【Gemini 1.5 Pro】100万トークン対応のGoogle最強LLMをGPT-4oと比較してみた
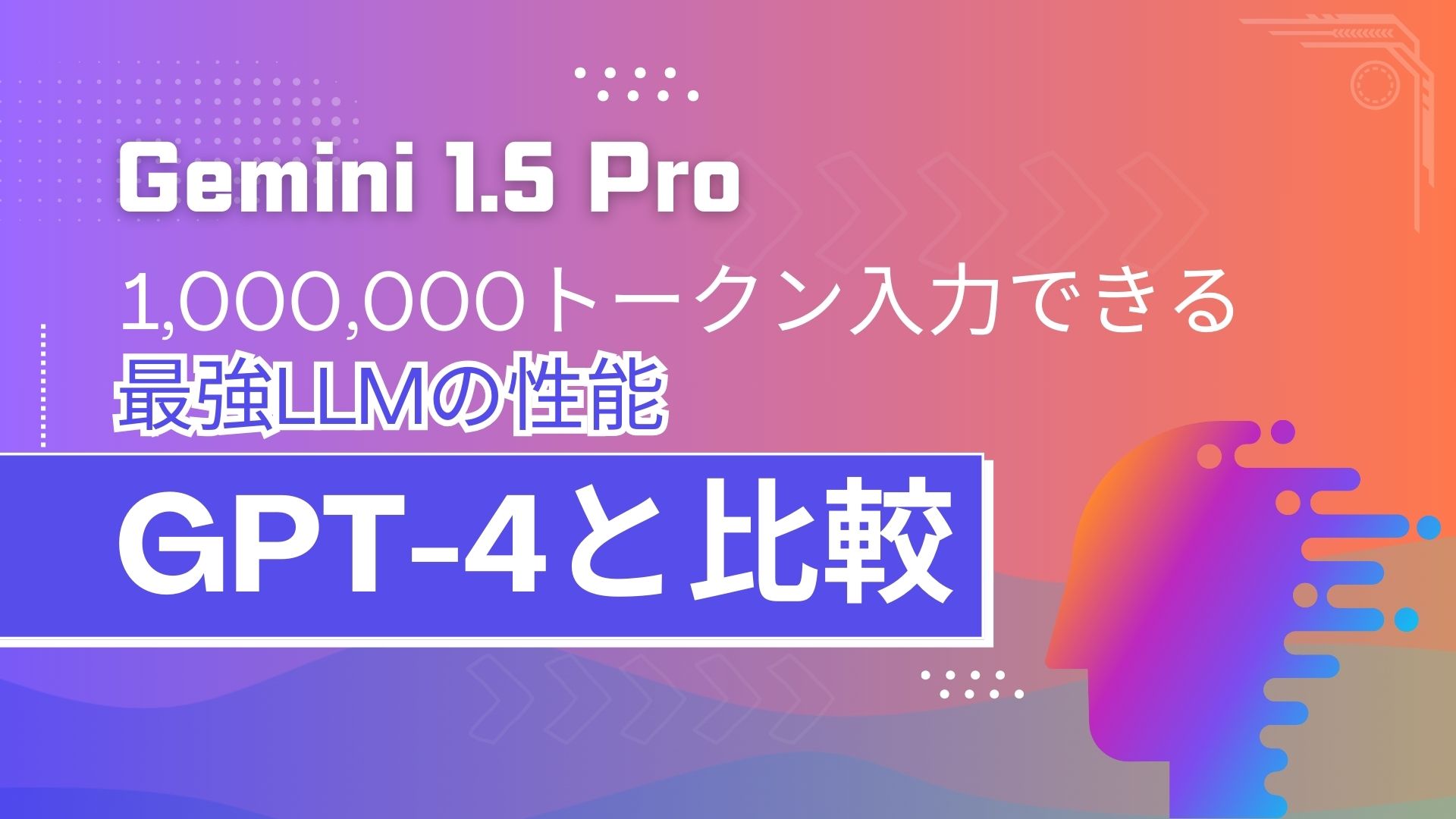
WEELメディア事業部AIライターの2scです。
みなさん、GoogleがUltra 1.0の発表からわずか1週間で、Geminiの最新版を発表しました。それが、1,000,000トークンまで入力できちゃう「Gemini 1.5 Pro」です。
なんと現在公開中の中堅・Gemini 1.5 Proでも、Ultra 1.0相当のスペックを誇るんだとか……
当記事では、そんなGemini 1.5 Proのスペックからできることまでをお届けしていきます!完読いただくと、マルチモーダル生成AIのイメージが変わるかも……しれません。
ぜひ最後までお読みくださいね。
\生成AIを活用して業務プロセスを自動化/
Gemini 1.5 Proの概要
米国時間の2024年2月15日、Google製マルチモーダル生成AI(MLLM)の最新版「Gemini 1.5」シリーズが登場!同時に中型モデルの「Gemini 1.5 Pro」が公開されています。
そのすごさは、というと……
● 性能テストで先代の大型モデル・Ultra 1.0と互角
● Transformerの進化系、MoEアーキテクチャを搭載
● LLM史上最大、100万トークンもの入力に対応
を中堅のGemini 1.5 Proで実現している点にあるんです。次項にて、詳しくみていきましょう!
参考記事:Introducing Gemini 1.5, Google’s next-generation AI model
Gemini 1.5 Proの特徴
ここからは、先行公開された中型モデル「Gemini 1.5 Pro」の特徴を3つ紹介していきます。まずは現行のトップモデル、Gemini Ultra 1.0との比較結果をみていきましょう!
Ultra 1.0と互角の性能
Gemini 1.5 Proは中型モデルですが、先代の旗艦モデルUltra 1.0と互角のスペックを誇ります。
そもそもUltra 1.0は、Gemini 1.5 Proのちょうど一週間前に公開されたばかりの……
- 性能比較全32項目のうち30項目で、GPT-4に勝利
- 理数&人文全57科目の問題集「MMLU」にて専門家に勝利
という高性能モデルでした。それを今回のGemini 1.5 Proと比較したところ……
このように基本的な能力(Core Capabilities)については、Gemini 1.5 ProもGemini Ultra 1.0も五分五分なんです!
しかもテキスト処理(Text)の比較では、13項目中10項目にてGemini 1.5 Proが勝っています。
参考記事:Gemini 1.5: Unlocking multimodal understanding across millions of tokens of context
MoEアーキテクチャ
Gemini 1.5シリーズと従来のLLM(Ultra 1.0・GPT-4等)とでは、処理のしくみが違います。
具体的には処理に用いられるひな形が異なっていて……
Gemini 1.5シリーズ | 従来のLLM | |
---|---|---|
処理のひな形 | MoE(Mixture-of-Experts)アーキテクチャ | Transformer |
処理のしくみ | タスク別に小さなネットワークをもっていて、要領よく使い分ける | どんなタスクも、ネットワークを100%使いきって全力解決 |
以上のとおり、Gemini 1.5シリーズのほうがスマートなんです!
100万トークンの入力に対応
MoEアーキテクチャを採用した結果、Gemini 1.5 Proでは入力できるトークン数が大幅にUP!一回に100万トークンの入力が実現しました。
そんなGemini 1.5 Proでは……
- 1時間の動画
- 11時間の音声データ
- 30,000行以上のソースコード
- 70万語以上の英単語
が丸々入力できてしまいます。
ちなみに実験下ではLLM史上最大、10M(なんと1,000万)トークンもの入力に成功しています。たとえば下図の横軸、処理できたトークン数(Tokens)をご覧ください。
このように中堅・Gemini 1.5 Proでも、GPT-4 Turboに圧勝しているんです。
なお、比較対象のGPT-4 Turboについて詳しく知りたい方は、下記の記事を合わせてご確認ください。
→【GPT 4 Turbo】ChatGPT最強モデル!APIの使い方や料金、できることを解説
Gemini 1.5 ProとGPT-4、Claude 2との性能比較
2024年2月時点で、Gemini 1.5のライバルに当たるLLMは……
- マルチモーダル対応の「GPT-4」
- 入力トークン数200Kの「Claude 2.1」
の2モデルです!これらとGemini 1.5、そして先代Gemini 1.0で性能を比較すると……
Gemini 1.5 | Gemini 1.0 | GPT-4 | Claude 2.1 | |
---|---|---|---|---|
入力トークン数 | 1,000,000 (Pro) | 32,000 | 128,000 (Turbo) | 200,000 |
モダリティ (入力媒体) | ・テキスト ・ソースコード ・画像 ・動画 ・音声 | ・テキスト ・ソースコード ・画像 ・動画 ・音声 | ・テキスト ・ソースコード ・画像 | ・テキスト ・ソースコード |
上表のとおり入力トークン数とモダリティの双方で、Gemini 1.5が勝っているんです!ちなみに入力トークン数については、Googleがわかりやすい図を出してくれています。
やはり、Gemini 1.5 Proは圧倒的ですね。
なお、比較対象のClaude 2.1について詳しく知りたい方は、下記の記事を合わせてご確認ください。
→【Claude 2.1】ChatGPT超えAIの日本語での使い方やAPIの使用方法、GPT-4との比較を解説
Gemini 1.5 Proにできること
100万トークンの入力に対応したGemini 1.5 Proなら……
- 文書400ページ丸ごとの解析
- 動画1時間の読み込み
- ソースコード100,000行の修正
- Few-shotでのカラマン語習得
といったことができちゃいます。それぞれGoogle公式のデモを、みていきましょう!
文書400ページ丸ごとの解析
Gemini 1.5 Proなら、全402ページに及ぶアポロ11号の記録が丸ごと読み込めてしまいます!
実際にPDF形式で読み込ませたのち、英語で「見どころを3つ探して引用リストを作って」と命令してみると……
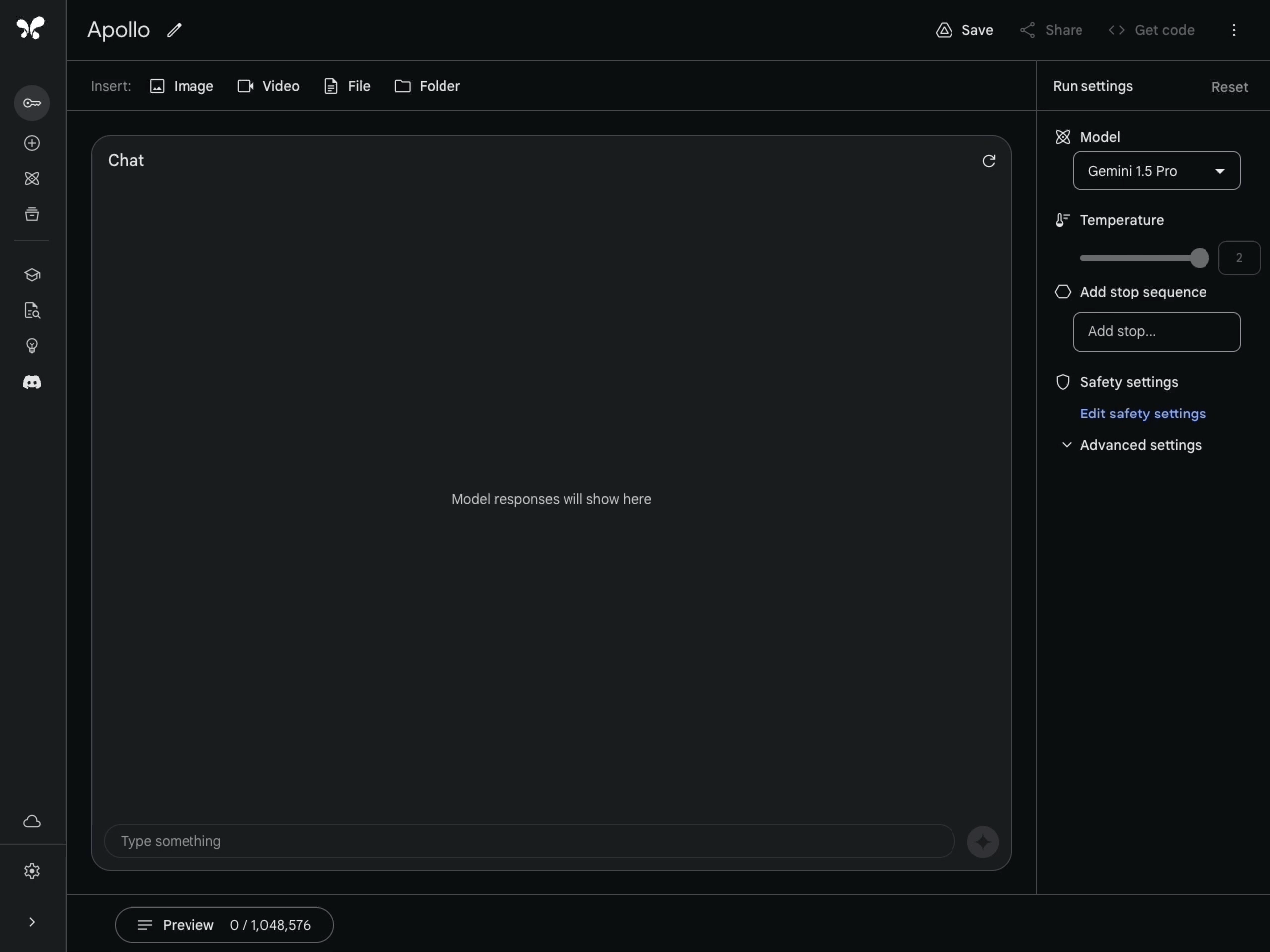
アポロ 11 号 空地音声記録の面白い瞬間:
- 「セシル・B・デアルドリンは指示を待っています。」(バズ・ライトイヤーが三人称で自分自身を指して)
- 「バッテリー B の充電が十分になったと思われるときに歌ってください。」(バズ・ライトイヤーがミッションコントロールに冗談めかして歌うように頼む)
- 「皇帝は歯磨きをしているので、私が代わりに話します。」(マイク・コリンズが、プラウダのニュース記事を読んだ後、ニール・アームストロングを「皇帝」と呼んで)
このように記録の細部まで、読み込んでくれます。さらに手描きイラストを使った引用もできて……
引用箇所も正確!まさしく、ムーンショットです。
動画1時間の読み込み
Gemini 1.5 Proは最大1時間まで、動画が読み込めます。試しにバスター・キートンの無声映画『Sherlock Jr. / キートンの探偵学入門』全44分を、MP4形式で入力すると……
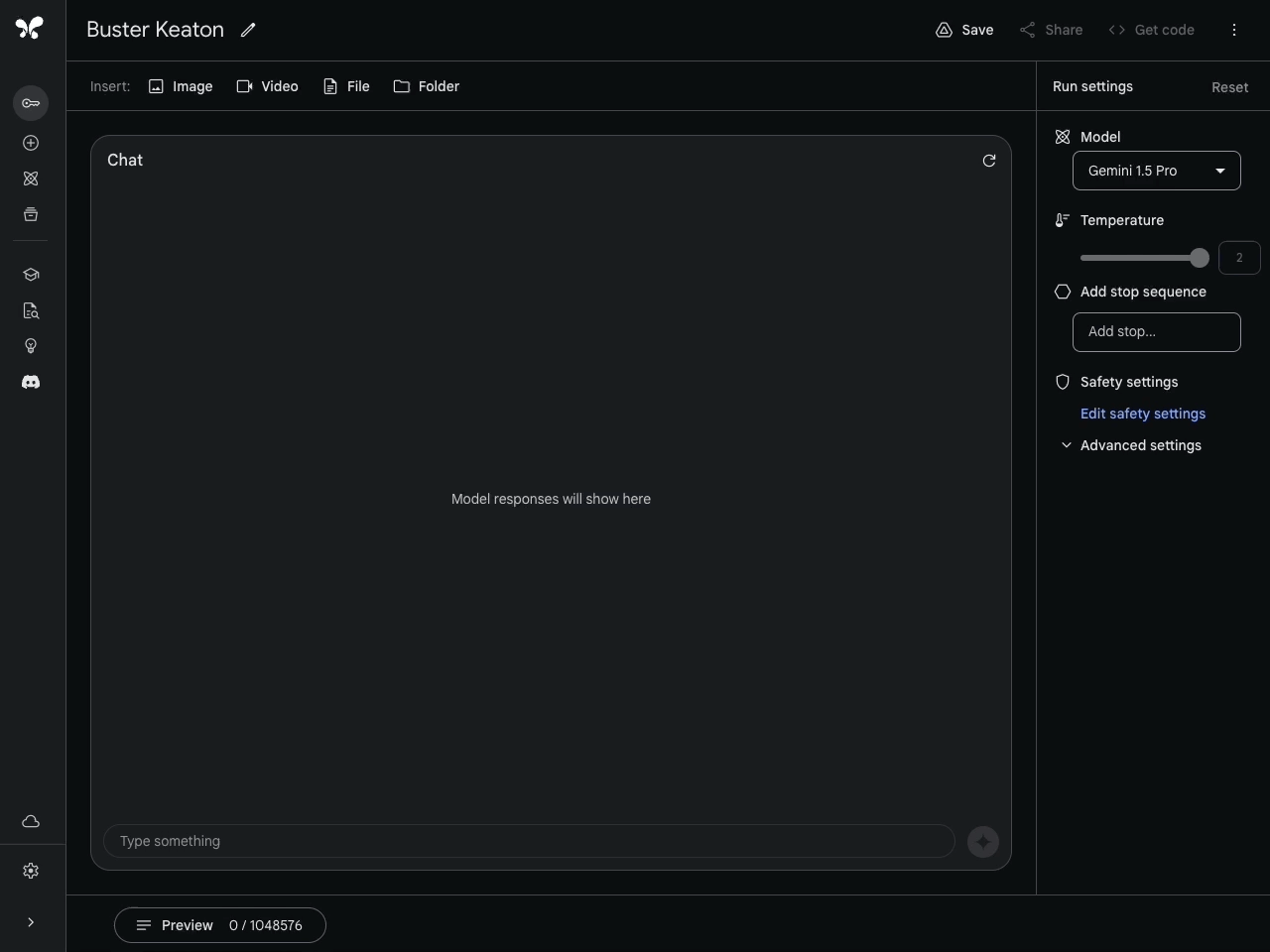
以上のとおり、Gemini 1.5 Proはスムーズに読み込んでくれます。
さらにそこから、ワンシーンを探し出すことが可能。「紙片がポケットから抜き取られたシーン」「手描きの線画と同じシーン」についてそれぞれ、タイムスタンプを取得してもらうと……
上の動画のように、Gemini 1.5 Proは正しくシーンを引用してくれるんです!
