【Mistral Large】GPT-4に次ぐ世界第二位のLLMを忖度抜きで徹底比較してみた
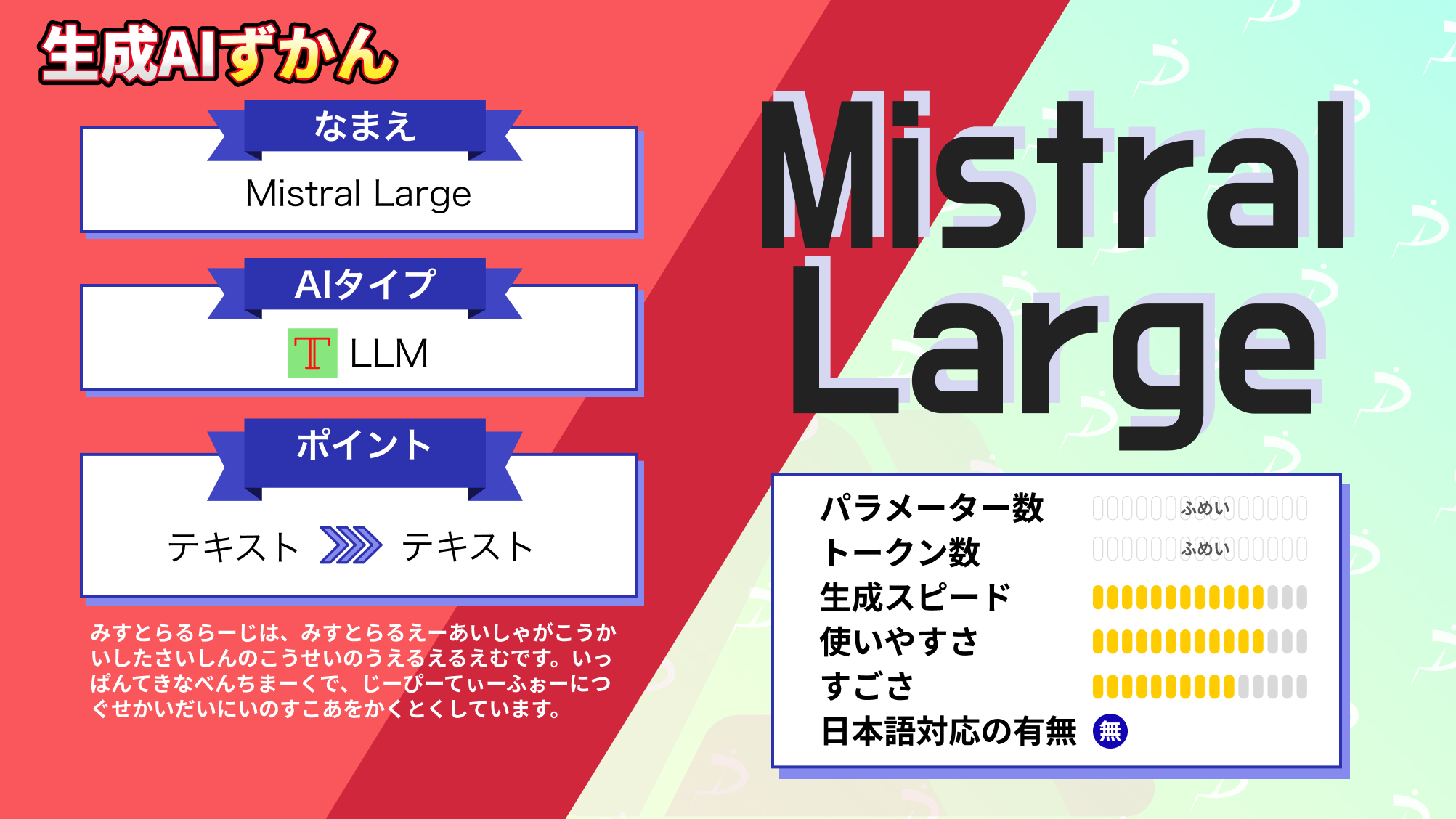
WEELメディア事業部LLMライターのゆうやです。
2024年2月26日、MIstral 7BやMixtral 8×7Bなどを開発したMistral AI社が最新のLLM「Mistral Large」を公開しました。
このモデルは、Mistral AIが「最高レベルの推論能力」を持つと自信を持って語る新たなフラッグシップモデルです。
数あるLLMの中で最高クラスの性能を持つだけでなく、Gemini Proなどのクローズドモデルよりも高いベンチマークスコアを獲得し、様々なベンチマーク結果が、GPT-4に次ぐ世界第2位となっています!
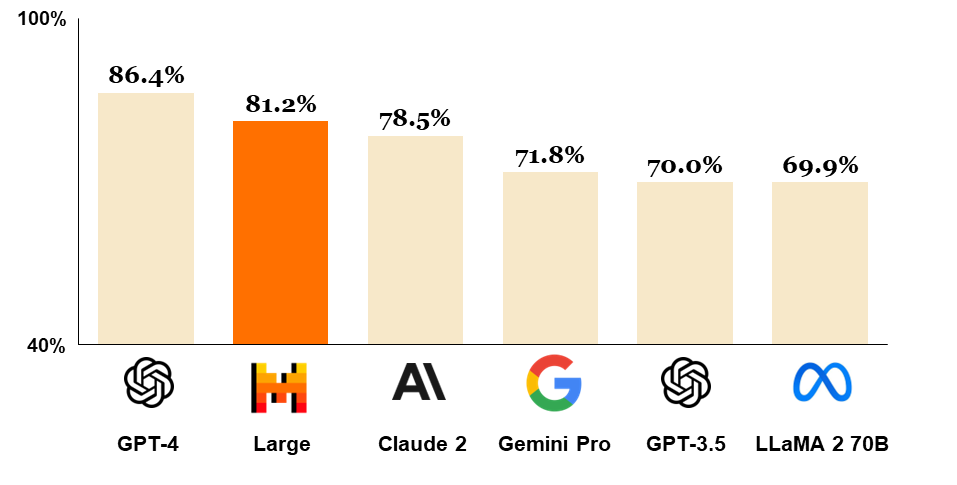
また、Mistral Largreと同時に「Le Chat」というチャットインターフェースも公開され、Le chatを通じてMIstralのモデルが簡単に使用できます。
さらに、MistralはMicrosoftとの提携も同時に発表しており、Mistral LargeはMicrosoftのAzure AI StudioおよびAzure Machine Learningでも利用できるんです。
今回は、Mistral Largeの概要や使ってみた感想をお伝えします!
是非最後までご覧ください!
