entry['surface']} ({entry['feature']})")
説明:
- ライブラリのインポート:
goslate
: 英語から日本語への翻訳に使用されます。MeCab
: 日本語の形態素解析に使用されます。
- 「translate_and_analyze」関数:
- 英語のテキスト (
text
) を入力として受け取ります。 - 翻訳:
goslate.Goslate()
オブジェクトを初期化します。gs.translate(text, 'ja')
を使用して入力テキストを日本語に翻訳します。- 形態素解析:
- 日本語テキストを解析するための
MeCab.Tagger()
オブジェクトを作成します。 tagger.parseToNode(japanese_text)
を使用して日本語翻訳を解析し、ノード オブジェクトを取得します。- ノードを反復処理します。
- 表面形式 (
node.surface
)、つまり実際の単語を抽出します。 - 特徴情報 (
node.feature
) を抽出します – 単語の品詞、語尾変化などの詳細。 - 表面とフィーチャを辞書に保存します。
- 辞書を「分析」リストに追加します。
- 表面形式 (
- 日本語訳と分析リストを含む辞書を返します。
- 英語のテキスト (
- メイン実行 (
if __name__ == '__main__':
):- ユーザーに英語のテキストを入力するように求めます。
translate_and_analyze
を呼び出して、翻訳と分析を取得します。- 日本語訳を印刷します。
- 形態素解析を出力します。
- 「分析」リストを反復処理します。
- エントリごとに、表面形状とフィーチャー情報をフォーマットされた方法で印刷します。
実行前:
goslate
をインストールします:pip install goslate
MeCab
をインストールします: システムのインストール手順に従います (通常、ソース コードのダウンロードとコンパイルが含まれます)。mecab-python3
をインストールします:pip install mecab-python3
実行方法:
- コードを Python ファイルとして保存します (例:
translator.py
)。 - ターミナルまたはコマンド プロンプトを開きます。
- ファイルを保存したディレクトリに移動します。
- スクリプト「python translator.py」を実行します。
使用例:
Enter English text: I love to eat Japanese food.
Japanese Translation: 私は日本の食べ物が大好きです。
Morphological Analysis:
私は (私-名詞-一般, は-助詞-格助詞-主格)
日本 (日本-名詞-固有名詞)
の (の-助詞-連体化)
食べ物 (食べ物-名詞-一般)
が (が-助詞-格助詞-主格)
大好き (大好き-形容詞-自立)
です (です-助動詞-判定)
。 (。-記号-句点)
GPT-4oの結果はこちら
Here is a Python program that translates English text into Japanese and performs morphological analysis using `googletrans` for translation and `mecab-python3` for morphological analysis:
### Requirements:
1. `googletrans` for translation.
2. `mecab-python3` for morphological analysis.
### Installation:
Install the required Python packages using `pip`:
```bash
pip install googletrans==4.0.0-rc1
pip install mecab-python3
```
### Python Program:
Below is the complete Python script:
```python
from googletrans import Translator
import MeCab
def translate_text(text, dest='ja'):
translator = Translator()
translation = translator.translate(text, dest=dest)
return translation.text
def morphological_analysis(text):
tagger = MeCab.Tagger()
return tagger.parse(text)
def main():
# Input English text
english_text = "Hello, how are you?"
print("English Text:", english_text)
# Translate English to Japanese
japanese_translation = translate_text(english_text)
print("Translated Text:", japanese_translation)
# Perform morphological analysis on the Japanese text
analysis = morphological_analysis(japanese_translation)
print("Morphological Analysis:\n", analysis)
if __name__ == "__main__":
main()
```
### Explanation:
- **Translation**: The `translate_text` function uses `googletrans` to translate an English string into Japanese.
- **Morphological Analysis**: The `morphological_analysis` function uses `MeCab` to perform morphological analysis on the translated Japanese text.
- **Main Function**: Combines these processes and prints the original English text, its Japanese translation, and the morphological analysis of the Japanese text.
This program allows you to input English text, get its Japanese translation, and analyze the structure of the translated text using morphological analysis.