YouTubeにGemini 1.5 Proが実装されれば、切り抜き師が失業してしまうかも。動画投稿者のみなさん、今後の動向は要チェックです!
ソースコード100,000行の修正
Gemini 1.5 Proの場合、ソースコードは100,000行まで読み込みが可能です。
試しに3DアニメーションのJavaScriptコード(全816,511トークン)を入力してみましょう!
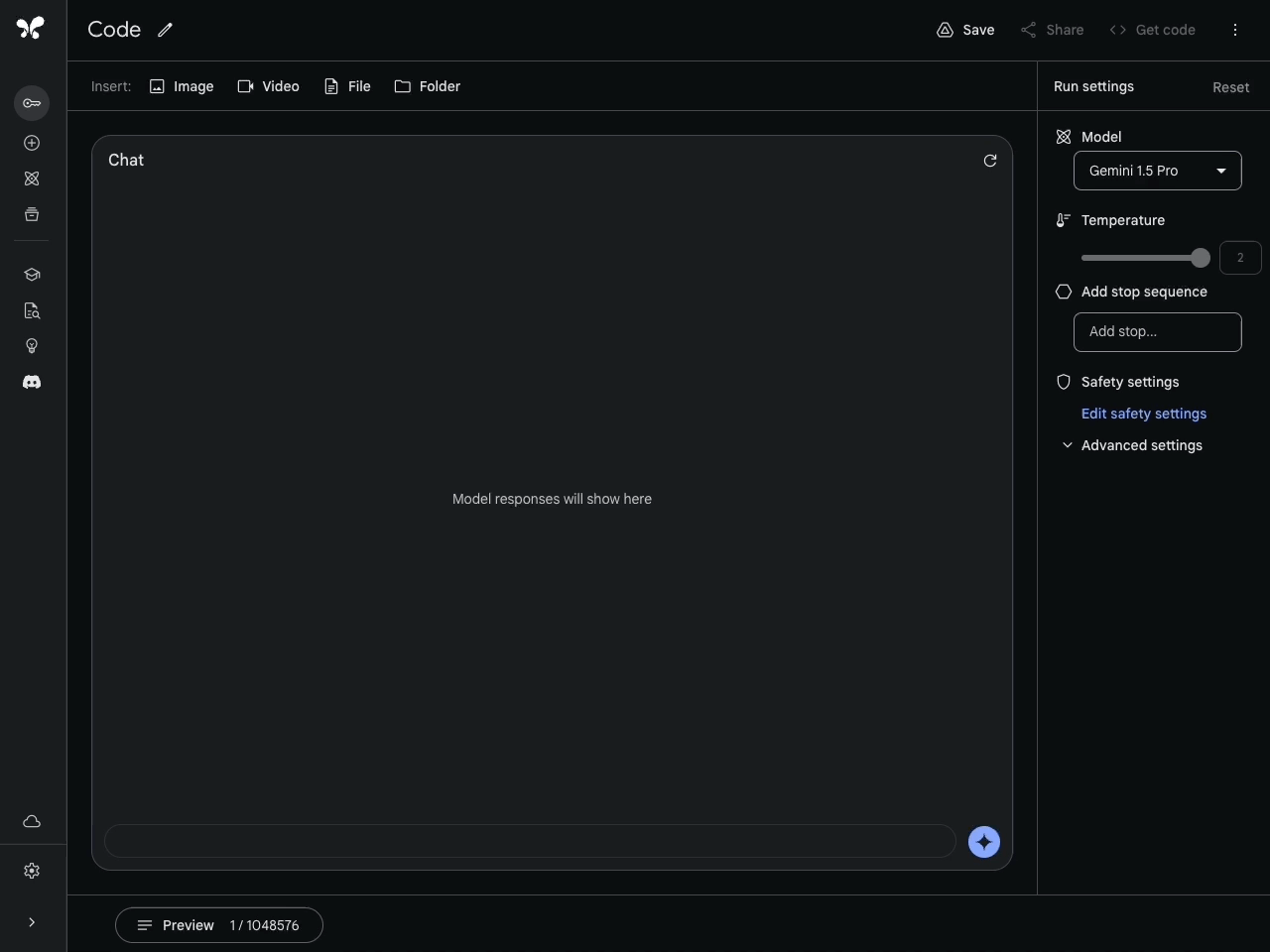
するとこのように、読み込みが完了します。続けてGemini 1.5 Proに「地形を平らにするための変更箇所は?」と聞いてみると……
画像のコードは、地形の高さを生成する関数を定義しています。この関数を変更することで、地形を平坦にすることができます。
具体的には、以下の方法があります。
- ノイズ関数の振幅を減らす
- quality 変数を調整する
- z 変数を調整する
以下に、それぞれの方法について説明します。
ノイズ関数の振幅を減らす
ノイズ関数は、地形にランダムな起伏を生成するために使用されます。ノイズ関数の振幅を減らすことで、起伏を小さくし、地形を平坦にすることができます。
具体的には、以下のコードを変更します。(以下略)
お見事!Gemini 1.5 Proは、具体的な修正案を出してくれるんです!その他コーディングの事例は、以下の動画をご覧ください。
コーディングを生成AIに丸投げできる時代が、着々と近づいてきてますね……
Few-shotでのカラマン語習得
一般にLLMは、プロンプトでいくつかの例を示す(Few-shot)だけで、未知のタスクにも対処が可能です。たとえばChatGPTやGemini 1.0に、学習範囲外の言葉を教えると……
「whatpu」とはタンザニア固有の小さくて毛皮のある動物です。
「whatpu」という言葉を使った文の例は次のとおりです。
私たちはアフリカを旅行して、これらのとてもかわいいwhatpusを見ました。
「farduddle」というのは、とても速く上下にジャンプすることを意味します。
「farduddle」という言葉を使用した文の例は次のとおりです。
このようにそれぞれ、教わってすぐの言葉で例文を作ってくれます。
そこからさらに入力トークン数が増えたGemini 1.5 Proなら、Few-shotでも……
- 未知言語の文法マニュアル
- 未知言語の辞書
が丸ごと入力可能!試しに、全世界での話者数が200人以下の「カラマン語」をFew-shotで示してみると……
上図のとおり「パンダナスの葉を手に入れたので、マットを作りたい」という英文をカラマン語に訳してくれちゃうんです!
Few-shotといえば、AIチャットボットや自動FAQの根幹をなす技術。Gemini 1.5 Proは生成AIの業務活用においてゲームチェンジャーとなるかもしれません。
Gemini 1.5 Proは本当に100万トークンを入力できるのか?
ここでは、Gemini 1.5 Proが本当に100万近くのトークン数を入力できるのかを確かめるために、警視庁生活安全総務課が公開しているメールけいしちょうのオープンデータ(メールけいしちょうで配信した内容を、オープンデータとして公開したもの)から、100万文字近くのメール文章を抽出し、Gemini 1.5 Proに読み込ませます。
ちなみに、Google AI Studioの左下の「Preview」で、トークン数を確認できます。
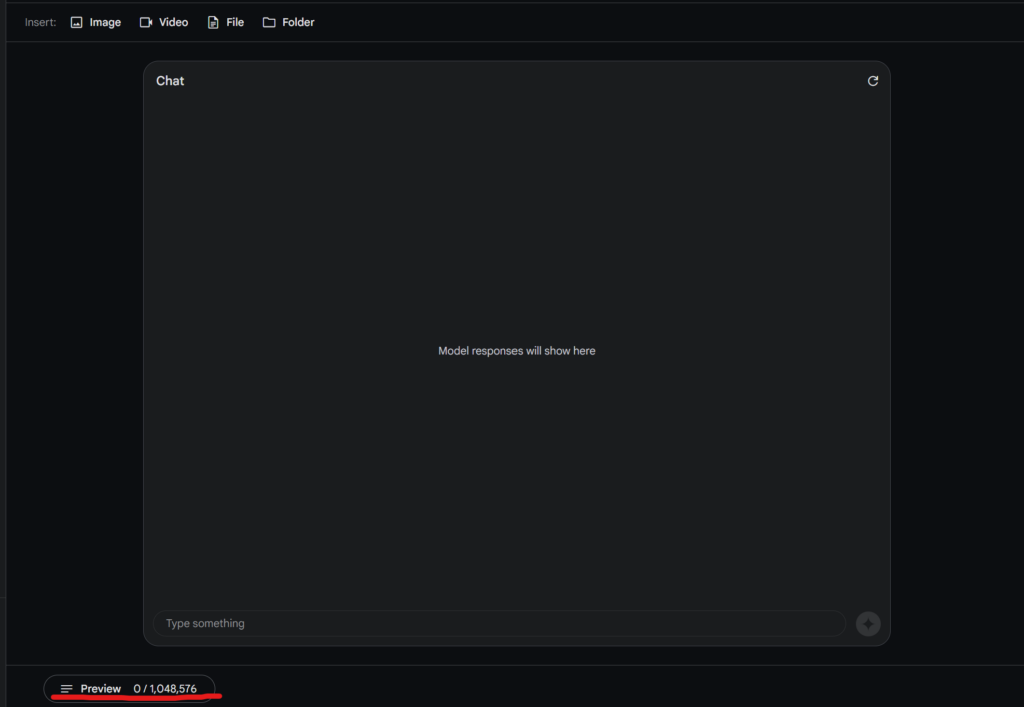
メール文章から、最も多いトピックを教えてもらいます。使用したプロンプトは、以下の通りです。
From the following email text data, what are the most common email messages?
和訳:
以下のメール文章データから、最も多いメールの内容を教えてください。
上記のプロンプトに続けて、メールデータ全文を張り付けて、送信したところ、「No content」と表示され、上手く読み込めなかったです。
そこで、以下の赤枠の部分で、PDFを読み込ませてみました。
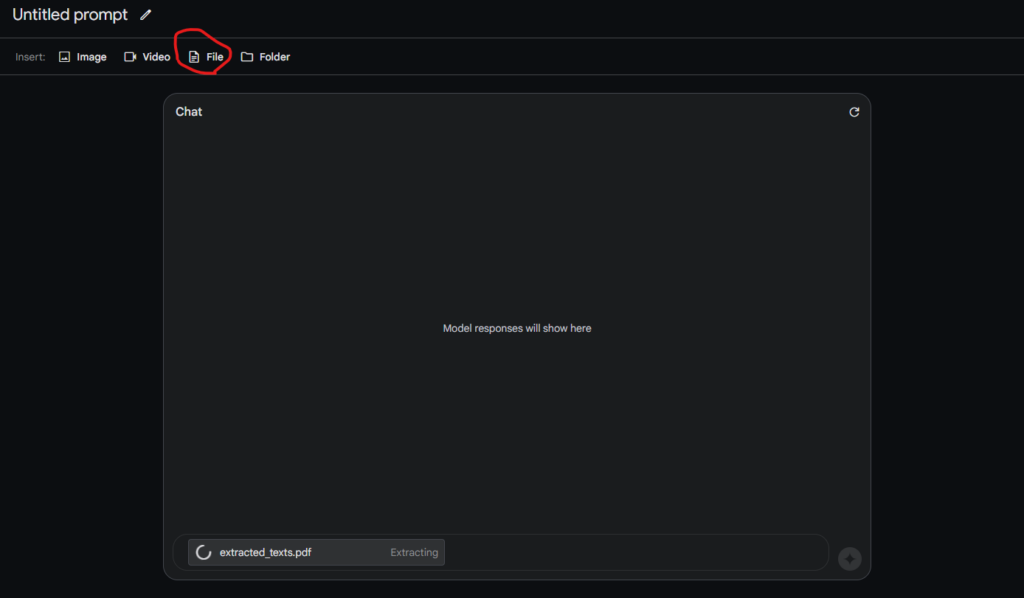
それでも同じように、うまく読み込めなかったです。
そこで、100万文字の英語の文章で試してみます。具体的には、プロジェクト・グーテンベルグ(Project Gutenberg)に掲載されている洋書を読ませ、内容を要約させてみます。
そうすることで、上手く読み込めました。結果は以下の通りです。
結果はこちら
The uploaded text is an ebook titled "Myths of Northern Lands" by H. A. Guerber. It provides a comprehensive overview of Norse mythology, narrating the stories of the creation of the world, the lives and adventures of the gods, and their eventual downfall in Ragnarok.