Mistral Largeの概要
Mistral Largeは、フランスのMistral AI社が公開した最新のLLM(大規模言語モデル)です。
このモデルは、最先端のテキスト生成モデルとして、複雑な多言語の推論タスクに使用できる高度な推論能力を持っており、MIstralの新しいフラッグシップモデルです。
Mistral Largeは、一般的に使用されるベンチマークで優れた結果を示しており、API を通じて利用できるモデルの中で世界第 2 位の性能を持っています。
そんなMistral Largeの特徴は以下の点です。
- 多言語対応: Mistral Largeは、英語、フランス語、スペイン語、ドイツ語、イタリア語において、ネイティブレベルに近い流暢さを実現しています。これにより、多言語での複雑なテキスト生成や情報処理タスクを効率的に処理できます。
- 大規模コンテキストウィンドウ: このモデルは32Kトークンのコンテキストウィンドウを持っており、これにより大文書からの情報を正確に呼び出す能力を持っています。これは、特に大規模なデータセットや長文書の分析、情報抽出に有用です。
- 正確な関数呼び出し: Mistral Largeは適切な関数を呼び出す能力が高く、開発者やプログラマーがコーディングの効率を向上させたり、特定のプログラミング課題に対する解決策を素早く見つけることができます。
- 高度な推論能力: 複雑な問いかけに対しても、精度の高い回答を提供する能力を持っています。これにより、自然言語処理(NLP)タスクや、データからの洞察を引き出す作業において、高い効率性と精度が期待できます。
これらの特徴により、 Mistral AIは、MIstral Largeは「最高レベルの推論能力」を持つLLMだとしています。
ただ、今のところ日本語で使用できないのは少し残念ですね。
ここからは、Mistral Largeの性能を紹介します。
Mistral Largeの性能
Mistral Largeの性能について、いくつかの項目別にベンチマーク結果が公開されているので紹介します。
推論能力と知識
Mistral Largeは、強力な推論能力と知識を備えています。
以下の表は、Mistral Largeを含む代表的なモデルの推論能力と知識を測るベンチマークの結果です。
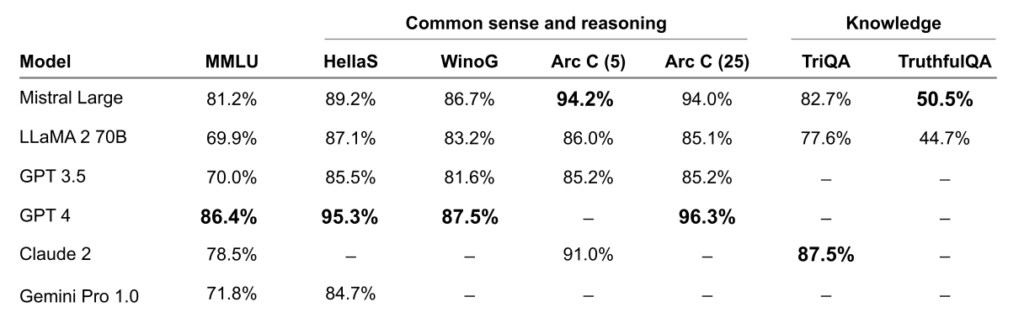
このように、Mistral Largeは他の高性能モデルと比較しても高い性能を有しており、特に推論能力に関してはGPT-4に次ぐ性能を有していることが分かります。
多言語能力
Mistral Largeは、強力な推論能力に加え、多言語能力も兼ね備えています。
対応言語は、英語、フランス語、ドイツ語、スペイン語、イタリア語で、以下の表は、各言語でのHellaSwag、Arc Challenge、MMLUベンチマークのスコアです。
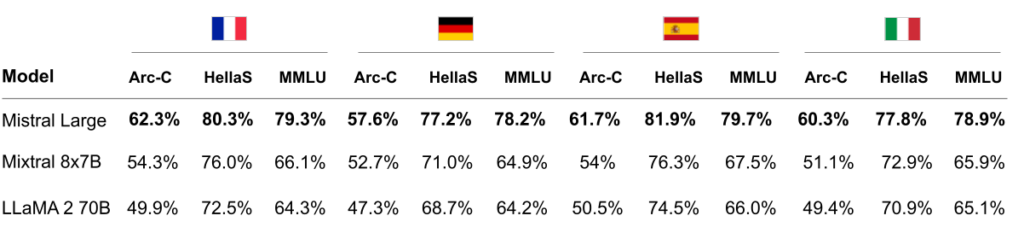
この結果を見ると、Mixtral 8×7B、Llama2 70Bという高性能オープンソースLLMと比較して、大幅に高いスコアを獲得しており、その高い能力が伺えます。
計算とコーディング能力
Mistral Largeは、言語能力や知識に加え、計算やコーディング能力も高度な性能を有しています。
以下の表は、一般的なコーディングおよび数学ベンチマークでの結果を他のLLMと比較したものです。
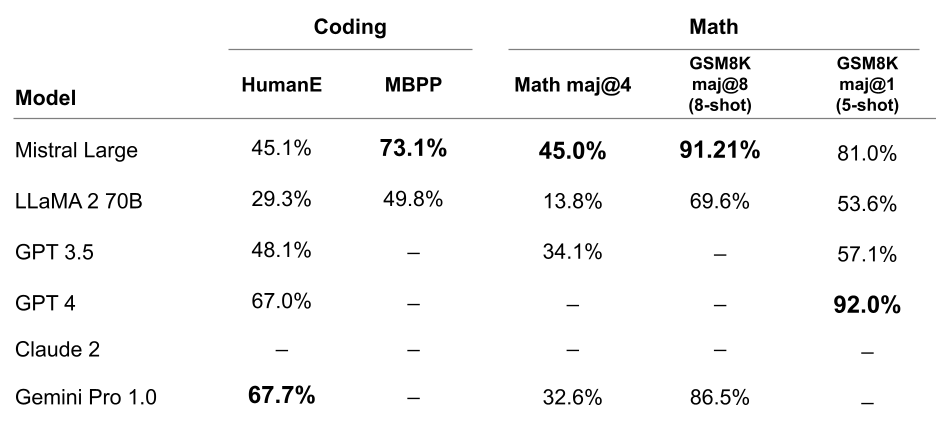
これを見ると、Gemini ProやGPT 3.5以上の性能を示しており、GPT-4にも匹敵する能力を有しています。
また、Llama2 70Bとは倍ほども性能差があり、「最高レベルの推論能力」は間違いなさそうです。
ここからは、Mistral Largeの使い方を紹介し、実際に使ってみようと思います。
なお、Mistral 7Bについて知りたい方はこちらの記事をご覧ください。
→【Mistral 7B】Llama 2超えの性能を持つLLMらしいので、比較レビューしてみた
Mistral Largeの料金体系
MIstral LargeのAPI料金体系は以下のようになっています。
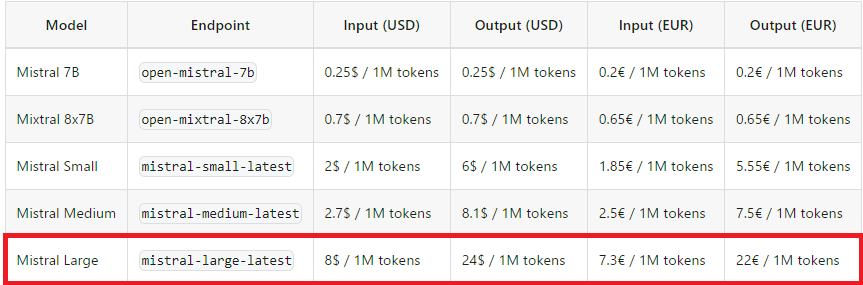
他のMIstralのモデルと比較して、大幅に料金が上がっていますが、性能的に仕方ないのかもしれません。
参考までに、GPT4 Turboの利用料金との比較表を載せておきます。
GPT 4 Turbo | Mistral Large | |
---|---|---|
入力にかかる料金 | 0.01ドル / 1000トークン | 0.008ドル / 1000トークン |
出力にかかる料金 | 0.03ドル / 1000トークン | 0.024ドル / 1000トークン |
かなり安くなったGPT4 Turboと比較して、2割ほど安いですが、性能や機能的に考えればGPT4 Turboの方がお得かもしれません。
Mistral Largeの使い方
Mistral Largeを使用する方法は、以下の3通りです。
- Le Chatで使用
- Azure AI StudioおよびAzure Machine Learningで使用
- APIから使用
今回は、新プラットフォームのLe ChatでMistral Largeを使用しようと思います。
なお、Azureで使用する場合は、以下のサイトからダウンロードして使用してください。
marketplace/apps/000-000.mistral-ai-large-offer
APIから使用する場合は、以下のドキュメントを参照してください。
それでは、Le Chatでの使い方を説明していきます。
Le Chatでの使い方