英語のテキストを日本語に翻訳し、形態素解析を行うプログラムを以下に示します。このプログラムでは、翻訳に googletrans
ライブラリを使用し、形態素解析に mecab-python3
ライブラリを使用します。
必要条件:
googletrans
:翻訳用mecab-python3
:形態素解析用
インストール:
以下のコマンドを使用して必要なPythonパッケージをインストールします。
pip install googletrans==4.0.0-rc1
pip install mecab-python3
Pythonプログラム:
以下に完全なPythonスクリプトを示します。
from googletrans import Translator
import MeCab
def translate_text(text, dest='ja'):
translator = Translator()
translation = translator.translate(text, dest=dest)
return translation.text
def morphological_analysis(text):
tagger = MeCab.Tagger()
return tagger.parse(text)
def main():
# 英語のテキストを入力
english_text = "Hello, how are you?"
print("英語のテキスト:", english_text)
# 英語を日本語に翻訳
japanese_translation = translate_text(english_text)
print("翻訳されたテキスト:", japanese_translation)
# 翻訳された日本語テキストの形態素解析を実行
analysis = morphological_analysis(japanese_translation)
print("形態素解析:\n", analysis)
if __name__ == "__main__":
main()
説明:
- 翻訳:
translate_text
関数は、googletrans
を使用して英語の文字列を日本語に翻訳します。 - 形態素解析:
morphological_analysis
関数は、MeCab