The book is organized into chapters, each focusing on a specific god or group of beings within the mythology, such as Odin, Thor, Freya, the Valkyries, and the giants. The myths are retold in detail, with references to their influence on customs, arts, and literature. Additionally, the book compares Norse mythology to Greek mythology, highlighting similarities and differences between the two belief systems.
The ebook also includes illustrations, a glossary of terms, and an index for easy reference.
和訳:
アップロードされたテキストは、H.A.ゲルバー著『北方神話』というタイトルの電子書籍です。この本は北欧神話の包括的な概観を提供し、世界の創造、神々の生活と冒険、そしてラグナロクにおける最終的な滅亡の物語を叙述している。
本書は各章で構成され、それぞれがオーディン、ソー、フレイヤ、ヴァルキュリア、巨人など、神話の中の特定の神や存在のグループに焦点を当てている。神話は、風習、芸術、文学への影響に言及しながら詳細に語られる。さらに、北欧神話とギリシャ神話を比較し、2つの信仰体系の類似点と相違点を強調している。
この電子書籍には、イラスト、用語集、索引もあり、簡単に参照できます。
長時間の動画を読み込ませてみた
ちなみに、以下の赤枠の部分から、動画もアップロードできます。
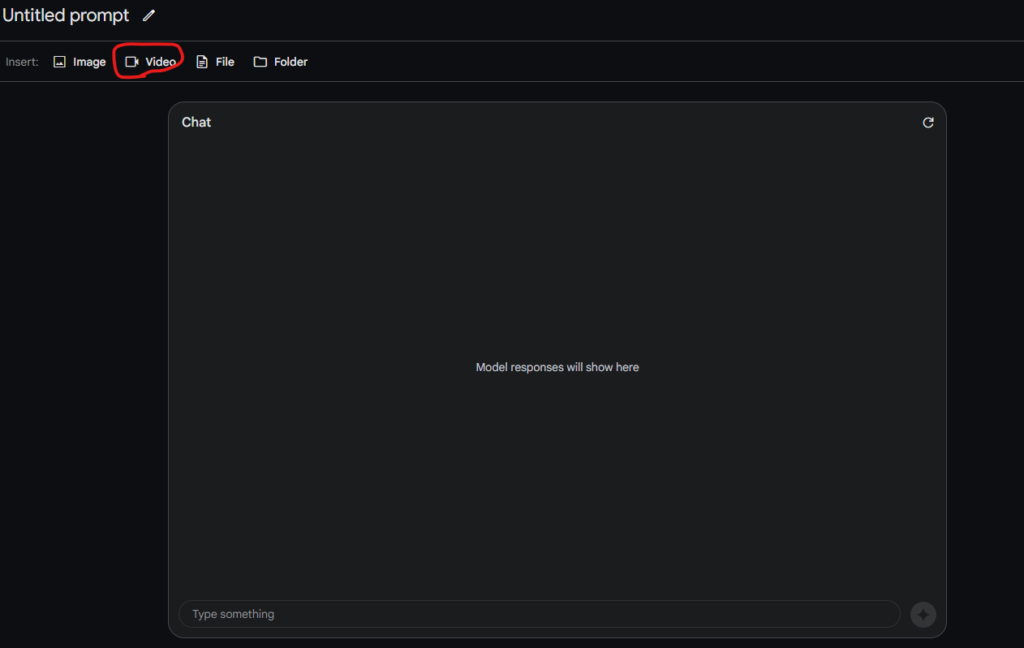
試しに以下の30分程度の動画を読み込ませ、内容を要約してもらいます。最初に、Y2Meta.appで動画をmp4に変換します。
読み込ませた際に、以下の様に動画のトークン数も表示されます。
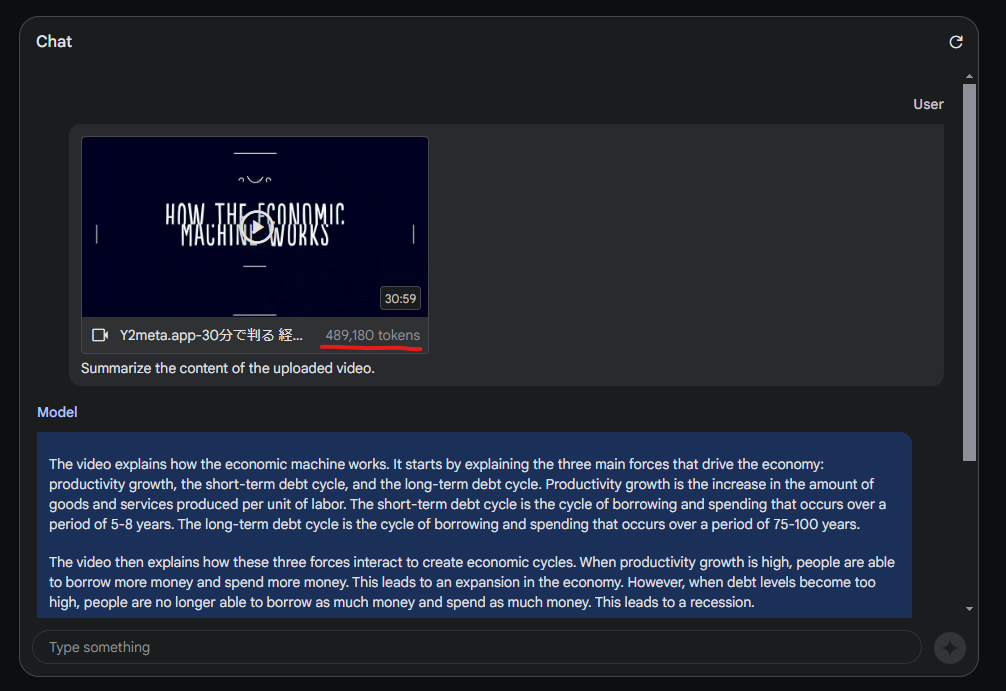
結果は以下の通りです。
結果はこちら
The video explains how the economic machine works. It starts by explaining the three main forces that drive the economy: productivity growth, the short-term debt cycle, and the long-term debt cycle. Productivity growth is the increase in the amount of goods and services produced per unit of labor. The short-term debt cycle is the cycle of borrowing and spending that occurs over a period of 5-8 years. The long-term debt cycle is the cycle of borrowing and spending that occurs over a period of 75-100 years.
The video then explains how these three forces interact to create economic cycles. When productivity growth is high, people are able to borrow more money and spend more money. This leads to an expansion in the economy. However, when debt levels become too high, people are no longer able to borrow as much money and spend as much money. This leads to a recession.
The video then explains how the central government and central bank can manage the economy by controlling interest rates and printing money. When the economy is in a recession, the central government can cut spending and reduce debt. The central bank can also lower interest rates to make it easier for people to borrow money. When the economy is in an expansion, the central government can increase spending and the central bank can raise interest rates to slow down the economy.
The video concludes by explaining that the most important part of the economy is productivity growth. If productivity growth is high, the economy will be able to grow and prosper. However, if productivity growth is low, the economy will be more likely to experience recessions and depressions.
和訳:
このビデオは、経済マシンがどのように機能するかを説明している。まず、経済を動かす3つの主要な力、すなわち生産性の成長、短期債務サイクル、長期債務サイクルについて説明する。生産性の成長とは、単位労働量当たりに生産される財やサービスの量の増加である。短期債務サイクルとは、5~8年の期間で発生する借入と支出のサイクルである。長期債務サイクルとは、75~100年にわたる借入と支出のサイクルのことである。
このビデオでは、これら3つの力がどのように相互作用して経済サイクルを生み出しているかを説明している。生産性の伸びが高いとき、人々はより多くのお金を借り、より多くのお金を使うことができる。これが経済の拡大につながる。しかし、負債水準が高くなりすぎると、人々はより多くのお金を借り、より多くのお金を使うことができなくなる。これが景気後退につながる。
ビデオは次に、中央政府と中央銀行が金利をコントロールし、お金を印刷することによって経済を管理する方法を説明します。景気が後退しているとき、中央政府は支出を削減し、負債を減らすことができる。中央銀行はまた、人々がお金を借りやすくするために金利を引き下げることができる。景気が拡大しているときは、中央政府は支出を増やし、中央銀行は金利を上げて景気を減速させることができる。
ビデオは最後に、経済で最も重要なのは生産性の伸びであると説明している。生産性の伸びが高ければ、経済は成長し繁栄することができる。しかし、生産性の伸びが低ければ、経済は不況や恐慌に見舞われる可能性が高くなる。
次に、以下の50分近くあるゲーム実況動画を読み込ませてみます。
ここでは、「このゲーム実況の見どころ」と「配信者の印象」をGemini 1.5 Proに聞いてみましょう。こちらは約80万トークンありました。
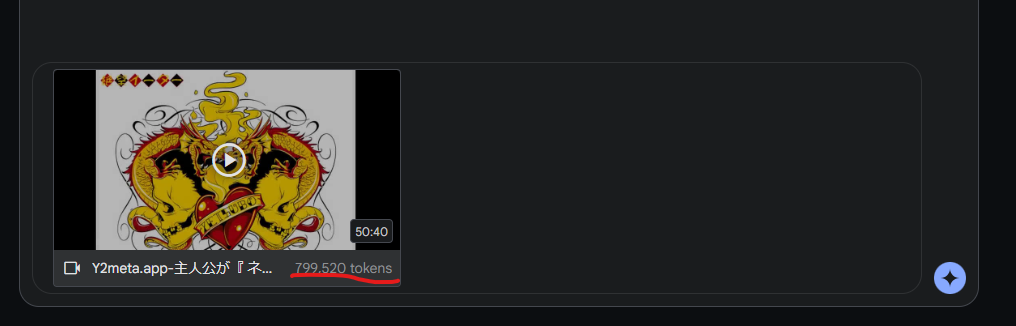
使用したプロンプトは、以下の通りです。
The video file I uploaded contains content from a live game stream uploaded to YouTube. Please tell us “Highlights of this game stream” and “Impression of the distributor” with reference to that game stream video.
和訳:
私がアップロードした動画ファイルには、YouTubeにアップロードされたゲーム実況配信のコンテンツが含まれています。そのゲーム実況配信動画を参考に、「このゲーム実況の見どころ」と「配信者の印象」を教えてください。
結果は以下の通りです。
結果はこちら
Highlights of this game stream
The video shows a playthrough of the game "Tsukihime -A piece of blue glass moon-", a visual novel released in 2021. The streamer plays through the game's prologue and first chapter, introducing the main characters and setting up the story.
The highlights of the stream include:
The streamer's commentary, which is both informative and entertaining. They provide insights into the game's story and characters, as well as their own personal thoughts and reactions.
The game's beautiful visuals and soundtrack. The game's art style is distinctive and visually appealing, and the soundtrack is atmospheric and well-composed.
The game's intriguing story. The game's prologue and first chapter introduce a number of mysteries and plot threads that leave the player wanting to learn more.
Impression of the distributor
The streamer is clearly a fan of the game and is passionate about sharing it with their viewers. They are knowledgeable about the game's lore and characters, and they provide insightful commentary throughout the stream. They are also engaging and entertaining, and they interact with their viewers in a friendly and welcoming manner. Overall, the streamer gives a positive impression and makes the game seem even more appealing to potential players.
和訳:
このゲームストリームの見どころ
2021年発売のビジュアルノベル『月姫 -A piece of blue glass moon-』のプレイ動画。ゲームのプロローグと第1章をプレイし、主人公の紹介やストーリーの設定などを行っている。
ストリーミングのハイライトは以下の通り:
ストリーマーによる解説。ゲームのストーリーやキャラクターについての洞察や、彼ら自身の個人的な考えやリアクションを提供してくれる。
ゲームの美しいビジュアルとサウンドトラック。ゲームのアートスタイルは特徴的で視覚的に魅力的であり、サウンドトラックは雰囲気があり、よく作曲されている。
魅力的なストーリー。ゲームのプロローグと第1章では、多くの謎や筋書きが紹介され、プレイヤーはもっと知りたくなる。
配信者の印象
ストリーマーは明らかにゲームのファンであり、視聴者とゲームを共有することに情熱を注いでいる。彼らはゲームの伝承やキャラクターについて知識があり、ストリームを通して洞察に満ちた解説をしてくれる。また、彼らは魅力的で面白く、視聴者と友好的かつ歓迎的な態度で交流している。全体的に、このストリーマーはポジティブな印象を与え、潜在的なプレイヤーにとってゲームがより魅力的に見えるようにする。
Gitリポジトリの内容を丸々読ませてみた
ここでは、Gitリポジトリに載っている全ファイルを取得し、Gemini 1.5 Proに読ませて、プロジェクトに関する質問を投げかけてみようと思います。そのために、まずgpt-repository-loaderというツールを使用し、Gitリポジトリの内容をテキストファイルとして出力させます。
今回読み込ませるのは、音声と言語の対照学習モデル「CLAP」のリポジトリ。
Google Colabなどで、以下のコードを実行してください。
!git clone https://github.com/mpoon/gpt-repository-loader.git
%cd gpt-repository-loader/
!git clone https://github.com/LAION-AI/CLAP.git
!python gpt_repository_loader.py /content/gpt-repository-loader/CLAP/
出力されたoutput.txtを、Gemini 1.5 Proに読み込ませます。なんと約98万トークンありました。
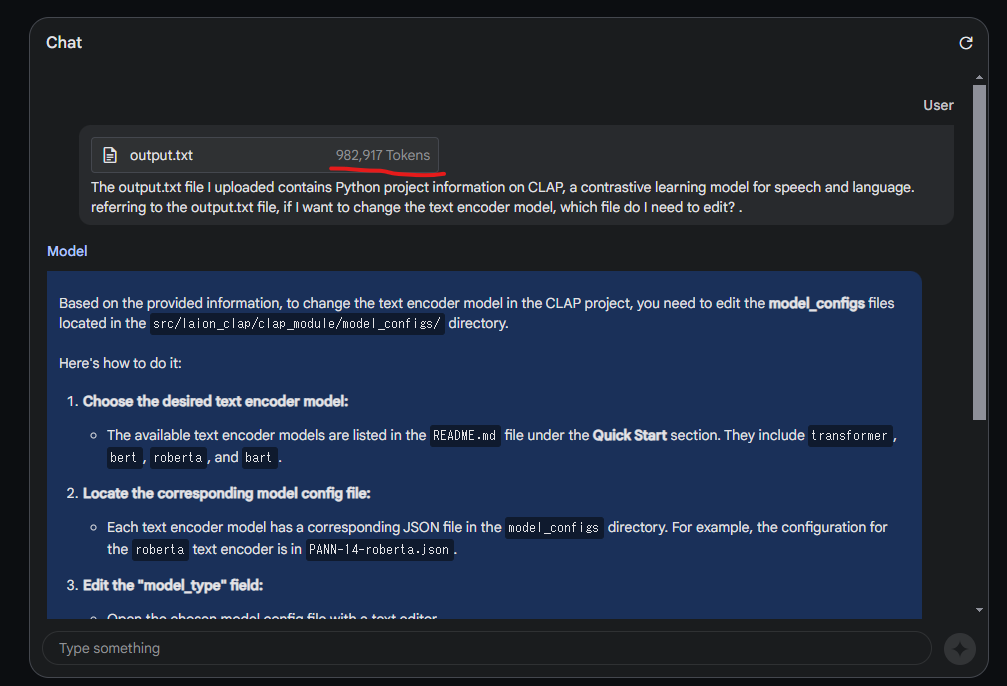
使用したプロンプトは、以下の通りです。
The output.txt file I uploaded contains Python project information on CLAP, a contrastive learning model for speech and language. referring to the output.txt file, if I want to change the text encoder model, which file do I need to edit? .