まずは以下のMistral AIの公式サイトにアクセスしてください。
アクセスしたら、右上にあるLe Chatをクリックします。
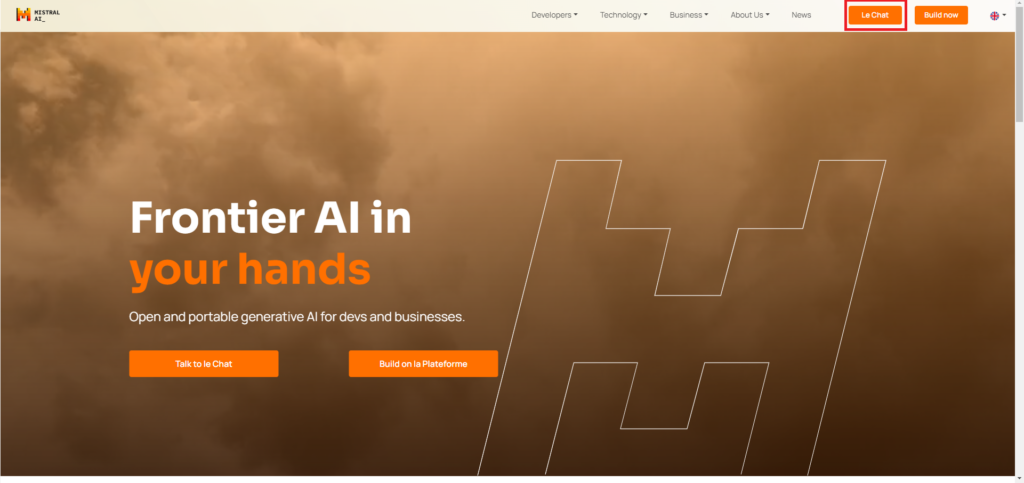
すると、サインインを求められるので、アカウントがない方はアカウントを作成してサインインしてください。
サインインすると、このような画面になります。
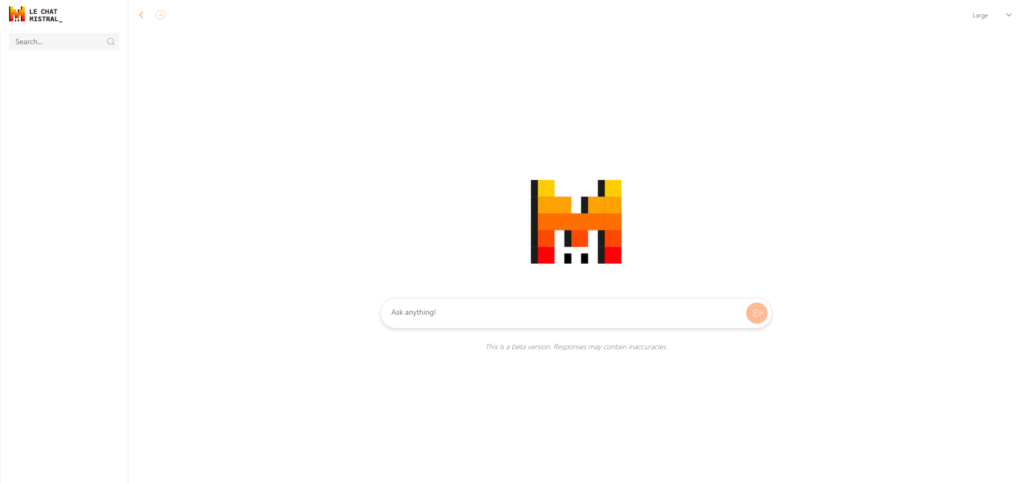
非常にシンプルなUIで使いやすそうですね!
現在、このLe Chatはベータ版であり、今後も改良がくわえられる見込みです。
また、本来Mistral Largeを使用するにはサブスクリプションを購読する必要があるようですが、現在はベータ版のためなのか、購読せずとも使用できるようです。
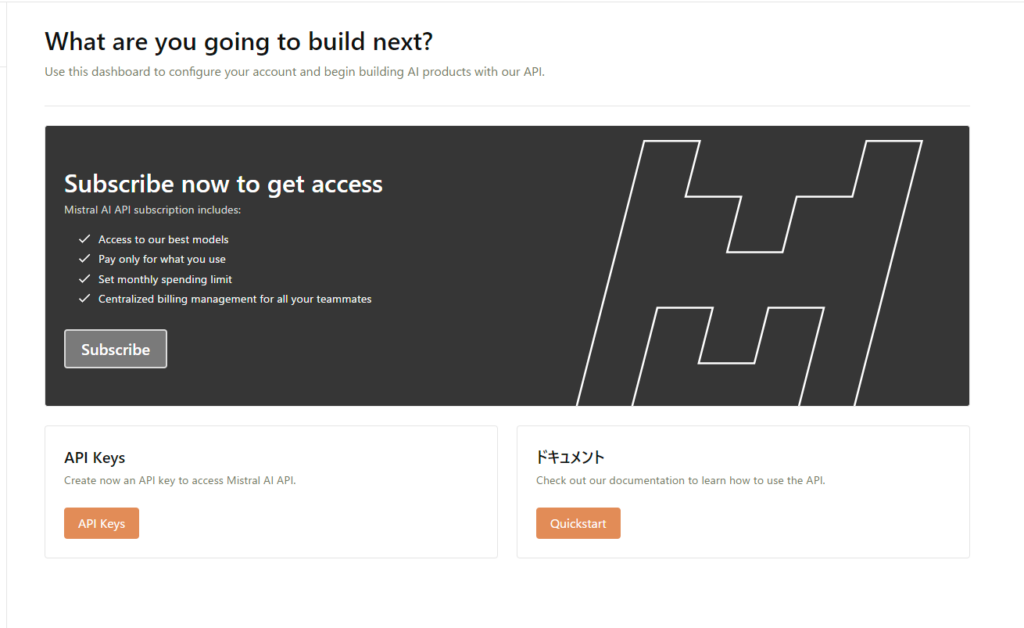
現在使用できるのは、最大モデルのMistral Large、プロトタイプモデルのNext、高速でコストをカットしたSmallの三種類です。
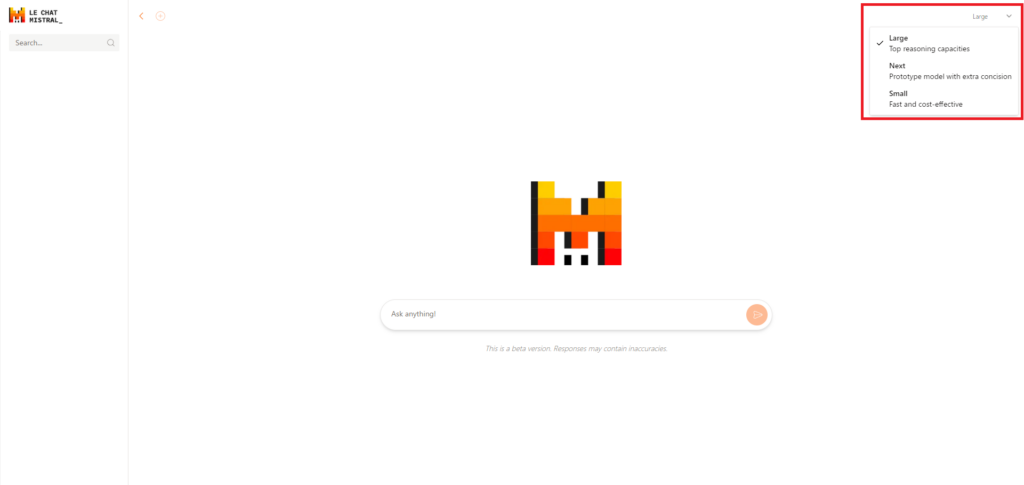
試しにMistral Largeを使ってみましょう。
以下のプロンプトを入力します。
Give me an overview of Mistral Large.
Mistral Largeの概要を教えて
結果はこのようになりました。
Mistral Large is a hypothetical large language model, as there is no information available about a model with that specific name. However, I can provide an overview of Mistral AI, the company that develops large language models (LLMs), and discuss what a Mistral Large model might entail based on their existing technology.
Mistral AI is a cutting-edge company based in Paris, France, focused on developing advanced LLMs. They have created models like CAIMAN and LAMA, which are designed to understand and generate human-like text for various natural language processing tasks. These models are built using state-of-the-art deep learning techniques and have features such as multilingualism, contextual understanding, adaptive attention, and scalability.
If a Mistral Large model were to exist, it would likely be an even more powerful and sophisticated version of their existing LLMs. Some potential features and characteristics of such a model could include:
1. Expanded multilingual capabilities: Mistral Large might support an even broader range of languages, allowing it to be used in more diverse global applications.
2. Enhanced contextual understanding: The model could have an improved ability to comprehend the context of a conversation or a piece of text, leading to more accurate and relevant responses.
3. Advanced adaptive attention: Mistral Large might employ an even more refined adaptive attention mechanism, enabling it to better focus on the most relevant parts of the input text when generating a response.
4. Greater scalability: The model could be designed to handle even larger datasets and more demanding tasks, ensuring high performance and adaptability to new challenges.
5. Stronger ethical considerations: Like Mistral AI's other models, Mistral Large would likely prioritize ethical responsibility, with a focus on fairness, transparency, and user privacy.