を使用して翻訳された日本語テキストの形態素解析を行います。 - メイン関数: オリジナルの英語テキスト、翻訳された日本語テキスト、およびその形態素解析結果を表示します。
このプログラムを使用すると、英語のテキストを入力して日本語に翻訳し、翻訳されたテキストの構造を形態素解析で分析することができます。
Gemini 1.5 Flashの出力したコードは、基本的には正しく形態素解析を実行できますが、翻訳で使用しているgoslateは、もうメンテナンスされていない古いツールなので、動作しない可能性があります。
このような場合は、googletransを使うのが一般的だと思われます。
GPT-4oのコードは、googletransとMecabを使用した一般的な形態素解析を実装しています。
こちらも基本的には正しく動作しますが、エラーハンドリング処理が無いなど、完璧とは言えません。
今回の検証の結果、Gemini 1.5 FlashはGPT-4oに引けを取らない性能を有していることが分かりました。
これで軽量版なのですから驚きです。
もしこの結果を見てGemini 1.5 Flashが気になる方は、非常に気軽に使用できるので、是非試してみてください!
なお、最新のGPT-4oについて知りたい方は、以下の記事をご覧ください。
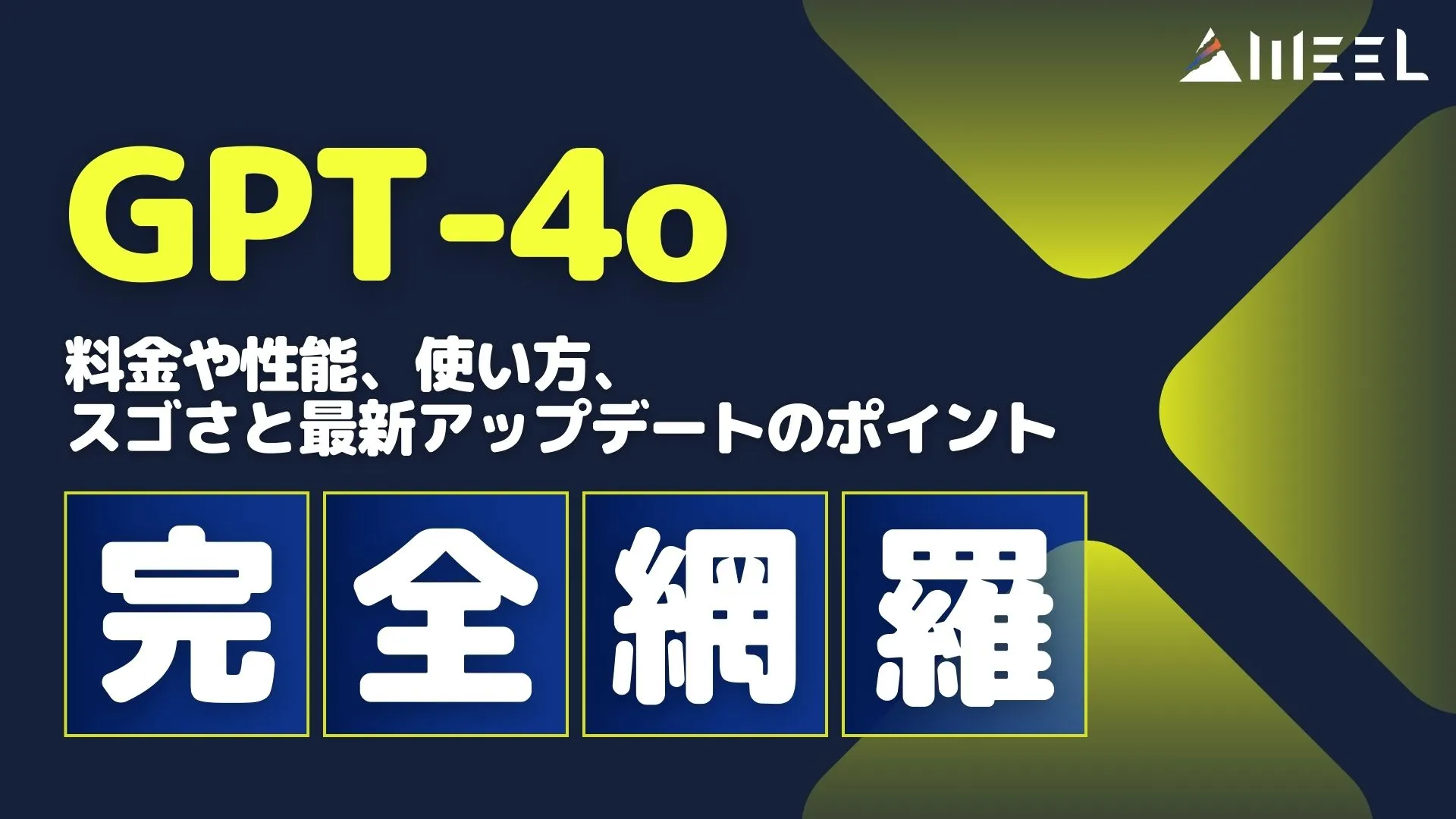
Gemini 1.5 Flashは性能とコスト効率を兼ね備えた次世代モデル
Gemini 1.5 Flashは、2024年5月15日に開催されたGoogle I/Oで発表された新しいAIモデルです。
このモデルは、Geminiシリーズのなかで最も軽量であり、応答の速さと非常に高いコスト効率を併せ持ちながら、100万トークンのコンテキストウィンドウを維持しています。
また、画像や動画、音声などの入力にも対応し、マルチモーダル推論が可能になっています。
長大なコンテキストウィンドウにより、1時間のビデオや11時間のオーディオ入力に対応しています。
さらに、ベンチマークでGemini 1.0 Ultraと同等のスコアを獲得するなど、軽量化しながら性能も確保し、高いコストパフォーマンスを持ち合わせています。
その利用料金は、Gemini 1.5 Proの1/10に抑えられています。
実際に使ってみたところ、GPT-4oにも引けを取らない非常に高い性能を有していることが分かりました。
この記事を読んで、Gemini 1.5 Flashが気になった方は是非試してみてください!