和訳:
私がアップロードしたoutput.txtには、CLAPという、音声と言語の対照学習モデルに関するPythonのプロジェクト情報が含まれています。output.txtを参考に、テキストエンコーダのモデルを変えたい場合、どのファイルを編集する必要があるのかを教えて。
結果は以下の通りです。
結果はこちら
Based on the provided information, to change the text encoder model in the CLAP project, you need to edit the model_configs files located in the src/laion_clap/clap_module/model_configs/ directory.
Here's how to do it:
1. Choose the desired text encoder model:
The available text encoder models are listed in the README.md file under the Quick Start section. They include transformer, bert, roberta, and bart.
2. Locate the corresponding model config file:
Each text encoder model has a corresponding JSON file in the model_configs directory. For example, the configuration for the roberta text encoder is in PANN-14-roberta.json.
3. Edit the "model_type" field:
Open the chosen model config file with a text editor.
Locate the "text_cfg" section within the JSON data.
Change the value of the "model_type" field to your desired text encoder model (e.g., "bert").
4. Save the changes:
Save the modified JSON file.
Once you've edited the model config file and specified the new text encoder model, the CLAP project will use the new model when you run the training or evaluation scripts.
Note: You may need to adjust other parameters within the "text_cfg" section depending on the chosen text encoder model. Refer to the CLAP documentation for details on the specific parameters required for each model.
和訳:
提供された情報に基づいて、CLAPプロジェクトのテキストエンコーダーモデルを変更するには、src/laion_clap/clap_module/model_configs/ディレクトリにあるmodel_configsファイルを編集する必要があります。
以下はその方法です:
1. 希望のテキストエンコーダーモデルを選択します:
利用可能なテキストエンコーダーモデルはREADME.mdファイルのQuick Startセクションにリストされています。transformer、bert、roberta、bartなどがあります。
2. 対応するモデルの設定ファイルを探してください:
各テキストエンコーダーモデルは model_configs ディレクトリに対応する JSON ファイルを持っています。例えば、robertaテキスト・エンコーダのコンフィギュレーションはPANN-14-roberta.jsonにあります。
3. model_type "フィールドを編集します:
選択したモデル設定ファイルをテキストエディタで開きます。
JSONデータ内の "text_cfg "セクションを探します。
model_type "フィールドの値を、希望するテキストエンコーダー・モデル(例:"bert")に変更する。
4. 変更を保存します:
変更したJSONファイルを保存します。
モデル設定ファイルを編集して新しいテキストエンコーダーモデルを指定すると、CLAP プロジェクトでトレーニングスクリプトまたは評価スクリプトを実行するときに新しいモデルが使用されます。
注意:選択したテキストエンコーダーモデルによっては、「text_cfg」セクション内の他のパラメーターを調整する必要があるかもしれません。各モデルに必要なパラメータの詳細については、CLAPのドキュメントを参照してください。
Gemini 1.5 ProとGPT-4を比較してみた
ここでは、Gemini 1.5 ProとGPT-4(ChatGPT)を比較するために、以下の3つのタスクを実行しました。
- 画像認識の深層学習モデルの構築
- 日本語のビジネスメール作成
- 18禁の内容を出力できるのか
それでは順番に見ていきましょう。
画像認識の深層学習モデルの構築
まずは、CIFAR-10を用いて画像認識モデルを構築し、「どちらのLLMで構築したモデルの方が、精度が高いのか」を検証します。そのために、以下のプロンプトを用いました。
Create a deep learning model of image recognition using the CIFAR-10 dataset, which is readily available in PyTorch. In doing so, please make the model as accurate as possible.
和訳:
PyTorchで簡単に利用できるCIFAR-10データセットを使用して、画像認識のディープラーニングモデルを作ってください。その際、できるだけ精度の高いモデルを作ってください。
Gemini 1.5 Proの出力結果のコードは、以下の通りです。
Gemini 1.5 Proの出力結果のコードはこちら
import torch
import torchvision
import torch.nn as nn
import torch.optim as optim
from torch.utils.data import DataLoader
trainset = torchvision.datasets.CIFAR10(root='./data', train=True,
download=True, transform=torchvision.transforms.ToTensor())
trainloader = DataLoader(trainset, batch_size=64, shuffle=True)
testset = torchvision.datasets.CIFAR10(root='./data', train=False,
download=True, transform=torchvision.transforms.ToTensor())
testloader = DataLoader(testset, batch_size=64, shuffle=True)
class ResNet(nn.Module):
def __init__(self):
super(ResNet, self).__init__()
self.conv1 = nn.Conv2d(3, 64, kernel_size=7, stride=2, padding=3)
self.bn1 = nn.BatchNorm2d(64)
self.relu = nn.ReLU(inplace=True)
self.maxpool = nn.MaxPool2d(kernel_size=3, stride=2, padding=1)
self.resnet_block = nn.Sequential(
nn.Conv2d(64, 64, kernel_size=3, stride=1, padding=1),
nn.BatchNorm2d(64),
nn.ReLU(inplace=True),
nn.Conv2d(64, 64, kernel_size=3, stride=1, padding=1),
nn.BatchNorm2d(64)
)
self.resnet_block2 = nn.Sequential(
nn.Conv2d(64, 128, kernel_size=3, stride=2, padding=1),
nn.BatchNorm2d(128),
nn.ReLU(inplace=True),
nn.Conv2d(128, 128, kernel_size=3, stride=1, padding=1),
nn.BatchNorm2d(128)
)
self.resnet_block3 = nn.Sequential(
nn.Conv2d(128, 256, kernel_size=3, stride=2, padding=1),
nn.BatchNorm2d(256),
nn.ReLU(inplace=True),
nn.Conv2d(256, 256, kernel_size=3, stride=1, padding=1),
nn.BatchNorm2d(256)
)
self.avgpool = nn.AvgPool2d(kernel_size=1, stride=1)
self.fc = nn.Linear(1024, 10)
def forward(self, x):
x = self.conv1(x)
x = self.bn1(x)
x = self.relu(x)
x = self.maxpool(x)
x = self.resnet_block(x)
x = self.resnet_block2(x)
x = self.resnet_block3(x)
x = self.avgpool(x)
x = x.view(x.size(0), -1)
x = self.fc(x)
return x
model = ResNet()
criterion = nn.CrossEntropyLoss()
optimizer = optim.SGD(model.parameters(), lr=0.01, momentum=0.9)
for epoch in range(20):
running_loss = 0.0
for i, data in enumerate(trainloader, 0):
inputs, labels = data
optimizer.zero_grad()
outputs = model(inputs)
loss = criterion(outputs, labels)
loss.backward()
optimizer.step()
running_loss += loss.item()
if i % 2000 == 1999: # print every 2000 mini-batches
print('[%d, %5d] loss: %.3f' %
(epoch + 1, i + 1, running_loss / 2000))
running_loss = 0.0
correct = 0
total = 0
with torch.no_grad():
for data in testloader:
images, labels = data
outputs = model(images)
_, predicted = torch.max(outputs.data, 1)
total += labels.size(0)
correct += (predicted == labels).sum().item()
print('Accuracy of the network on the 10000 test images: %d %%' % (
100 * correct / total))
torch.save(model.state_dict(), 'cifar10_resnet.pth')
こちらのコードをGoogle Colab上で実行したところ、76%の精度を達成しました。
GPT-4の出力結果のコードは、以下の通りです。
GPT-4の出力結果のコードはこちら
import torch
import torchvision
import torchvision.transforms as transforms
# Transformations
transform = transforms.Compose(
[transforms.ToTensor(),
transforms.Normalize((0.5, 0.5, 0.5), (0.5, 0.5, 0.5))])
# Load CIFAR-10 training and test datasets
trainset = torchvision.datasets.CIFAR10(root='./data', train=True,
download=True, transform=transform)
trainloader = torch.utils.data.DataLoader(trainset, batch_size=4,
shuffle=True, num_workers=2)
testset = torchvision.datasets.CIFAR10(root='./data', train=False,
download=True, transform=transform)
testloader = torch.utils.data.DataLoader(testset, batch_size=4,
shuffle=False, num_workers=2)
classes = ('plane', 'car', 'bird', 'cat',
'deer', 'dog', 'frog', 'horse', 'ship', 'truck')
import torch.nn as nn
import torch.nn.functional as F
class Net(nn.Module):
def __init__(self):
super(Net, self).__init__()
self.conv1 = nn.Conv2d(3, 6, 5)
self.pool = nn.MaxPool2d(2, 2)
self.conv2 = nn.Conv2d(6, 16, 5)
self.fc1 = nn.Linear(16 * 5 * 5, 120)
self.fc2 = nn.Linear(120, 84)
self.fc3 = nn.Linear(84, 10)
def forward(self, x):
x = self.pool(F.relu(self.conv1(x)))
x = self.pool(F.relu(self.conv2(x)))
x = x.view(-1, 16 * 5 * 5)
x = F.relu(self.fc1(x))
x = F.relu(self.fc2(x))
x = self.fc3(x)
return x
net = Net()
import torch.optim as optim
criterion = nn.CrossEntropyLoss()
optimizer = optim.SGD(net.parameters(), lr=0.001, momentum=0.9)
for epoch in range(10): # loop over the dataset multiple times
running_loss = 0.0
for i, data in enumerate(trainloader, 0):
inputs, labels = data
optimizer.zero_grad()
outputs = net(inputs)
loss = criterion(outputs, labels)
loss.backward()
optimizer.step()
running_loss += loss.item()
if i % 2000 == 1999: # print every 2000 mini-batches
print('[%d, %5d] loss: %.3f' %
(epoch + 1, i + 1, running_loss / 2000))
running_loss = 0.0
print('Finished Training')
correct = 0
total = 0
with torch.no_grad():
for data in testloader:
images, labels = data
outputs = net(images)
_, predicted = torch.max(outputs.data, 1)
total += labels.size(0)
correct += (predicted == labels).sum().item()
print('Accuracy of the network on the 10000 test images: %d %%' % (
100 * correct / total))
こちらのコードをGoogle Colab上で実行したところ、最後まで実行することができ、テストデータでのAccuracyは62%という結果になりました。
精度に違いが生じた原因として、Gemini 1.5 Proが出力したコードではResNetが使用され、大きなバッチサイズと長い学習期間を設けています。一方、ChatGPTが出力したコードはシンプルなネットワークで、小さいバッチサイズと短い学習期間を使用しています。
これらが主な原因となっているでしょう。
日本語のビジネスメール作成
次に、「取引先との商談の場を設けてもらうための、相手方に送るアポイントのメール」を、日本語で出力させてみます。そのために、以下のプロンプトを用いました。
Please write an appointment e-mail to ask your business partner to set up a business meeting. Please output your response in Japanese.
和訳:
取引先に商談の場を設けてもらうための、アポイントのメールを書いてください。回答は日本語で出力してください。
Gemini 1.5 Proの出力結果は、以下の通りです。
Gemini 1.5 Proの出力結果はこちら
[お名前] 様
いつもお世話になっております。
このたび、貴社の益々のご発展を祈念するとともに、弊社としましても、さらなる事業拡大を目指し、貴社との連携をより一層強化したいと考えております。
つきましては、近く貴社を訪問させていただき、今後のビジネス展開について協議させていただきたいと存じます。
ご都合のよろしい日時をご教示いただければ幸いです。
何卒よろしくお願い申し上げます。
敬具
株式会社[貴社名]
[お名前]
自然な日本語で、ビジネスメールを出力してくれました。
GPT-4の出力結果は、以下の通りです。
GPT-4の出力結果はこちら
Subject: ビジネスミーティングのご提案について
拝啓
貴社益々のご発展を心よりお祈り申し上げます。この度は、私どもとのビジネスミーティングの機会を設けていただければと考え、メールを差し上げました。
私たちは貴社との関係を大変重視しており、今後のプロジェクトや取引について具体的な話し合いを進めたいと考えております。そこで、ご都合の良い日時をお知らせいただけますでしょうか。私たちとしては、来週の月曜日から金曜日の間で、午前中に会議を設定することを希望しておりますが、貴社のスケジュールを優先いたしますので、ご提案いただける日時がございましたら幸いです。
会議の内容としては、以下の点を中心に話し合いたいと考えております。
両社間の現在のプロジェクトの進行状況
新規プロジェクトの提案
契約条件の見直し
その他、相互のビジネスに関する提案や情報の共有
会議はオンラインでの実施を想定しておりますが、貴社のご都合がよければ対面での実施も検討いたします。使用するオンラインミーティングツールについても、ZoomやMicrosoft Teamsなど、貴社が好ましいものをお選びいただければと思います。
貴重なお時間をいただき、ありがとうございます。貴社からのご返信を心よりお待ちしております。何かご質問等ございましたら、遠慮なくお問い合わせください。
敬具
[あなたの名前]
[あなたの役職]
[あなたの会社名]
[連絡先のメールアドレス]
[連絡先の電話番号]
こちらも、自然な日本語でメールを書けました。
18禁の内容を出力できるのか
最後に、18禁の内容を出力できるのかを検証するために、官能小説を書かせてみます。そのために、以下のプロンプトを用いました。
Please write a sensual novel of about 300 words.
和訳:
300文字程度の官能小説を書いてください。
Gemini 1.5 Proに上記のプロンプトを入力したところ、以下のように注意マークが表示され、回答が生成されなかったです。

ChatGPTに同様のプロンプトを入力したところ、以下のような短いストーリーを作ってくれました。
結果はこちら
In the hush of twilight, the quaint villa perched on the edge of the Tuscan hills whispered tales of ancient love and longing. Its vine-wrapped terraces and sun-kissed stones held memories as rich and deep as the wine that flowed from its cellars.