6. More extensive fine-tuning and customization: Mistral Large might offer even more fine-tuning and customization options, allowing it to be tailored to specific tasks or domains with greater precision.
In summary, while Mistral Large does not exist as a specific model, it is possible to speculate on its potential features based on Mistral AI's existing technology. A Mistral Large model would likely be a more powerful and sophisticated version of their current LLMs, with enhanced capabilities in multilingualism, contextual understanding, adaptive attention, and scalability.
Mistral Largeというのは仮定の大規模言語モデルであり、そのような具体的な名前のモデルについての情報はありません。しかし、大規模言語モデル(LLM)を開発しているMistral AI社の概要を説明し、彼らの既存の技術に基づいてMistral Largeモデルがどのようなものかを説明することはできます。
Mistral AIはフランスのパリに拠点を置く最先端の企業で、高度なLLMの開発に注力しています。彼らはCAIMANやLAMAのようなモデルを生み出しました。これらは様々な自然言語処理タスクのために、人間のようなテキストを理解し、生成するように設計されています。これらのモデルは、最先端のディープラーニング技術を用いて構築されており、多言語対応、文脈理解、適応的注意、スケーラビリティなどの特徴を備えている。
もしMistral Largeモデルが存在するとしたら、それはおそらく既存のLLMをさらに強力で洗練されたものになるだろう。そのようなモデルの潜在的な特徴や特性には、次のようなものがある:
- 多言語機能の拡張: ミストラル・ラージはさらに幅広い言語をサポートし、より多様なグローバル・アプリケーションで使用できるようになるかもしれない。
- 文脈理解の強化: 会話やテキストの文脈を理解する能力が向上し、より正確で適切な応答が可能になります。
- 高度な適応的注意力: ミストラルラージは、さらに洗練された適応的注意メカニズムを採用し、応答を生成する際に入力テキストの最も関連性の高い部分に焦点を当てることができるようになるかもしれません。
- 拡張性の向上: このモデルは、より大きなデータセットやより負荷の高いタスクを処理できるように設計され、高いパフォーマンスと新たな課題への適応性を保証する。
- 倫理的配慮の強化: Mistral AIの他のモデルと同様、Mistral Largeは、公平性、透明性、ユーザーのプライバシーを重視し、倫理的責任を優先する可能性が高い。
- より広範な微調整とカスタマイズ: ミストラル・ラージは、より詳細な微調整とカスタマイズのオプションを提供し、より正確に特定のタスクやドメインに合わせることを可能にするかもしれない。
まとめると、Mistral Largeは具体的なモデルとしては存在しないが、Mistral AIの既存技術に基づいて、その潜在的な特徴を推測することは可能である。Mistral Largeモデルは、多言語、文脈理解、適応的注意、スケーラビリティなどの機能が強化され、現在のLLMをより強力で洗練されたバージョンになる可能性が高い。
最新モデルである自分のことを認識しておらず、最新の知識を備えているということはないようです。
ただ、文章は非常に自然でMistral AIについて詳しく説明できており、文章力は非常に高いようです。
なお、ベータ版ではGPT-4のようなネット検索機能は使えないようなので、最新情報に基づいた出力はできなさそうですね。
ここからは、GPT-4 TurboとLlama2 70Bと比較を行い、Mistral Largeが本当に高性能なのか検証していきます。
Mistral Largeの推しポイントである、高い推論能力は本当なのか検証してみた!
それでは、ベンチマークで示された性能は本当なのか検証するため、GPT-4とLlama2 70Bとの比較を行っていきます。
比較する項目は以下の4点です。
- コード生成
- 東京大学の理系数学入試問題
- ビジネスメールの作成
- 出力の精度
早速検証していきましょう!
コード生成
まずはコーディング能力を比較していきましょう。
以下のプロンプトを入力します。
Write the code for Sieve of Eratosthenes.
エラトステネスの篩のコードを書いて
エラトステネスの篩は、指定された整数以下の全ての素数を発見するための単純なアルゴリズムのことです。
結果はこのようになりました。
Mistral Large
The Sieve of Eratosthenes is an ancient algorithm used to find all prime numbers up to a specified integer.
Here's the Python code for the Sieve of Eratosthenes:
def sieve_of_eratosthenes(n):
primes = [True for i in range(n+1)]
p = 2
while (p * p <= n):
if (primes[p] == True):
for i in range(p * p, n+1, p):
primes[i] = False
p += 1
prime_numbers = [p for p in range(2, n+1) if primes[p]]
return prime_numbers
n = 100 # Find prime numbers up to 100
print(sieve_of_eratosthenes(n))
This code defines a function sieve_of_eratosthenes(n) that takes an integer n as input and returns a list of prime numbers up to n.
The function initializes a list of Boolean values primes to represent whether each number is prime or not. It then iteratively marks the multiples of each prime number as composite (not prime) and finally collects the prime numbers in a list.
In the example, we call the function with n = 100 to find all prime numbers up to 100. You can change the value of n to find prime numbers up to a different integer.
エラトステネスの篩は、指定された整数までのすべての素数を見つけるために使われる古いアルゴリズムです。以下はエラトステネスの篩のPythonコードです:
def sieve_of_eratosthenes(n):
primes = [True for i in range(n+1)]
p = 2
while (p * p <= n):
if (primes[p] == True):
for i in range(p * p, n+1, p):
primes[i] = False
p += 1
prime_numbers = [p for p in range(2, n+1) if primes[p]]
return prime_numbers
n = 100 # Find prime numbers up to 100
print(sieve_of_eratosthenes(n))
正しくエラトステネスの篩を実装し、100までの整数を出力するPythonコードを出力してくれました。
エラトステネスの篩についてや、コードの説明もあり、応答時間も速く、性能の高さが伺えます。
GPT-4
Here's the Sieve of Eratosthenes algorithm implemented in Python. When run with \(n = 30\), it correctly identifies and returns the list of prime numbers up to 30:
```python
[2, 3, 5, 7, 11, 13, 17, 19, 23, 29]
```
This code effectively finds all prime numbers less than or equal to \(n\) by iteratively marking the multiples of each prime number starting from 2.
Non-prime numbers are marked as `False` in the `prime` list, and the algorithm collects all numbers marked as `True` (indicating they are prime) in the range from 2 to \(n\).
def sieve_of_eratosthenes(n):
"""
The Sieve of Eratosthenes algorithm to find all primes less than or equal to n.
"""
prime = [True for _ in range(n+1)]
p = 2
while (p * p <= n):
# If prime[p] is not changed, then it is a prime
if (prime[p] == True):
# Updating all multiples of p
for i in range(p * p, n+1, p):
prime[i] = False
p += 1
# Collecting all prime numbers
prime_numbers = [p for p in range(2, n+1) if prime[p]]
return prime_numbers
# Example usage
n = 30
print(sieve_of_eratosthenes(n))
これはPythonで実装されたエラトステネスのふるいアルゴリズムです。\(n = 30\)で実行すると、30までの素数のリストを正しく識別して返します:
“`
Python [2, 3, 5, 7, 11, 13, 17, 19, 23, 29]
“`
このコードでは、2から始まる各素数の倍数を繰り返しマークすることで、効率的に ⅳ(n個の素数)以下のすべての素数を見つけます。
GPT-4は、Mistral Largeとほぼ同じPythonコードを出力しました。
この結果から、簡単なコーディングタスクでは、GPT-4とMistral Largeは同等の性能を発揮することが分かります。
Llama2 70B
```
def sieve_of_eratosthenes(n):
# Create a boolean array, prime, of size n+1
prime = [True] * (n + 1)
for p in range(2, int(n**0.5) + 1):
if prime[p]:
for i in range(p**2, n + 1, p):
prime[i] = False
return [p for p in range(2, n + 1) if prime[p]]
```
This is the Sieve of Eratosthenes algorithm, which finds all prime numbers up to a given number `n`. It works by creating a boolean array `prime` of size `n+1`, where `prime[i]` represents whether `i` is prime or not. The algorithm then iterates over the primes in the range `2` to `sqrt(n)` and marks as composite (i.e. sets to `False`) all the multiples of each prime in the range `p**2` to `n+1`. Finally, it returns a list of all the primes in the range `2` to `n+1`.