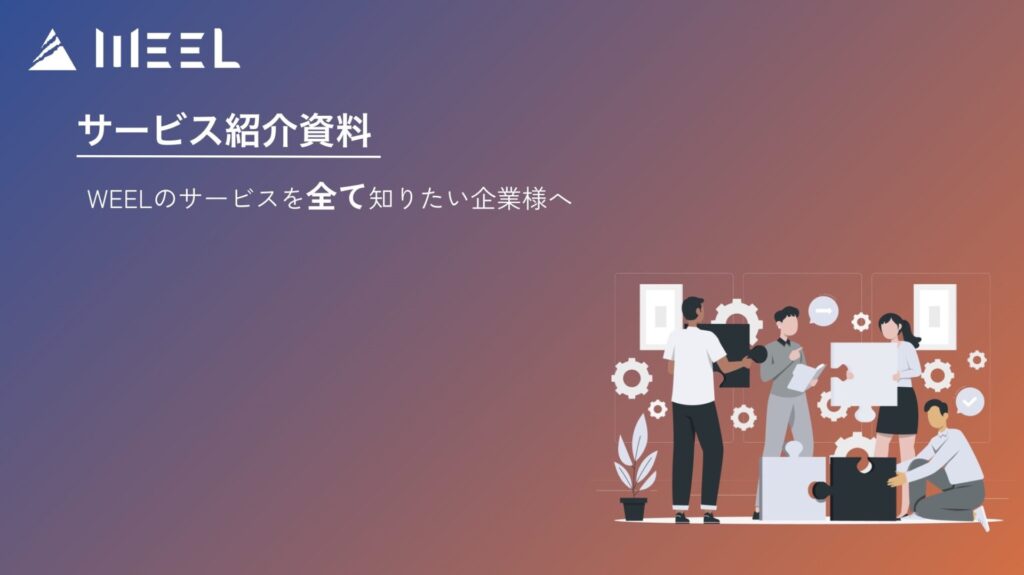
生成系AIの業務活用なら!
・生成系AIを活用したPoC開発
・生成系AIのコンサルティング
・システム間API連携
最後に
いかがだったでしょうか?
GPT-3.5 Turboの最新アップデートで、より高速かつ低コストでのAI活用が可能になりました。自社での導入・活用を検討する際に、最適なモデル選定や活用方法について、一緒に考えてみませんか?
弊社では
・マーケティングやエンジニアリングなどの専門知識を学習させたAI社員の開発
・要件定義・業務フロー作成を80%自動化できる自律型AIエージェントの開発
・生成AIとRPAを組み合わせた業務自動化ツールの開発
・社内人事業務を99%自動化できるAIツールの開発
・ハルシネーション対策AIツールの開発
・自社専用のAIチャットボットの開発
などの開発実績がございます。
まずは、「無料相談」にてご相談を承っておりますので、ご興味がある方はぜひご連絡ください。
➡︎生成AIを使った業務効率化、生成AIツールの開発について相談をしてみる。
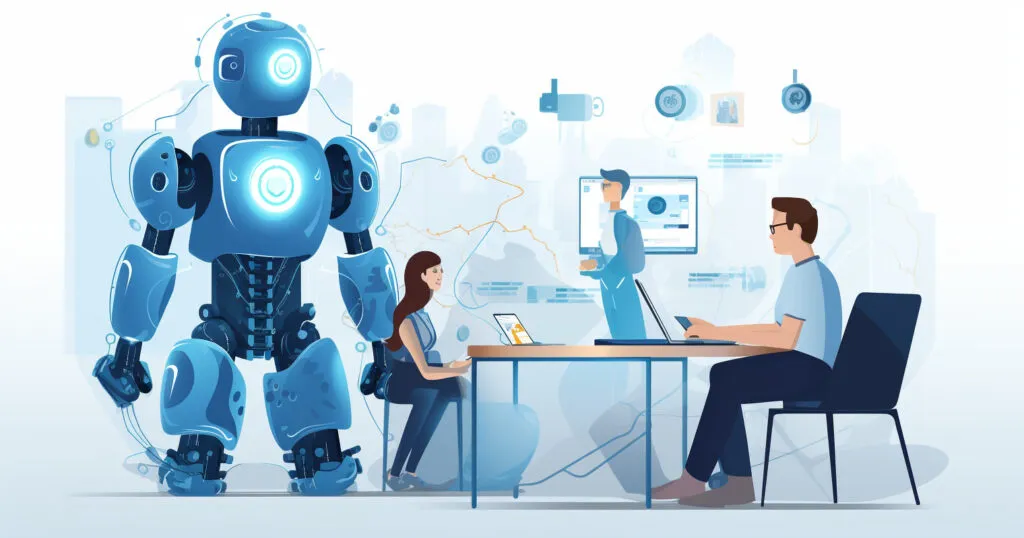
「生成AIを社内で活用したい」「生成AIの事業をやっていきたい」という方に向けて、生成AI社内セミナー・勉強会をさせていただいております。
セミナー内容や料金については、ご相談ください。
また、サービス紹介資料もご用意しておりますので、併せてご確認ください。