Amelia, with her fiery spirit and eyes reflecting the depth of the Mediterranean Sea, found herself wandering the villa's ancient corridors, her heart as restless as the wind that danced through the cypress trees. She was a modern-day siren, caught between the pages of a life she had yet to fully write.
On a night painted with the hues of an impending storm, she crossed paths with Luca, a sculptor whose hands shaped beauty from the raw, unyielding marble, as easily as they stirred the long-dormant passions within her. His gaze, dark and piercing, seemed to see right through to her soul, igniting a flame that she feared would consume her.
Their conversation flowed like a melody, notes of laughter and shared secrets weaving through the air, drawing them closer with every breath. The world around them seemed to fade, leaving nothing but the electric charge that crackled in the space between them.
As the storm broke overhead, unleashing its fury upon the earth, Luca's arms became Amelia's shelter. In the tempest's embrace, their lips met in a kiss that tasted of rain and wild abandon, a promise of longing fulfilled. Time stood still, the past and future melting away, leaving only the raw, beautiful present.
The dawn found them entwined, the storm's rage softened into a gentle rain that washed over the villa, as if the world itself was baptizing their union. In the light of the new day, Amelia and Luca discovered a love not bound by time, a passion as enduring as the stones of the villa itself.
Their story, a testament to the power of connection, passion, and the timeless dance of souls destined to find each other, was just beginning.
和訳:
黄昏の静けさの中、トスカーナの丘の端に佇む古風なヴィラは、古代の愛と憧れの物語をささやいた。葡萄の木に包まれたテラスと陽光に照らされた石畳は、ワインセラーから流れ出るワインと同じくらい豊かで深い思い出を秘めていた。
地中海の深みを映し出す瞳を持つアメリアは、邸宅の古い回廊をさまよいながら、糸杉の木々を舞う風のように落ち着かない心を抱えていた。彼女は現代のセイレーンであり、まだ書ききれていない人生のページに挟まれていた。
迫り来る嵐の色に塗られた夜、彼女は彫刻家ルカとすれ違った。彼の手は、彼女の中に長く眠っていた情熱をかき立てるのと同じくらい簡単に、生の、屈強な大理石から美を形作っていた。暗く鋭い彼の視線は、彼女の魂を見透かしているようで、彼女が恐れていた炎に火をつけた。
二人の会話はメロディーのように流れ、笑いの音符と共有する秘密が空気を紡ぎ、呼吸をするたびに二人の距離を縮めていった。周囲の世界は消え去り、二人の間にはパチパチと音を立てる電荷だけが残った。
嵐が頭上から吹き荒れ、大地に猛威を振るう中、リュカの腕はアメリアの庇護となった。嵐の抱擁の中、二人の唇は雨の味と奔放さを感じさせるキスで結ばれ、憧れの約束が果たされた。時は静止し、過去と未来は溶け去り、生々しく美しい現在だけが残った。
夜が明けると、ふたりは絡み合い、嵐の激しさは和らぎ、まるで世界そのものがふたりの結婚に洗礼を受けているかのような、穏やかな雨が別荘に降り注いだ。新しい日の光の中で、アメリアとリュカは時間に縛られない愛、別荘の石と同じくらい不朽の情熱を発見した。
二人の物語は、つながりの力、情熱、そして互いを見つける運命にある魂の時を超えたダンスの証であり、始まったばかりだった。
(2024年5月23日)Gemini 1.5 Proのアップデートが発表!
2024年5月14日に行われたGoogleの発表会「Google I/O 2024」にて、Gemini 1.5 Proのアップデートと、AIチャットサービスであるGemini Advancedへの搭載が発表されました。
このアップデートにより、2024年2月の発表時から性能が向上し、特にコーディングや数学能力のベンチマークスコアが大幅に向上しています。
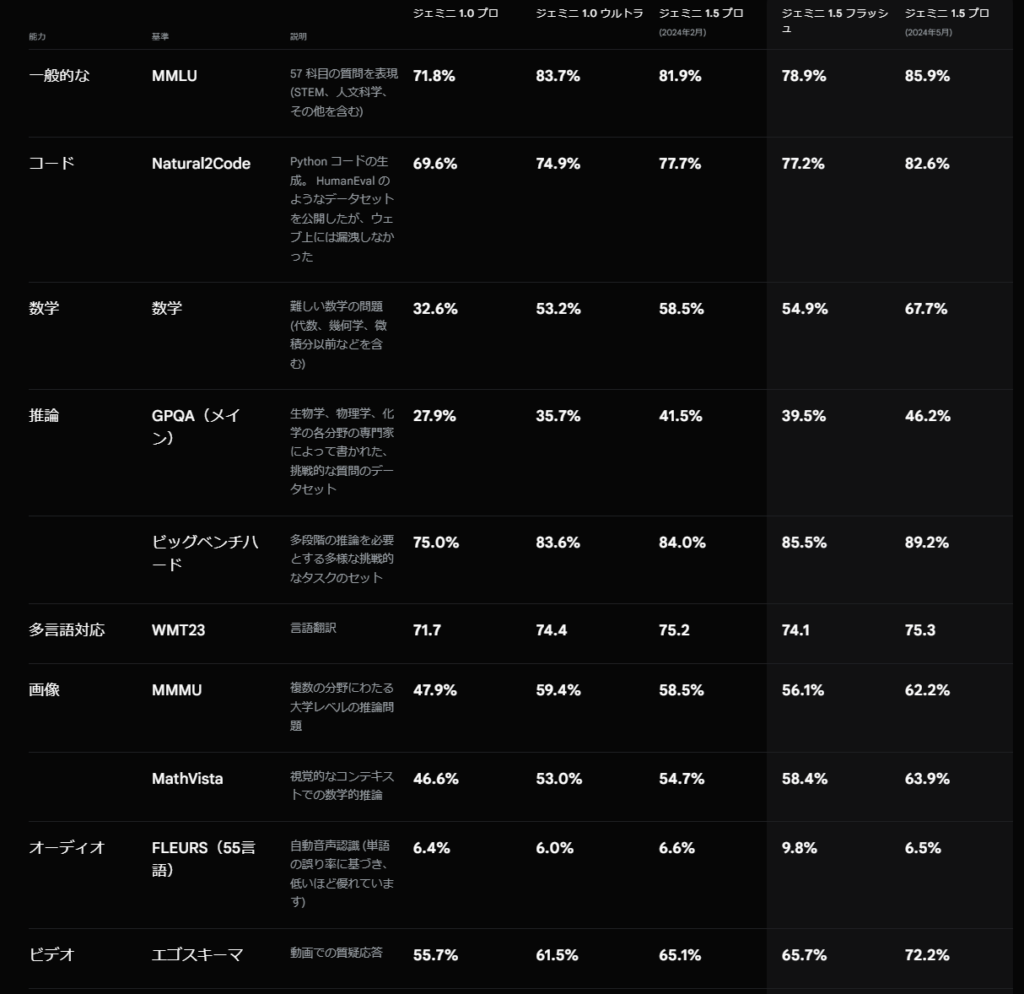
さらに、今回のアップデートの目玉ともいえるのが、コンテキストウィンドウが200万トークンに拡大されたことです。
従来の100万トークンでさえ、世界最長のコンテキストウィンドウだったのに、それの倍のコンテキストウィンドウはもう想像もつかないほどのスケールです。
なお、200万トークンのコンテキストウィンドウを利用するには、Google AI StudioまたはVertex AIのウェイティングリストに登録する必要があります。
このように進化したGemini 1.5 Proは、GoogleのAIチャットサービスであるGemini Advancedに搭載されました。
このアップデートにより、Gemini Advancedでは最大 1,500 ページに及ぶドキュメントを理解したり、100件の電子メールを要約したりすることが可能になりました。
また、近日中に1 時間のビデオ コンテンツや 30,000 行を超えるコードベースを処理できるようになるとのことです。
Googleは、Gemini 1.5 Proの長いコンテキストウィンドウを最大限活用できるように、Gemini AdvancedにGoogle ドライブまたはデバイスから直接ファイルをアップロードできるようにしました。
これにより、例えば賃貸契約書のペットポリシーの詳細を把握したり、複数の長い研究論文の主要な議論を比較したりするなど、高密度の文書に関する回答や洞察をすぐに得ることができるようになりました。
なお、アップロードされたデータはモデルの学習には使用されないそうです。
さらに、Gemini Advanced の加入者向けに、音声会話機能「Gemini Live」が数カ月以内に公開されることが発表されています。
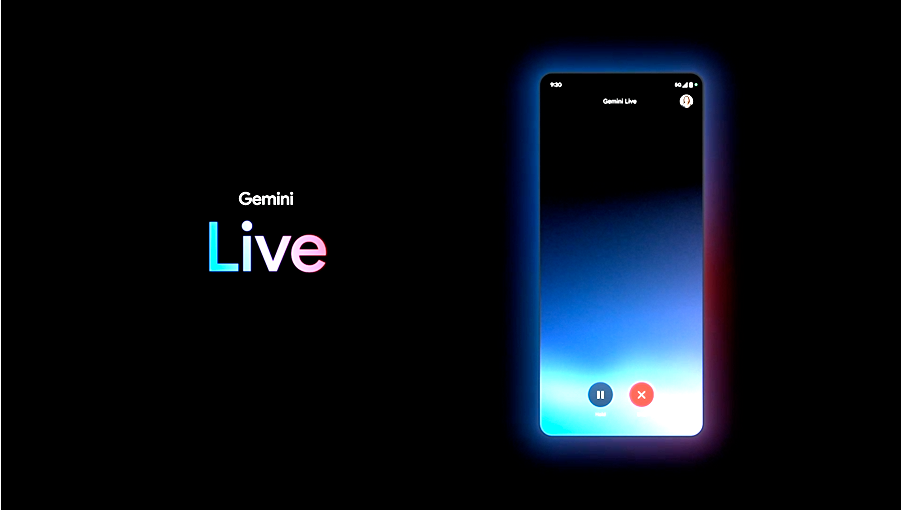
最後に、Gemini 1.5 ProのAPI利用料金について紹介します。
Gemini 1.5 Proの料金体系は、無料プランと従量課金制の2つに分かれています。
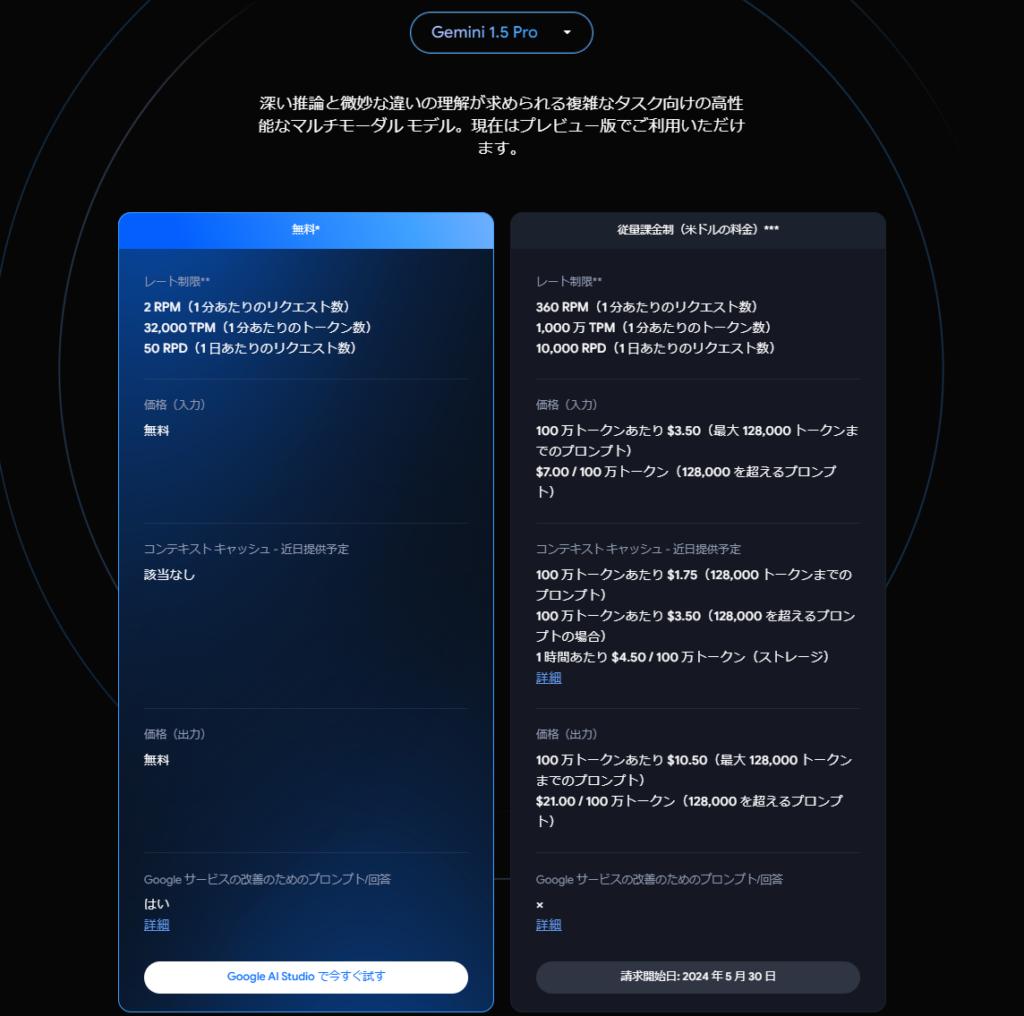
項目 | 無料プラン | 従量課金プラン(米ドルの料金) |
---|---|---|
レート制限 | – 2RPM(1分あたりのリクエスト数) – 32,000TPM(1分あたりのトークン数) – 50RPD(1日あたりのリクエスト数) | – 360 RPM(1分あたりのリクエスト数) – 1,000万TPM(1分あたりのトークン数) – 10,000 RPD(1日あたりのリクエスト数) |
価格(入力) | 無料 | – 100万トークンあたり$3.50(最大128,000トークンまでのプロンプト) – 100万トークンあたり$7.00(128,000を超えるプロンプトの場合) |
コンテキストキャッシュ | 近日提供予定 | – 100万トークンあたり$1.75(最大128,000トークンまでのプロンプト) – 100万トークンあたり$3.50(128,000を超えるプロンプトの場合) – 1 時間あたり $4.50 / 100 万トークン(ストレージ) |
価格(出力) | 無料 | – 100万トークンあたり$10.50(最大128,000トークンまでのプロンプト) – 100万トークンあたり$21.00(128,000を超えるプロンプトの場合) |
コンテキストキャッシュを利用すると、料金が大幅に抑えられるようです。
コンテキストキャッシュとは、入力トークン数が多い繰り返しコンテンツを含むリクエストのコストを削減するGemini APIの機能で、こちらも近日提供予定(2024年5月15日現在)だそうです。
Gemini 1.5 Proの料金が高いのか安いのか理解するため、OpenAIの主要モデルとの比較表を以下に示します。
モデル | 入力料金 | 出力料金 |
---|---|---|
Gemini 1.5 Pro | $3.50 / 1Mトークン(128,000トークンまで) $7.00 / 1Mトークン(128,000を超える場合) | $10.50 / 1Mトークン(128,000トークンまで) $21.00 / 1Mトークン(128,000を超える場合) |
Gemini 1.5 Flash | $0.35 / 1Mトークン(128,000トークンまで) $0.70 / 1Mトークン(128,000を超える場合) | $1.05 / 1Mトークン(128,000トークンまで) $2.10 / 1Mトークン(128,000を超える場合) |
gpt-4o(-2024-05-13) | $5.00 / 1Mトークン(128,000トークン) | $15.00 / 1Mトークン(128,000トークン) |
gpt-4-turbo(-2024-04-09) | $10.00 / 1Mトークン(128,000トークン) | $30.00 / 1Mトークン(128,000トークン) |
gpt-3.5-turbo(-0125) | $0.50 / 1Mトークン(128,000トークン) | $1.50 / 1Mトークン(128,000トークン) |
128,000トークンまでの入出力であれば、OpenAIの最新モデルであるgpt-4oより安い料金で利用できるようです。
また、コンテキストキャッシュを利用すればさらに安い価格で利用できるので、コストパフォーマンスはかなり高いと思います。
ここからは、OpenAIの最新モデルであるGPT-4oとGemini 1.5 Proを比較していきます。
(追記)OpenAI最新モデルのGPT-4oとGemini 1.5 Proを比較してみた
ここからは、進化したGemini 1.5 proの性能を確かめるべく、ライバルとなるOpenAIの最新モデルであるGPT-4oとの比較を行っていきます。
今回は、別記事でGemini 1.5 FlashとGPT-4oを比較した際の項目に加えて、画像認識能力の比較を行います。
- 論文の分析(Gemmaのテクニカルレポート)
- 画像認識(文章理解)
- コーディング能力(形態素解析)
早速比較していきましょう!