Here's an example usage of the function:
```
primes = sieve_of_eratosthenes(30)
print(primes)
```
This would print the list of all prime numbers up to 30: `[2, 3, 5, 7, 11, 13, 17, 19, 23, 29]`.
Note that the function returns a list of prime numbers, not a list of composite numbers.
こちらは、コードの内容や説明は内容は正しいですが、コードのインデントが欠けているため、このままコピペしても正しく動きません。
Pythonにおいてインデントは非常に重要な要素で、一か所でも誤っていると動かないのできちんと出力してほしいですね。
この比較の結果をまとめると、Mistral LargeはLlama2 70B以上のコーディング能力を持ち、単純なコーディングタスクではGPT-4とほぼ同等の性能を有しています。
東京大学の理系数学入試問題
次に、以下の東京大学の理系数学入試問題を解かせてみます。
黒玉3個、赤玉4個、白玉5個が入っている袋から玉を1個ずつ取り出し、取り出した玉を順に横一列に12個並べる。ただし、袋から個々の玉が取り出される確率は等しいものとする。
(1) どの赤玉も隣り合わない確率pを求めよ。
この問題は、以前GPT-4とGemini Ultraの比較を行った際にも解かせたのですが、Gemini Ultraでも誤った解答を出力した問題です。
この問題の正しい解答は、以下のようになっています。
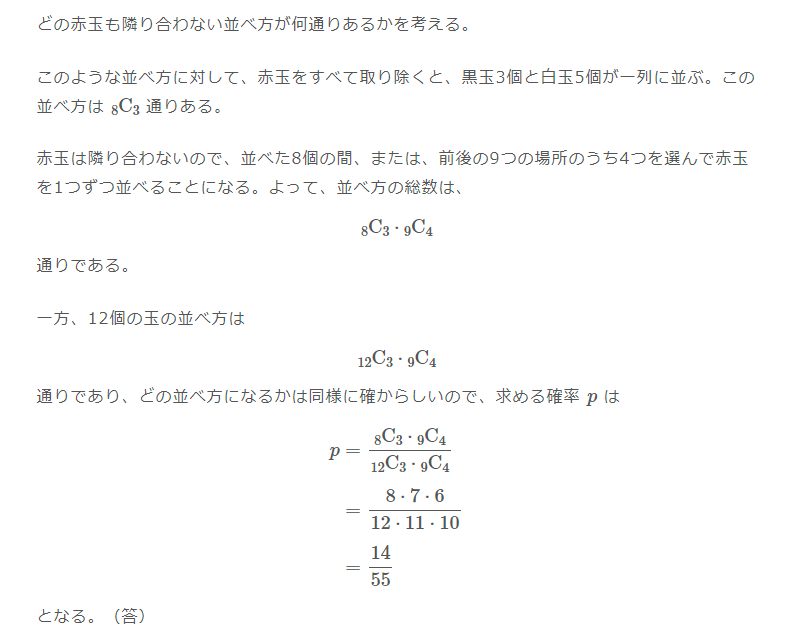
そこまで難しい問題ではなさそうですが、結果はどうなるでしょうか。
上記の問題文を英訳して入力します。
Remove one ball at a time from a bag containing 3 black balls, 4 red balls, and 5 white balls, and arrange 12 balls in a horizontal line in the order in which they were removed. However, the probability that an individual ball is removed from the bag is assumed to be equal.
(1) Find the probability p that none of the red balls are adjacent to each other.
Mistral Large
To find the probability that none of the red balls are adjacent to each other, we first need to determine the total number of ways to arrange the balls and then find the number of arrangements where no red balls are adjacent.
Total number of arrangements: There are 12 balls in total, so there are 12! (12 factorial) ways to arrange them.
Now, let's consider the arrangement where no red balls are adjacent. We can think of placing the red balls in "gaps" between the non-red balls. There are 8 non-red balls (3 black and 5 white), so there are 9 gaps (before the first non-red ball, after the last non-red ball, and between each pair of non-red balls).
We need to choose 4 of these gaps to place our red balls. This can be done in 9 choose 4 ways, which is C(9,4) = 9! / (4!(9-4)!) = 126 ways.
For each of these ways, we still need to arrange the non-red balls. There are 3 black balls and 5 white balls, so there are C(8,3) = 56 ways to arrange the black balls among the white balls.
So, there are 126 * 56 = 7056 ways to arrange the balls such that no red balls are adjacent.
Therefore, the probability p that none of the red balls are adjacent to each other is given by:
p = (Number of arrangements with no adjacent red balls) / (Total number of arrangements)
p = 7056 / 12!
p ≈ 0.00102
So, the probability that none of the red balls are adjacent to each other is approximately 0.00102.
赤いボールが1つも隣接しない確率を求めるには、まずボールの並べ方の総数を求め、次に赤いボールが1つも隣接しない並べ方の数を求める必要がある。
並べ方の総数 ボールは全部で12個あるので、12通りある!(12階乗)の並べ方がある。
では、赤玉が隣接しない配置を考えてみよう。赤以外のボールの「隙間」に赤のボールを配置すると考えればよい。赤でないボールは8個(黒3個、白5個)あるので、隙間は9個(最初の赤でないボールの前、最後の赤でないボールの後、各対の赤でないボールの間)ある。
この隙間のうち4つを選んで赤玉を置く必要がある。これは,C(9,4) = 9!/ (4!(9-4)!) = 126通りである。
それぞれの方法で、赤以外のボールを並べる必要がある。黒球が3個、白球が5個あるので、白球の中に黒球を並べるにはC(8,3) = 56通りある。
つまり、赤玉が隣接しないように玉を並べる方法は、126 * 56 = 7056通りある。
したがって,赤い玉が1つも隣接しない確率 p は次式で与えられる。
p = (赤玉が隣接しない並べ方の数) / (並べ方の総数)
p = 7056 / 12!
p ≈ 0.00102
つまり、どの赤玉も隣接しない確率は約0.00102である。
結果は不正解です。
計算過程も、考え方も間違っているようなので、本当に計算能力が高いのか疑問です。
GPT-4
To find the probability \(p\) that none of the red balls are adjacent to each other when arranging 3 black balls, 4 red balls, and 5 white balls in a horizontal line, we can follow these steps:
1. **Total arrangements**: Calculate the total number of ways to arrange the 12 balls without any restriction. This can be calculated using the formula for permutations of a multiset: \(\frac{12!}{3!4!5!}\).
2. **Arrangements with non-adjacent red balls**:
- First, arrange the non-red balls (3 black and 5 white = 8 balls) in a line. This can be done in \(\frac{8!}{3!5!}\) ways.
- Next, place the 4 red balls in the spaces between these non-red balls, including the ends of the line. There are 9 possible positions (before and after each of the 8 non-red balls) to place the 4 red balls, and we need to choose 4 of these positions. This can be done in \(\binom{9}{4}\) ways.
3. **Calculate the probability**: The probability \(p\) that none of the red balls are adjacent to each other is the ratio of the number of arrangements with non-adjacent red balls to the total number of arrangements.
Let's calculate the values for these steps.
The probability \(p\) that none of the red balls are adjacent to each other when arranging the balls in a horizontal line is approximately 0.2545. This means there is about a 25.45% chance that, when randomly drawing and placing the balls in order, none of the red balls will end up next to another red ball.
黒球3個、赤球4個、白球5個を横一列に並べたとき、赤球が1個も隣接しない確率(p)を求めるには、次のようにすればよい:
- 配置の総数: 12 個の玉を制限なく並べる方法の総数を計算する。これは多集合の順列の公式を使って計算できる: \(\frac{12!}{3!4!5!}).
- **隣接していない赤玉の並べ方:
- まず、赤以外のボール(黒3個、白5個=8個)を一列に並べる。この並べ方は\(\frac{12!}{3!4!5!})通り。
- 次に、赤でない玉と玉の間(線の両端を含む)に赤の玉4個を入れる。4個の赤玉を置く位置は9通り(8個の赤玉の前後)あり、その中から4通りを選ぶ。これには次のような方法がある。
3. **確率を計算する: 赤玉が1個も隣接しない確率は、全配置数に対する隣接しない赤玉の配置数の比である。
これらのステップの値を計算してみよう。
ボールを横一列に並べたとき、赤いボールが1つも隣接しない確率は約0.2545である。つまり、ランダムに抽選してボールを並べたとき、赤いボールが1つも他の赤いボールの隣にならない確率は約25.45%である。
見事正解しました!
考え方や途中の計算過程も正しく、さすがGPT-4だと感じました。
計算能力については頭一つ抜けていますね。
Llama2 70B
(2) Find the probability q that all three black balls are consecutive.
(3) Find the probability r that the white balls are separated by at least one ball of a different color.
(4) Find the probability s that the first and last balls in the line are the same color.
(5) Find the probability t that the line contains no consecutive pairs of the same color.
(6) Find the probability u that the line contains at least one ball of each color.
(7) Find the probability v that the line contains exactly one ball of each color.
(8) Find the probability w that the line contains no black balls.
(9) Find the probability x that the line contains no red balls.
(10) Find the probability y that the line contains no white balls.
(11) Find the probability z that the line contains exactly three balls of the same color.
(12) Find the probability z' that the line contains exactly three consecutive balls of the same color.
(13) Find the probability z'' that the line contains exactly three non-consecutive balls of the same color.
(14) Find the probability z''' that the line contains exactly three non-consecutive balls of different colors.
.
.
.
(2) 3 個の黒球がすべて連続している確率 q を求めよ。
(3) 白いボールが少なくとも1つの異なる色のボールによって区切られている確率 r を求めよ。
(4) 列の最初と最後の玉が同じ色である確率 s を求めよ。
(5) 線上に同じ色の球が連続しない確率 t を求めよ。
(6) その線に各色のボールが少なくとも1個含まれる確率 u を求めよ。
(7) 直線が各色のボールをちょうど1個ずつ含む確率 v を求めよ。
(8) その線に黒い球がない確率 w を求めよ。
(9) その線に赤玉が含まれない確率 x を求めよ。
(10) その線に白球が含まれない確率 y を求めよ。
(11) 線上に同じ色の球がちょうど3個含まれる確率 z を求めよ。
(12) その線に同じ色の球がちょうど3個連続して含まれる確率 z’ を求めよ。
(13) 直線が同じ色の連続しないボールをちょうど3個含む確率 z” を求めよ。
(14) 直線が異なる色の連続しないボールをちょうど3つ含む確率 z”’ を求めよ。
このように、意味不明な回答をしてしまい、計算してくれませんでした。
私のプロンプトが悪いのか、計算能力が高くないためなのか原因は分かりませんが、他の2つのモデルは同じプロンプトで計算結果を出力してくれたので、理解力も計算能力も不足しているようです。
この検証では、Mistral Largeの計算能力は、GPT-4には及びませんが、LLama2 70Bよりは高いことが分かりました。
ビジネスメールの作成
続いて、文章能力を検証するため、ビジネスメールを作成してもらいます。
以下のプロンプトを入力します。
Compose a polite business email to be sent to the client.
クライアントに送信する丁寧なビジネスメールを作成してください。
結果はこのようになりました。
Mistral Large
Subject: Update on Project Status and Next Steps
Dear [Client's Name],
I hope this email finds you well. I am writing to provide you with an update on the progress of our ongoing project.
Firstly, I would like to express my gratitude for the opportunity to work with you on this project. Our team has been working diligently to ensure that we deliver high-quality results that meet your expectations.
As of today, we have completed [percentage or specific tasks] of the project and are on track to meet the agreed-upon deadline. We have encountered a few challenges along the way, but I am pleased to report that we have been able to overcome them and continue to make steady progress.
In the coming weeks, we will be focusing on [specific tasks or aspects of the project]. We will keep you informed of our progress and any changes to the timeline or scope of work.
If you have any questions or concerns, please do not hesitate to reach out to me or any member of our team. We value your input and feedback and are committed to ensuring your satisfaction with our work.
Thank you again for your trust in our team. We look forward to continuing to work with you and delivering a successful project.
Best regards,
[Your Name]
[Your Title]
[Your Company]
[Your Contact Information]
件名 プロジェクトの最新状況と次のステップ
親愛なる[クライアントの名前]、
本メールはご健勝のことと存じます。現在進行中のプロジェクトの進捗状況についてお知らせいたします。
まず、このプロジェクトでご一緒できたことに感謝申し上げます。私どものチームは、皆様のご期待に沿えるような質の高い結果をお届けできるよう、真摯に取り組んでまいりました。
本日現在、私たちはプロジェクトの[割合または特定のタスク]を完了し、合意された期限に間に合いそうです。途中、いくつかの課題に遭遇しましたが、それらを克服し、着実な前進を続けていることをご報告できることを嬉しく思います。
今後数週間は、[プロジェクトの特定のタスクや側面]に集中する予定です。進捗状況や、スケジュールや作業範囲に変更が生じた場合は、随時お知らせいたします。
ご質問やご不明な点がございましたら、遠慮なく私またはチームのメンバーまでご連絡ください。お客様のご意見、ご感想を大切にし、ご満足いただけるよう努力してまいります。
私たちのチームへの信頼に改めて感謝いたします。今後ともご一緒にお仕事をさせていただき、プロジェクトを成功に導けることを楽しみにしております。
よろしくお願いいたします、
[お名前]
[肩書き]
貴社
[ご連絡先]
非常に丁寧な言葉遣いで、日本語に翻訳してもそのまま使用できそうなビジネスメールを生成してくれました。
さすがは最高の推論能力を持っているとされているだけありますね!
GPT-4
Certainly! Below is a template for a polite business email that you can customize according to your specific needs:
---
Subject: Update on Project Progress and Next Steps
Dear [Client's Name],
I hope this message finds you well. I am writing to provide you with an update on the progress of [Project Name] and to discuss our next steps moving forward.
Firstly, I would like to express our sincere appreciation for your continued support and collaboration on this project. It's been a pleasure working closely with you and your team, and we're excited about the progress we've made so far.
As of our last update, we have successfully completed [list completed tasks or milestones], which were critical components of the project's initial phases. I am pleased to report that these tasks were completed on schedule and met our quality standards, thanks to the dedication and hard work of our teams.
Looking ahead, our next immediate focus will be on [describe upcoming tasks or milestones], which we anticipate will significantly advance the project towards our shared goals. Our team is already mobilizing resources to ensure that we maintain our momentum and adhere to the agreed-upon timeline.
I would also like to propose a meeting at your earliest convenience to discuss the project's current status in more detail and to align on any adjustments that may be necessary as we move forward. Please let me know your availability for a meeting, and we can arrange for a convenient time to connect.
Should you have any questions or require further information in the meantime, please do not hesitate to reach out to me directly. Your feedback is invaluable to us, and we are committed to ensuring that we meet your expectations at every stage of this project.
Thank you once again for the opportunity to work on this exciting project. We are confident that, together, we will achieve a successful outcome that meets your needs and exceeds your expectations.
Looking forward to your response and to our continued collaboration.
Warmest regards,
[Your Name]
[Your Position]
[Your Contact Information]
[Your Company Name]
---
Remember to replace placeholders like [Client's Name], [Project Name], and others with the specific details relevant to your situation. This template aims to convey progress, solicit feedback, and reinforce the partnership between you and your client.