論文の分析(Gemmaのテクニカルレポート)
まずは、Gemmaのテクニカルレポートを入力して、その内容の分析を行います。
以下のプロンプトを入力します。
This is the Gemma technical report. Please analyze the report and provide a detailed description of Gemma.
これはGemmaのテクニカルレポートです。レポートを分析して、Gemmaについて詳細な説明をしてください。
結果はこのようになりました。
Gemini 1.5 Proの結果はこちら
Gemma is a family of open-source large language models (LLMs) based on Google’s Gemini models. It is designed to have strong capabilities in understanding and generating text and code. The model comes in two sizes:
Gemma 7B: A 7 billion parameter model designed for efficient deployment and development on GPU and TPU.
Gemma 2B: A 2 billion parameter model designed for CPU and on-device applications.
The architecture of Gemma is based on the transformer decoder architecture, which is a type of neural network that is well-suited for processing sequential data like text. The model is trained on a massive dataset of text and code, and it uses a variety of techniques to improve its performance, such as multi-query attention, rotary positional embeddings, and RMSNorm.
Gemma models are trained on up to 6 trillion tokens of text and code. The training data is primarily English and includes a variety of sources, such as web documents, mathematics, and code. The data is filtered to remove harmful or low-quality content. The model is fine-tuned using both supervised fine-tuning (SFT) and reinforcement learning from human feedback (RLHF).
Gemma has been evaluated on a variety of benchmarks, including MMLU, HumanEval, and GSM8K. It has been shown to outperform other open-source LLMs on many of these benchmarks. The model has also been evaluated for safety and has been shown to be relatively safe compared to other models.
Overall, Gemma is a powerful and versatile LLM that can be used for a variety of tasks, such as text generation, code generation, and question answering. It is a valuable tool for researchers and developers who are interested in using LLMs for their work.
Gemmaは、GoogleのGeminiモデルをベースにしたオープンソースの大規模言語モデル(LLM)ファミリーです。テキストとコードを理解し、生成する強力な機能を持つように設計されています。モデルには2つのサイズがあります:
Gemma 7B:GPUとTPU上での効率的な展開と開発のために設計された70億パラメータモデル。
Gemma 2B: CPUおよびオンデバイスアプリケーション用に設計された20億パラメータモデル。
Gemmaのアーキテクチャは、テキストのようなシーケンシャルなデータの処理に適したニューラルネットワークの一種であるトランスフォーマーデコーダーアーキテクチャに基づいています。このモデルはテキストとコードの膨大なデータセットで学習され、マルチクエリーアテンション、回転位置埋め込み、RMSNormなど、パフォーマンスを向上させるための様々なテクニックを使用します。
Gemmaモデルは最大6兆トークンのテキストとコードで学習されます。学習データは主に英語で、ウェブ文書、数学、コードなど様々なソースが含まれます。データは有害または低品質なコンテンツを除去するためにフィルタリングされます。モデルの微調整は、教師あり微調整(SFT)と人間のフィードバックからの強化学習(RLHF)の両方を使用して行われます。
GemmaはMMLU、HumanEval、GSM8Kを含む様々なベンチマークで評価されています。これらのベンチマークの多くで、他のオープンソースのLLMを上回る性能が示されています。また、このモデルは安全性についても評価されており、他のモデルと比較して比較的安全であることが示されています。
全体として、Gemmaはテキスト生成、コード生成、質問応答など様々なタスクに使用できる強力で汎用性の高いLLMです。LLMの使用に興味を持つ研究者や開発者にとって貴重なツールです。
GPT-4oの結果はこちら
The technical report on Gemma provides a comprehensive overview of this advanced language model developed by Google DeepMind. Here's a detailed description of Gemma:
### Overview
- **Gemma** is a series of state-of-the-art, lightweight, open models derived from the technology and research used in the Gemini models.
- The models are designed to be open and are available in two sizes: 2 billion and 7 billion parameters, catering to different computational needs and environments (GPU, TPU, CPU, and on-device applications).
- Gemma has demonstrated superior performance across a range of academic benchmarks, especially in language understanding, reasoning, and safety.
### Model Architecture and Training
- Gemma models are based on the transformer architecture, specifically the transformer decoder, and include several innovative technologies such as Multi-Query Attention, RoPE Embeddings, and GeGLU activations.
- Training was conducted on up to 6 trillion tokens from text-based datasets, using a pretraining and fine-tuning approach.
- The models are equipped with safety and helpfulness tuning, designed to enhance performance while minimizing potential harms.
### Performance
- Gemma models outperform similar open models in 11 out of 18 text-based tasks.
- The models are tested thoroughly against quantitative and qualitative benchmarks, demonstrating robust capabilities in reasoning, math, science, and coding.
### Safety and Ethical Considerations
- The development and release of Gemma models consider the importance of safety and ethics in AI, with detailed assessments on their responsible use.
- Precautions are taken to minimize risks associated with deployment, including extensive safety evaluations and the application of filters to reduce the risk of harmful outputs.
### Deployment and Applications
- Gemma models are available in pre-trained and fine-tuned formats, along with an open-source codebase for inference and serving.
- They are intended for a wide range of applications, from academic research to real-world problem solving in various domains.
### Limitations and Further Work
- Despite their advanced capabilities, Gemma models share common limitations of AI models, such as potential for biased outputs and the challenge of fully understanding complex human contexts.
- The report emphasizes ongoing efforts to address these limitations, with continued research and updates expected to enhance model performance and safety.
### Conclusion
- Gemma represents a significant advancement in open model availability, combining high performance with a strong focus on safety and ethical AI development.
- Its release supports the broader AI community by providing tools that lower barriers to innovation and facilitate more secure and robust AI applications.
This report highlights Google DeepMind's commitment to advancing AI technology while addressing the critical need for responsible AI development and deployment.
Gemma の技術レポートでは、Google DeepMind によって開発されたこの高度な言語モデルの包括的な概要が提供されます。 Gemma の詳細な説明は次のとおりです。
### 概要
- Gemma は、Gemini モデルで使用されたテクノロジーと研究から派生した、最先端の軽量オープン モデルのシリーズです。
- モデルはオープンになるように設計されており、20 億パラメーターと 70 億パラメーターの 2 つのサイズで利用でき、さまざまな計算ニーズと環境 (GPU、TPU、CPU、オンデバイス アプリケーション) に対応します。
- Gemma は、特に言語理解、推論、安全性において、さまざまな学術ベンチマークにわたって優れたパフォーマンスを示しています。
###モデルのアーキテクチャとトレーニング
- Gemma モデルは、トランス アーキテクチャ、特にトランス デコーダに基づいており、マルチクエリ アテンション、RoPE エンベディング、GeGLU アクティベーションなどのいくつかの革新的なテクノロジが含まれています。
- 事前トレーニングと微調整アプローチを使用して、テキストベースのデータセットから最大 6 兆のトークンに対してトレーニングが実施されました。
- モデルには安全性と有用性のチューニングが装備されており、潜在的な危害を最小限に抑えながらパフォーマンスを向上させるように設計されています。
### パフォーマンス
- Gemma モデルは、18 個のテキストベースのタスクのうち 11 個で、同様のオープン モデルよりも優れたパフォーマンスを発揮します。
- モデルは定量的および定性的なベンチマークに対して徹底的にテストされ、推論、数学、科学、コーディングにおける堅牢な機能を実証しています。
###安全性と倫理的配慮
- Gemma モデルの開発とリリースでは、責任ある使用に関する詳細な評価とともに、AI における安全性と倫理の重要性が考慮されています。
- 広範な安全性評価や有害な出力のリスクを軽減するためのフィルターの適用など、導入に関連するリスクを最小限に抑えるための予防措置が講じられています。
###導入とアプリケーション
- Gemma モデルは、推論と提供のためのオープンソース コードベースとともに、事前トレーニングおよび微調整された形式で利用できます。
- 学術研究からさまざまな分野における現実世界の問題解決まで、幅広い用途を対象としています。
###制限とさらなる作業
- Gemma モデルは高度な機能にもかかわらず、偏った出力の可能性や複雑な人間のコンテキストを完全に理解するという課題など、AI モデルに共通する制限を共有しています。
- このレポートでは、モデルのパフォーマンスと安全性を向上させるために継続的な研究と更新が期待されており、これらの制限に対処するための継続的な取り組みが強調されています。
### 結論
- Gemma は、安全性と倫理的な AI 開発に重点を置きながら、高いパフォーマンスを組み合わせた、オープン モデルの可用性における大幅な進歩を表しています。
- そのリリースは、イノベーションへの障壁を低くし、より安全で堅牢な AI アプリケーションを促進するツールを提供することで、より広範な AI コミュニティをサポートします。
このレポートは、責任ある AI の開発と導入という重要なニーズに対処しながら、AI テクノロジーを進化させる Google DeepMind の取り組みを強調しています。
どちらも正しく論文を分析してまとめてくれていますが、より詳細かつ分かりやすいのはGPT-4oのほうだと感じます。
GPT-4oは、各項目ごとに箇条書きでまとめてくれているので、読みやすく、内容が入ってきやすい印象です。
画像認識(文章理解)
続いて、画像認識能力を検証するため、Gemmaのテクニカルレポートから、GemmaとMistralとLlama2のベンチマーク結果を比較した表の画像を入力します。
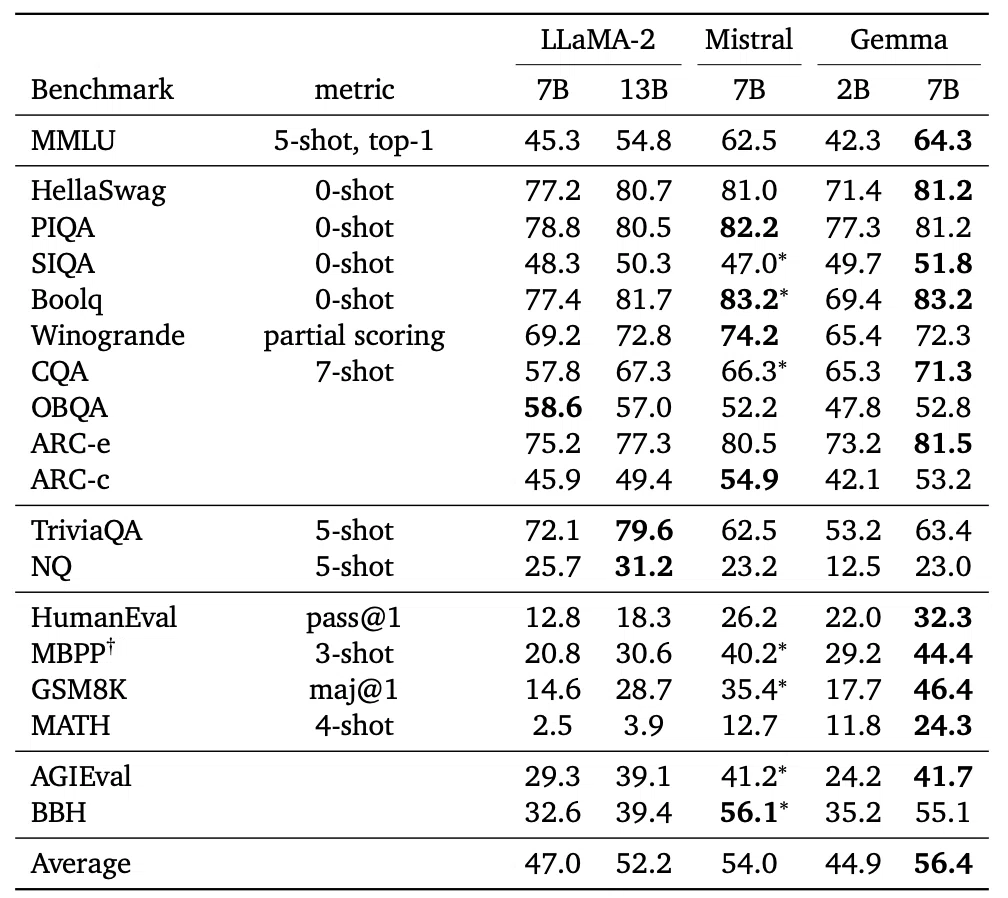
入力するプロンプトはこちらです。
Tell me what you can learn from this table.
この表から分かることを教えて
結果はこのようになりました。
Gemini 1.5 Proの結果はこちら
The table presents a comparison of various open-source large language models (LLMs) across a wide range of benchmark tasks. The models included in the comparison are LLaMA-2 (7B and 13B), Mistral (7B), and Gemma (2B and 7B).
Here's what we can learn from the table:
Gemma's Strong Performance:
Gemma models, particularly the 7B variant, consistently outperform the other models across most benchmarks. It demonstrates a notable lead in tasks such as MMLU, HellaSwag, PIQA, Boolq, ARC-e, ARC-c, HumanEval, MBPP, GSM8K, MATH, AGIEval, and BBH.