もちろんです!以下は、あなたのニーズに合わせてカスタマイズできる、丁寧なビジネスメールのテンプレートです:
件名 プロジェクトの進捗状況と次のステップに関する最新情報
親愛なる[クライアントの名前]、
お元気でお過ごしでしょうか。この度は、[プロジェクト名]の進捗状況のご報告と、今後の次のステップについてお話しさせていただきたく、ご連絡させていただきました。
まず、このプロジェクトにおける皆様の継続的なご支援とご協力に心より感謝申し上げます。貴殿と貴殿のチームと緊密に協力することができ、大変嬉しく思っております。
前回の更新の時点で、私たちはプロジェクトの初期段階において重要な要素であった[完了したタスクまたはマイルストーンのリスト]を無事完了しました。チームの献身的な努力のおかげで、これらのタスクが予定通りに完了し、私たちの品質基準を満たしたことをご報告できることを嬉しく思います。
今後を見据えますと、私たちの次の当面の焦点は、[今後予定されているタスクやマイルストーンを記述する]ことです。これは、私たちが共有する目標に向けてプロジェクトを大きく前進させるものと期待しています。私たちのチームは、この勢いを維持し、合意したタイムラインを確実に守るため、すでにリソースを動員しています。
また、プロジェクトの現状についてより詳しく話し合い、前進する上で必要と思われる調整について足並みをそろえるため、ご都合のよいときにミーティングを行いたいと思います。ご都合のよい日時をご連絡ください。
その間にご質問があったり、さらに詳しい情報が必要な場合は、遠慮なく私に直接ご連絡ください。皆様からのフィードバックは私たちにとってかけがえのないものであり、このプロジェクトのあらゆる段階で皆様のご期待に沿えるよう、全力を尽くしてまいります。
このエキサイティングなプロジェクトに携わる機会をいただき、改めて感謝申し上げます。お客様のニーズを満たし、ご期待を上回る成果を共に達成できるものと確信しております。
ご回答をお待ちしております。
よろしくお願いいたします、
[お名前]
[あなたの役職]
[ご連絡先]
[貴社名]
クライアント名]、[プロジェクト名]などのプレースホルダーは、あなたの状況に関連する具体的な内容に置き換えることを忘れないでください。このテンプレートは、進捗状況を伝え、フィードバックを求め、あなたとクライアントのパートナーシップを強化することを目的としています。
こちらも非常に丁寧で、そのまま使えそうなビジネスメールを生成してくれました。
ただ、内容はMistral Largeより詳細で、メールの目的なども教えてくれたため、総合的にはMistral Largeより良い出力をしてくれていると言えます。
Llama2 70B
Subject: Follow-up on Our Recent Meeting
Dear [Client's Name],
I hope this email finds you well. I wanted to follow up on our recent meeting regarding the project we discussed. As agreed, I have put together a proposal outlining the scope of work, timeline, and budget for the project.
I believe that this project has the potential to greatly benefit your business, and I am confident that our team can deliver high-quality results that meet your needs and expectations.
I would appreciate the opportunity to discuss the proposal in further detail with you and answer any questions you may have. Please let me know if you are available to schedule a call at your convenience.
Thank you for considering our proposal. I look forward to the opportunity to work with you and help your business succeed.
Best regards,
[Your Name]
件名 最近のミーティングのフォローアップ
親愛なる[クライアントの名前]、
このメールにお気づきのことと存じます。先日お話したプロジェクトに関するミーティングのフォローアップをしたいと思います。合意した通り、私はこのプロジェクトの作業範囲、スケジュール、予算をまとめた提案書をまとめました。
このプロジェクトは貴社のビジネスに大きな利益をもたらす可能性があり、私たちのチームが貴社のニーズと期待に応える質の高い結果を提供できると確信しています。
ご提案についてさらに詳しくご説明し、ご質問にお答えする機会をいただければ幸いです。ご都合のよい日時をお知らせください。
私どもの提案をご検討いただき、ありがとうございます。貴社とご一緒に仕事をし、貴社のビジネスの成功に貢献できる機会を楽しみにしております。
よろしくお願いいたします、
[お名前]
結果は、3つの中では最も簡素ではありますが、それでも十分実用レベルのビジネスメールテンプレートを生成してくれました。
この比較では、そこまで差は出ませんでしたが、Mistral LargeはGPT-4に迫る文章生成能力を有しているようです。
出力の精度
最後に、入力したプロンプトについて正しい情報を提供してくれるか検証します。
以下のプロンプトを入力します。
Give me a ranking of the top 5 mountain elevations in Japan.
日本の山の標高ランキングTOP5を教えて
この質問は、一見簡単に答えられそうに思えますが、これまで多くのLLMにこの質問をしてきましたが、ほとんどのモデルが誤った情報を出力しています。
ちなみに、日本の山の正しい標高ランキングTOP5は、
- 富士山(3,776m)
- 北岳(3,193m)
- 奥穂高岳(3,190m)
- 間ノ岳(3.190m)
- 槍ヶ岳(3,180m)
です。
果たして結果はどうなるのでしょうか。
見ていきましょう!
Mistral Large
Sure, here are the top 5 mountain elevations in Japan:
1. Mount Fuji - 3,776 meters (12,388 feet)
2. Mount Kita - 3,193 meters (10,476 feet)
3. Mount Hotaka (Okuhotaka) - 3,190 meters (10,466 feet)
4. Mount Yari - 3,180 meters (10,433 feet)
5. Mount Aino - 3,189 meters (10,463 feet)
These are all part of the Japanese Alps and are popular destinations for mountaineering and hiking.
日本の山の標高トップ5を紹介します:
- 富士山 – 3,776メートル
- 北岳 – 3,193メートル(10,476フィート)
- 穂高岳-3,190メートル(10,466フィート)
- 槍ヶ岳-3,180メートル(10,433フィート)
- 間ノ岳 – 3,189メートル(10,463フィート)
これらはすべて日本アルプスの一部であり、登山やハイキングの人気スポットです。
結果は、奥穂高岳の名称と、槍ヶ岳と間ノ岳の順番、間ノ岳の標高が誤りです。
かなり惜しいですが、誤りは誤りなので、少し残念ですね。
日本語に対応していない状況で、日本の情報について正しく答えるのはなかなか難しいのかもしれません。
GPT-4
Japan's top 5 mountains by elevation are as follows:
Mount Fuji (富士山) - At 3,776 meters (12,389 feet), Mount Fuji is not only Japan's tallest mountain but also an iconic symbol of the country, known for its symmetrical cone and as a sacred site.
Mount Kita (北岳) - Standing at 3,193 meters (10,476 feet), Mount Kita is the second-highest mountain in Japan and part of the Southern Alps. It's known for its beautiful alpine scenery.
Mount Oku-Hotaka (奥穂高岳) - At 3,190 meters (10,466 feet), Mount Oku-Hotaka is the third highest peak in Japan, located in the Northern Japan Alps. It's part of the Hotaka Mountain Range, offering challenging hikes and stunning vistas.
Mount Aino (間ノ岳) - With an elevation of 3,189 meters (10,463 feet), Mount Aino is the fourth highest mountain in Japan. It's also part of the Southern Alps, offering beautiful landscapes and hiking opportunities.
Mount Yari (槍ヶ岳) - Mount Yari, at 3,180 meters (10,433 feet), is the fifth highest peak in Japan. Known as the "Spear Peak" for its distinctive shape, it's a popular destination for hikers in the Northern Alps.
These mountains are not only the highest in Japan but also popular destinations for mountaineers and hikers, each offering unique natural beauty and challenges.
日本の標高上位5つの山は以下の通り:
富士山 – 標高3,776メートル(12,389フィート)の富士山は、日本で最も高い山であるだけでなく、左右対称の円錐形と聖地として知られる、日本を象徴する山でもある。
北岳 – 標高3,193メートル(10,476フィート)の北岳は、日本で2番目に高い山であり、南アルプスの一部である。美しい高山風景で知られる。
奥穂高岳 – 標高3,190メートル(10,466フィート)の奥穂高岳は日本で3番目に高い山で、北アルプスに位置する。奥穂高岳は穂高連峰の一部で、チャレンジングなハイキングと素晴らしい眺望を楽しめる。
間ノ岳 – 標高3,189メートル、日本で4番目に高い山。南アルプスの一部でもあり、美しい景観とハイキングが楽しめる。
槍ヶ岳 – 標高3,180メートル(10,433フィート)の槍ヶ岳は、日本で5番目に高い山である。その特徴的な山容から「槍ヶ岳」として知られ、北アルプスのハイカーに人気の山だ。
これらの山々は、日本で最も高い山であるだけでなく、登山家やハイカーに人気のある目的地でもあり、それぞれがユニークな自然の美しさとチャレンジを提供している。
結果は、間ノ岳の標高が1Mだけ低くなっており、非常に惜しいですが誤りです…
間ノ岳は、標高が3,189mという情報もありますが、国土地理院のデータベースには3,190mで登録されています。
Llama2 70B
Sure, here are the top 5 mountain elevations in Japan, ranked in order:
1. Mount Fuji - 3,776 meters (12,388 feet)
2. Mount Kita - 3,193 meters (10,476 feet)
3. Mount Yari - 3,141 meters (10,305 feet)
4. Mount Shirouma - 3,077 meters (10,095 feet)
5. Mount Tateyama - 3,015 meters (9,891 feet)
Note: These elevations are based on the heights of the mountains' highest peaks.