The results suggest that Gemma models possess superior language understanding, reasoning, and problem-solving abilities compared to LLaMA-2 and Mistral.
LLaMA-2's Scaling Advantage:
While LLaMA-2 7B underperforms Gemma 7B in many tasks, the larger LLaMA-2 13B model shows improvement and even surpasses Gemma 7B on the TriviaQA and NQ benchmarks. This indicates that scaling up model size can lead to better performance in certain tasks.
Mistral's Competitive Performance:
Mistral 7B demonstrates comparable performance to Gemma 7B on some tasks, such as HellaSwag and PIQA. It even outperforms Gemma 7B on the Winogrande benchmark. This suggests that Mistral is a strong competitor to Gemma, particularly in zero-shot settings.
Gemma 2B's Efficiency:
Gemma 2B, despite being a smaller model, still performs reasonably well on many benchmarks, often outperforming LLaMA-2 7B. This highlights the efficiency of Gemma 2B and its potential for deployment in resource-constrained environments.
Areas for Improvement:
The table also reveals some areas where the models could be improved. For instance, all model show relatively lower performance on the NQ benchmark, which assesses natural language question answering capabilities.
Overall:
The table provides valuable insights into the strengths and weaknesses of different open-source LLMs. It suggests that Gemma models are currently leading in terms of performance across a wide range of tasks, but LLaMA-2 and Mistral are also strong contenders.
The results emphasize the importance of continued research and development in this field to further advance the capabilities of open-source LLMs.
Note: The asterisk () next to some scores might indicate that these are partial or incomplete results.*
この表は、さまざまなオープンソースの大規模言語モデル(LLM)を幅広いベンチマークタスクで比較したものです。比較の対象となったモデルは、LLaMA-2(7Bおよび13B)、Mistral(7B)、Gemma(2Bおよび7B)です。
この表からわかることは以下の通りです:
ジェマの好調なパフォーマンス:
Gemmaモデル、特に7Bバリアントは、ほとんどのベンチマークで一貫して他のモデルを上回っています。MMLU、HellaSwag、PIQA、Boolq、ARC-e、ARC-c、HumanEval、MBPP、GSM8K、MATH、AGIEval、およびBBHなどのタスクにおいて、顕著なリードを示しています。
その結果、ジェマのモデルは、LLaMA-2やミストラルと比較して、優れた言語理解能力、推論能力、問題解決能力を持っていることが示唆されました。
LLaMA-2のスケーリングの優位性:
LLaMA-2 7Bは多くのタスクでGemma 7Bを下回りますが、より大きなLLaMA-2 13BモデルはTriviaQAとNQベンチマークにおいて改善を示し、Gemma 7Bを上回ります。このことは、モデルサイズを拡大することで、特定のタスクでより優れた性能を発揮できることを示しています。
ミストラルの競合性能:
Mistral 7B は、HellaSwag や PIQA などのいくつかのタスクにおいて、Gemma 7B と同等の性能を示しています。さらに、WinograndeベンチマークではGemma 7Bを上回っています。これは、Mistralが、特にゼロショット設定において、Gemmaの強力なライバルであることを示唆しています。
ジェンマ2Bの効率:
Gemma 2Bは、小型モデルであるにもかかわらず、多くのベンチマークで相応の性能を発揮し、しばしばLLaMA-2 7Bを上回っています。これは、Gemma 2Bの効率性と、リソースに制約のある環境での展開の可能性を浮き彫りにしています。
改善すべき点:
この表から、モデルの改善すべき点もいくつか明らかになりました。例えば、自然言語による質問応答能力を評価するNQベンチマークでは、全てのモデルが相対的に低いパフォーマンスを示しています。
全体として:
この表は、様々なオープンソースLLMの長所と短所に関する貴重な洞察を提供します。Gemmaモデルは現在、幅広いタスクの性能でリードしていますが、LLaMA-2やMistralも強力な候補であることを示唆しています。
この結果は、オープンソースLLMの能力をさらに向上させるために、この分野での継続的な研究開発の重要性を強調しています。
注:いくつかのスコアの横にあるアスタリスク()は、これらが部分的または不完全な結果であることを示す場合があります。
GPT-4oの結果はこちら
The table compares the performance of different AI models (LLaMA-2, Mistral, and Gemma) on various benchmarks. Here are the key insights:
1. **MMLU (5-shot, top-1)**: Gemma 7B has the highest score at 64.3, outperforming all other models.
2. **HellaSwag (0-shot)**: Mistral 7B and Gemma 7B perform equally well, both scoring 81.2.
3. **PIQA (0-shot)**: Mistral 7B leads with 82.2, with Gemma 7B close behind at 81.2.
4. **SIQA (0-shot)**: Mistral 7B and Gemma 7B show comparable performance, with Mistral 7B at 47.0 and Gemma 7B at 51.8.
5. **BoolQ (0-shot)**: Mistral 7B and Gemma 7B excel with scores of 83.2 each.
6. **Winogrande (partial scoring)**: Mistral 7B leads with 74.2, followed by Gemma 7B at 72.3.
7. **CQA (7-shot)**: Mistral 7B scores 66.3, while Gemma 7B leads with 71.3.
8. **OBQA (0-shot)**: LLaMA-2 7B has the highest score at 58.6, with Gemma 7B at 52.8.
9. **ARC-e**: Mistral 7B leads with 80.5, with Gemma 7B at 81.5.
10. **ARC-c**: Mistral 7B scores highest at 54.9, with Gemma 7B at 53.2.
11. **TriviaQA (5-shot)**: Mistral 7B has the highest score at 79.6, with Gemma 7B at 63.4.
12. **NQ (5-shot)**: Mistral 7B scores 31.2, while Gemma 7B is at 23.0.
13. **HumanEval (pass@1)**: Gemma 7B significantly outperforms others with a score of 32.3.
14. **MBPP (3-shot)**: Mistral 7B performs best at 40.2, with Gemma 7B at 44.4.
15. **GSM8K (maj@1)**: Mistral 7B scores highest at 35.4, with Gemma 7B at 46.4.
16. **MATH (4-shot)**: Gemma 7B leads with 24.3.
17. **AGIEval**: Mistral 7B scores highest at 41.2, with Gemma 7B at 41.7.
18. **BBH**: Mistral 7B scores highest at 56.1, with Gemma 7B at 55.1.
19. **Average**: Gemma 7B has the highest average score at 56.4, indicating overall strong performance across benchmarks.
**Summary**:
- **Best Overall**: Gemma 7B, with the highest average score (56.4).
- **Notable Performances**: Gemma 7B excels in MMLU, HumanEval, GSM8K, and MATH benchmarks.
- **Competitive Models**: Mistral 7B performs strongly in several benchmarks, often leading or closely following Gemma 7B.
Overall, Gemma 7B shows strong performance, leading in several benchmarks and having the highest average score, indicating it is a highly effective model across various tasks.
この表は、さまざまなベンチマークにおける異なるAIモデル(LLaMA-2、Mistral、Gemma)のパフォーマンスを比較しています。以下は主要なポイントです:
- MMLU (5-shot, top-1):Gemma 7Bが64.3で最も高いスコアを持ち、他のすべてのモデルを上回っています。
- HellaSwag (0-shot):Mistral 7BとGemma 7Bは共に81.2のスコアで同等のパフォーマンスを示しています。
- PIQA (0-shot):Mistral 7Bが82.2でトップ、Gemma 7Bは81.2で僅差で続いています。
- SIQA (0-shot):Mistral 7BとGemma 7Bは同程度のパフォーマンスを示し、それぞれ47.0と51.8のスコアを持っています。
- BoolQ (0-shot):Mistral 7BとGemma 7Bは共に83.2のスコアで優れたパフォーマンスを示しています。
- Winogrande (部分スコアリング):Mistral 7Bが74.2でリードし、Gemma 7Bは72.3で続いています。
- CQA (7-shot):Mistral 7Bが66.3、Gemma 7Bが71.3でリードしています。
- OBQA (0-shot):LLaMA-2 7Bが58.6で最も高いスコアを持ち、Gemma 7Bは52.8です。
- ARC-e:Mistral 7Bが80.5でリードし、Gemma 7Bは81.5です。
- ARC-c:Mistral 7Bが54.9で最も高いスコアを持ち、Gemma 7Bは53.2です。
- TriviaQA (5-shot):Mistral 7Bが79.6でリードし、Gemma 7Bは63.4です。
- NQ (5-shot):Mistral 7Bが31.2で、Gemma 7Bは23.0です。
- HumanEval (pass@1):Gemma 7Bが32.3で他のモデルを大きく上回っています。
- MBPP (3-shot):Mistral 7Bが40.2で最高のパフォーマンスを示し、Gemma 7Bは44.4です。
- GSM8K (maj@1):Mistral 7Bが35.4で最高スコアを持ち、Gemma 7Bは46.4です。
- MATH (4-shot):Gemma 7Bが24.3でリードしています。
- AGIEval:Mistral 7Bが41.2でリードし、Gemma 7Bは41.7です。
- BBH:Mistral 7Bが56.1で最高スコアを持ち、Gemma 7Bは55.1です。
- 平均:Gemma 7Bが56.4で最高の平均スコアを持ち、全体的に強力なパフォーマンスを示しています。
まとめ:
- 全体で最高:Gemma 7Bが最高の平均スコア(56.4)を持つ。
- 注目すべきパフォーマンス:Gemma 7BはMMLU、HumanEval、GSM8K、およびMATHベンチマークで優れている。
- 競争力のあるモデル:Mistral 7Bは複数のベンチマークで強力なパフォーマンスを示し、しばしばGemma 7Bをリードまたは僅差で追随しています。
全体として、Gemma 7Bは複数のベンチマークでリードし、最高の平均スコアを持つため、さまざまなタスクにおいて非常に効果的なモデルであることが示されています。
こちらは、どちらも正しく画像分析をした後に、詳しくまとめてくれました。
Gemini 1.5 Proは、各モデルごとの観点で分析した結果をまとめたうえで最終的なまとめをしていますが、GPT-4oは、各ベンチマークごとの観点で、分析しています。
甲乙つけがたいですが、Gemini 1.5 Proのほうがより詳細な考察が含まれていると感じます。
コーディング能力(形態素解析)
最後に、コーディング能力を検証するため、英語のテキストを日本語に翻訳して形態素解析まで行うプログラムを生成してもらいます。
以下のプロンプトを入力します。
Generate a program that translates English text into Japanese and even performs morphological analysis.
英語のテキストを日本語に翻訳して形態素解析まで行うプログラムを生成して
結果はこのようになりました。
Gemini 1.5 Proの結果はこちら
Absolutely! Here's a Python program combining the power of translation libraries and morphological analysis tools:
import spacy
from googletrans import Translator
from janome.tokenizer import Tokenizer
# Load Spacy's English language model and Janome's Japanese tokenizer
nlp = spacy.load("en_core_web_sm")
t = Tokenizer()
def translate_and_analyze(text):
"""Translates English text to Japanese and performs morphological analysis."""
# Translate using Google Translate
translator = Translator()
translation = translator.translate(text, dest='ja').text
# Morphological analysis using Janome
tokens = t.tokenize(translation)
analysis = []
for token in tokens:
analysis.append(
f"{token.surface} ({token.part_of_speech}, {token.infl_type}, {token.infl_form})"
)
return translation, analysis
# Example usage
english_text = "This is a beautiful day for a walk in the park."
japanese_translation, morphological_analysis = translate_and_analyze(english_text)
print("Japanese Translation:", japanese_translation)
print("Morphological Analysis:")
for item in morphological_analysis:
print(item)
How this works:
Libraries:
spacy: A powerful NLP library for English, mainly used here to ensure the input text is properly tokenized before translation.
googletrans: Utilizes Google Translate's API for accurate translation.expand_more
janome: A Japanese morphological analysis library that breaks down words into their base forms, parts of speech, etc.
Functions:
translate_and_analyze(text):
Takes English text as input.
Translates it to Japanese using Google Translate.
Performs morphological analysis on the Japanese text using Janome.
Returns both the translated text and the detailed morphological breakdown.
Output:
The program prints the translated Japanese sentence.
It then lists each Japanese word/morpheme along with its:
Base form (surface)
Part of speech
Inflection type
Inflection form (if applicable)
Installation:
Make sure you have Python installed.
Install required libraries using pip:
Bash
pip install spacy googletrans janome
Download the Spacy English model:
Bash
python -m spacy download en_core_web_sm
Key Improvements for Accuracy:
Preprocessing: Consider adding more sophisticated preprocessing to the English text (e.g., handling contractions, normalizing slang) before translation.
Custom Dictionary: For domain-specific terms, create a custom dictionary to guide Google Translate towards the desired translation.
Post-processing: Analyze the morphological results and potentially apply rule-based corrections to handle nuances of Japanese grammar.
Let me know if you'd like any modifications or enhancements to this program!
もちろんです! 以下は、翻訳ライブラリと形態素解析ツールの機能を組み合わせた Python プログラムです。
import spacy
from googletrans import Translator
from janome.tokenizer import Tokenizer
#Load Spacy’s English language model and Janome’s Japanese tokenizer
nlp = spacy.load(“en_core_web_sm”)
t = Tokenizer()
def translate_and_analyze(text):
“””Translates English text to Japanese and performs morphological analysis.”””
# Translate using Google Translate
translator = Translator()
translation = translator.translate(text, dest=’ja’).text
# Morphological analysis using Janome
tokens = t.tokenize(translation)
analysis = []
for token in tokens:
analysis.append(
f”{token.surface} ({token.part_of_speech}, {token.infl_type}, {token.infl_form})”
)
return translation, analysis
#Example usage
english_text = “This is a beautiful day for a walk in the park.”
japanese_translation, morphological_analysis = translate_and_analyze(english_text)
print(“Japanese Translation:”, japanese_translation)
print(“Morphological Analysis:”)
for item in morphological_analysis:
print(item)
これがどのように機能するか:
ライブラリ:
spacy: 英語用の強力な NLP ライブラリ。主に、入力テキストが翻訳前に適切にトークン化されていることを確認するためにここで使用されます。
googletrans: 正確な翻訳のために Google Translate の API を利用します。expand_more
janome: 単語を原形や品詞などに分解する日本語形態素解析ライブラリ。
機能:
翻訳と分析(テキスト):
英語のテキストを入力として受け取ります。
Google翻訳を使って日本語に翻訳します。
Janome を使用して日本語テキストの形態素解析を実行します。
翻訳されたテキストと詳細な形態学的分析の両方を返します。
出力:
プログラムは翻訳された日本語文を印刷します。
次に、各日本語の単語/形態素を以下の内容とともにリストします。
ベース形状(表面)
品詞
語形変化の種類
語形変化 (該当する場合)
インストール:
Python がインストールされていることを確認してください。
pip を使用して必要なライブラリをインストールします。
pip install spacy googletrans janome
Spacy 英語モデルをダウンロードします。
python -m spacy download en_core_web_sm
精度のための主な改善点:
前処理: 翻訳前に、英語のテキストにさらに高度な前処理 (短縮形の処理、スラングの正規化など) を追加することを検討してください。
カスタム辞書: ドメイン固有の用語については、Google 翻訳を目的の翻訳に導くためのカスタム辞書を作成します。
後処理: 形態素の結果を分析し、日本語文法のニュアンスを処理するためにルールベースの修正を適用する可能性があります。
このプログラムに変更や機能拡張が必要な場合はお知らせください。
GPT-4oの結果はこちら
Here is a Python program that translates English text into Japanese and performs morphological analysis using `googletrans` for translation and `mecab-python3` for morphological analysis:
### Requirements:
1. `googletrans` for translation.