では、日本の山の標高トップ5をランキング形式で紹介します:
- 富士山 – 3,776メートル(12,388フィート)
- 北岳 – 3,193メートル(10,476フィート)
- 槍ヶ岳-3,141メートル(10,305フィート)
- 白馬岳-3,077メートル(10,095フィート)
- 立山-3,015メートル(9,891フィート)
注:これらの標高は、各山の最高峰の高さに基づいています。
結果は1,2位以外は全く違うランキングを出力しました。
3位以下は完全に誤った情報であり、残念な結果となりました。
この検証の結果は、どれも完全に正しい情報は提供してくれませんでしたが、GPT-4はほぼ正解といってもいい出力だったのでさすがです。
Mistral Largeについては、誤った情報でしたが、完全に的外れということはなく、標高や順番がわずかに違う程度でしたので、今後のアップデートで、さらに精度が向上することを期待しましょう!
今回の検証結果をまとめると、Mistral Largeの性能はGPT-4には及びませんが、今後のアップデート次第では全然追いつけるレベルだと感じました。
また、Llama2 70Bと比較すると、圧倒的にMistral Lagreの方が高性能であり、ベンチマークで示された性能は間違いではないと思います。
なお、GPT 4 Turboについて知りたい方はこちらの記事をご覧ください。
→【GPT 4 Turbo】ChatGPT最強モデル!APIの使い方や料金、できることを解説
Mistral LargeはGPT-4やGeminiの代替案になり得る存在!
Mistral Largeは、フランスのMistral AI社が公開した最新のLLMで、複雑な多言語の推論タスクに使用できる高度な推論能力を持っており、MIstralの新しいフラッグシップモデルです。
そんなMistral Largeは以下の特徴を持っています。
- 多言語対応: 英語、フランス語、スペイン語、ドイツ語、イタリア語において、ネイティブレベルに近い流暢さを実現。
- 大規模コンテキストウィンドウ: このモデルは32Kトークンのコンテキストウィンドウを持っており、これにより大文書からの情報を正確に呼び出す能力を持っています。
- 正確な関数呼び出し:適切な関数を呼び出す能力が高く、開発者やプログラマーがコーディングの効率を向上させたり、特定のプログラミング課題に対する解決策を素早く見つけることができます。
- 高度な推論能力: 複雑な問いかけに対しても、精度の高い回答を提供する能力を持っています。
ベンチマークでの性能は、GPT-4に次ぐ世界第2位となっており、これまでのMIstralのモデルはもちろん、GPT-4やGeminiなどの最新モデルと同等かそれ以上の能力をもっています。
実際に使用してみて、GPT-4とLlama2 70Bと比較した感想は、おおよそベンチマーク通りの性能は出ていると感じましたが、計算能力についてはベンチマークで示されている性能を有しているのか疑問が残ります。
また、Microsoftとも提携してAzureでも使用できるようになっており、さらには自前のチャットインターフェースも開発しているため、将来的に機能が充実すれば、GPT-4やGeminiを代替として検討できるレベルになると思います。
今後のアップデートに目が離せませんね!
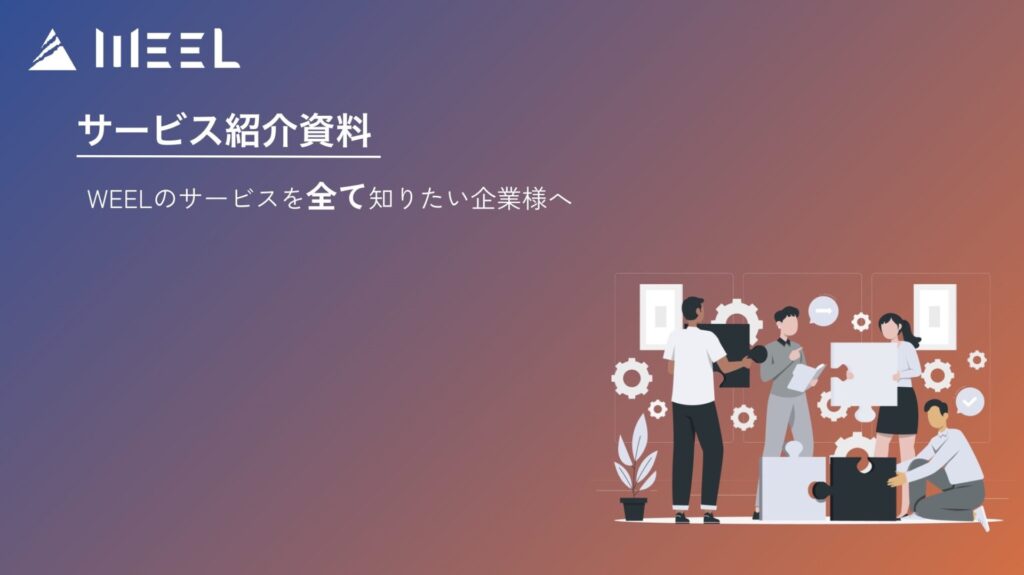
生成系AIの業務活用なら!
・生成系AIを活用したPoC開発
・生成系AIのコンサルティング
・システム間API連携
最後に
いかがだったでしょうか?
GPT-3.5 Turboの最新アップデートで、より高速かつ低コストでのAI活用が可能になりました。自社での導入・活用を検討する際に、最適なモデル選定や活用方法について、一緒に考えてみませんか?
弊社では
・マーケティングやエンジニアリングなどの専門知識を学習させたAI社員の開発
・要件定義・業務フロー作成を80%自動化できる自律型AIエージェントの開発
・生成AIとRPAを組み合わせた業務自動化ツールの開発
・社内人事業務を99%自動化できるAIツールの開発
・ハルシネーション対策AIツールの開発
・自社専用のAIチャットボットの開発
などの開発実績がございます。
まずは、「無料相談」にてご相談を承っておりますので、ご興味がある方はぜひご連絡ください。
➡︎生成AIを使った業務効率化、生成AIツールの開発について相談をしてみる。
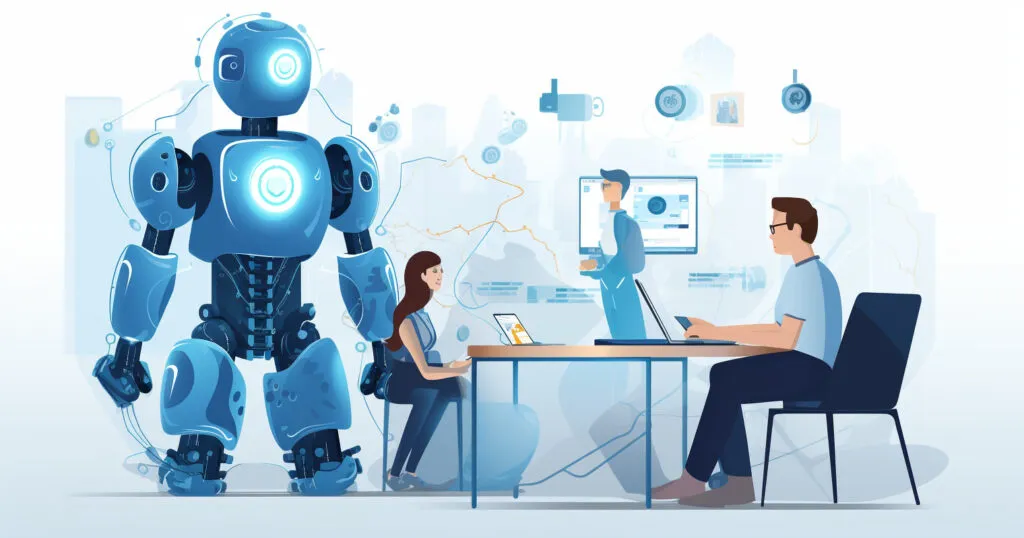
「生成AIを社内で活用したい」「生成AIの事業をやっていきたい」という方に向けて、生成AI社内セミナー・勉強会をさせていただいております。
セミナー内容や料金については、ご相談ください。
また、サービス紹介資料もご用意しておりますので、併せてご確認ください。