2. `mecab-python3` for morphological analysis.
### Installation:
Install the required Python packages using `pip`:
```bash
pip install googletrans==4.0.0-rc1
pip install mecab-python3
```
### Python Program:
Below is the complete Python script:
```python
from googletrans import Translator
import MeCab
def translate_text(text, dest='ja'):
translator = Translator()
translation = translator.translate(text, dest=dest)
return translation.text
def morphological_analysis(text):
tagger = MeCab.Tagger()
return tagger.parse(text)
def main():
# Input English text
english_text = "Hello, how are you?"
print("English Text:", english_text)
# Translate English to Japanese
japanese_translation = translate_text(english_text)
print("Translated Text:", japanese_translation)
# Perform morphological analysis on the Japanese text
analysis = morphological_analysis(japanese_translation)
print("Morphological Analysis:\n", analysis)
if __name__ == "__main__":
main()
```
### Explanation:
- **Translation**: The `translate_text` function uses `googletrans` to translate an English string into Japanese.
- **Morphological Analysis**: The `morphological_analysis` function uses `MeCab` to perform morphological analysis on the translated Japanese text.
- **Main Function**: Combines these processes and prints the original English text, its Japanese translation, and the morphological analysis of the Japanese text.
This program allows you to input English text, get its Japanese translation, and analyze the structure of the translated text using morphological analysis.
英語のテキストを日本語に翻訳し、形態素解析を行うプログラムを以下に示します。このプログラムでは、翻訳に googletrans
ライブラリを使用し、形態素解析に mecab-python3
ライブラリを使用します。
必要条件:
googletrans
:翻訳用mecab-python3
:形態素解析用
インストール:
以下のコマンドを使用して必要なPythonパッケージをインストールします。
pip install googletrans==4.0.0-rc1
pip install mecab-python3
Pythonプログラム:
以下に完全なPythonスクリプトを示します。
from googletrans import Translator
import MeCab
def translate_text(text, dest='ja'):
translator = Translator()
translation = translator.translate(text, dest=dest)
return translation.text
def morphological_analysis(text):
tagger = MeCab.Tagger()
return tagger.parse(text)
def main():
# 英語のテキストを入力
english_text = "Hello, how are you?"
print("英語のテキスト:", english_text)
# 英語を日本語に翻訳
japanese_translation = translate_text(english_text)
print("翻訳されたテキスト:", japanese_translation)
# 翻訳された日本語テキストの形態素解析を実行
analysis = morphological_analysis(japanese_translation)
print("形態素解析:\n", analysis)
if __name__ == "__main__":
main()
説明:
- 翻訳:
translate_text
関数は、googletrans
を使用して英語の文字列を日本語に翻訳します。 - 形態素解析:
morphological_analysis
関数は、MeCab
を使用して翻訳された日本語テキストの形態素解析を行います。 - メイン関数: オリジナルの英語テキスト、翻訳された日本語テキスト、およびその形態素解析結果を表示します。
このプログラムを使用すると、英語のテキストを入力して日本語に翻訳し、翻訳されたテキストの構造を形態素解析で分析することができます。
結果は、どちらも基本的には正しく動作するコードを出力してくれました。
どちらも必要なパッケージのインストール手順も説明されており、そのままでも動作はしますが、エラーハンドリングがなかったりと、改善の余地はあります。
コーディング能力にそこまで差はなく、どちらもかなり高い水準の能力を持っていると感じます。
今回の検証の結果、Gemini 1.5 ProはGPT-4oと同等の性能を有していることが分かりました。
さらに、コンテキストウィンドウは圧倒的にGeminiのほうが大きいので、動画の文字起こしなどのタスクは、Gemini 1.5 Proのほうが有利かもしれません。
なお、最新のGPT-4oについて知りたい方は、以下の記事をご覧ください。
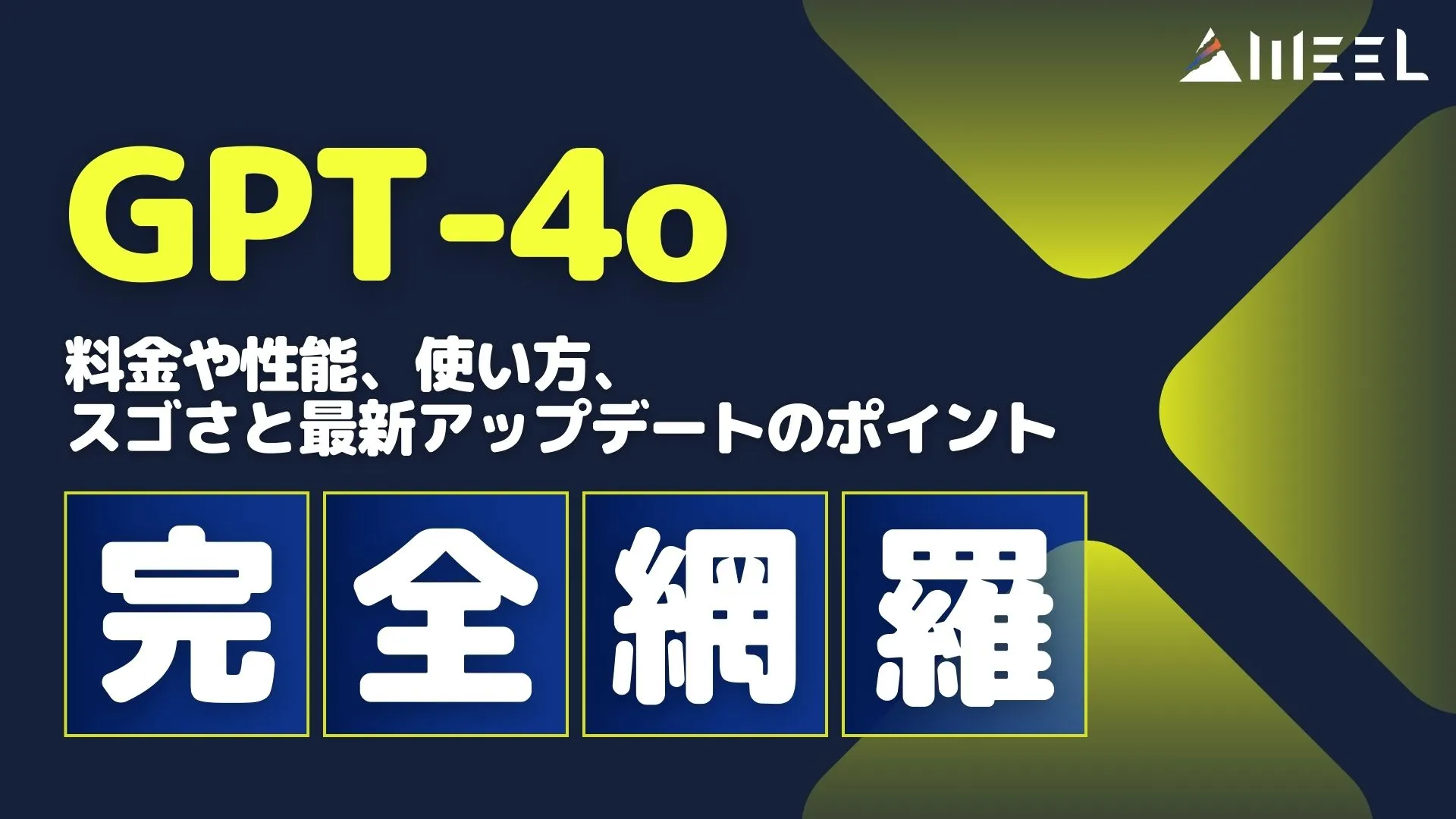
2024年5月14日の発表で、さらに進化したGemini 1.5 Proは、単純な性能が大幅に向上しただけでなく、現在はまだ使用できませんがなんと200万トークンのコンテキストウィンドウを獲得しています。
他の追随を許さない圧倒的なコンテキストウィンドウによって、他のモデルではできなかったようなタスクをこなすことが可能になります。
また、このGemini 1.5 ProはGoogleのAIチャットサービスであるGemini Advancedに搭載されました。
これにより、Gemini Advancedでは最大 1,500 ページに及ぶドキュメントを理解したり、100件の電子メールを要約したりすることが可能になりました。
また、まもなく1 時間のビデオ コンテンツや 30,000 行を超えるコードベースを処理できるようになるとのことです。
Gemini Advancedに登録してなくても、Google AI StudioやVertex AIからも気軽に利用できるので、もし気になった方は是非試してみてください!
入力トークン数が大幅増加したGemini 1.5 Pro
当記事では、Googleが送る次世代のMLLM「Gemini 1.5」を紹介しました。そのすごいところは、中型モデルのGemini 1.5 Proでも……
● 性能テストで先代の大型モデル・Ultra 1.0と互角
● Transformerの進化系、MoEアーキテクチャを搭載
● LLM史上最大、100万トークンもの入力に対応
を達成していることにありましたね。
GPT-4との比較では、以下のような結果になりました。
タスク | Gemini 1.5 Proの結果 | GPT-4の結果 |
---|---|---|
画像認識の深層学習モデルの構築 | 76%の精度を達成 | 62%の精度を達成 |
日本語のビジネスメール作成 | 202文字程度の自然なメールを作成できた | 615文字程度の自然なメールを作成できた |
18禁の内容を出力できるのか | 出力できなかった | 1800文字程度の短いストーリーを作成できた |
将来的には、YouTubeやAIチャットボットにGemini 1.5が実装されるかも……しれませんよ。
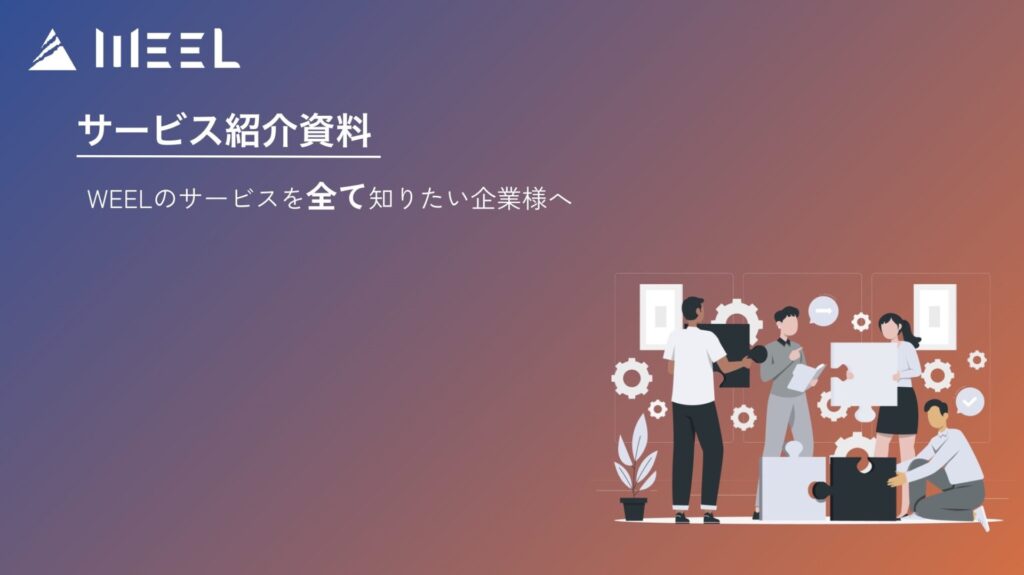
生成系AIの業務活用なら!
・生成系AIを活用したPoC開発
・生成系AIのコンサルティング
・システム間API連携
最後に
いかがだったでしょうか?
GPT-3.5 Turboの最新アップデートで、より高速かつ低コストでのAI活用が可能になりました。自社での導入・活用を検討する際に、最適なモデル選定や活用方法について、一緒に考えてみませんか?
弊社では
・マーケティングやエンジニアリングなどの専門知識を学習させたAI社員の開発
・要件定義・業務フロー作成を80%自動化できる自律型AIエージェントの開発
・生成AIとRPAを組み合わせた業務自動化ツールの開発
・社内人事業務を99%自動化できるAIツールの開発
・ハルシネーション対策AIツールの開発
・自社専用のAIチャットボットの開発
などの開発実績がございます。
まずは、「無料相談」にてご相談を承っておりますので、ご興味がある方はぜひご連絡ください。
➡︎生成AIを使った業務効率化、生成AIツールの開発について相談をしてみる。
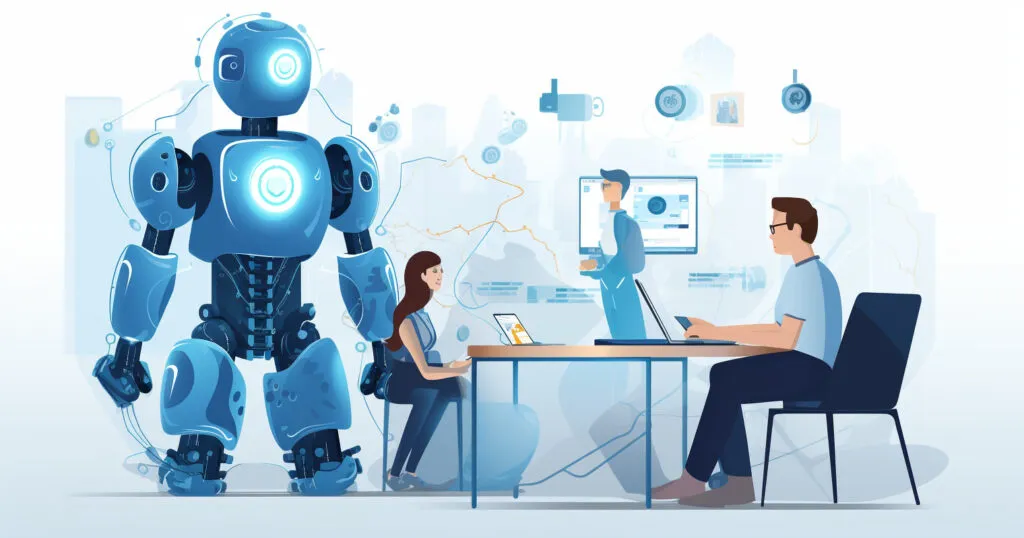
「生成AIを社内で活用したい」「生成AIの事業をやっていきたい」という方に向けて、生成AI社内セミナー・勉強会をさせていただいております。
セミナー内容や料金については、ご相談ください。
また、サービス紹介資料もご用意しておりますので、併せてご確認ください。