【DataGemma】リアルな統計データ×RAGを使ったハルシネーション対策極みLLM
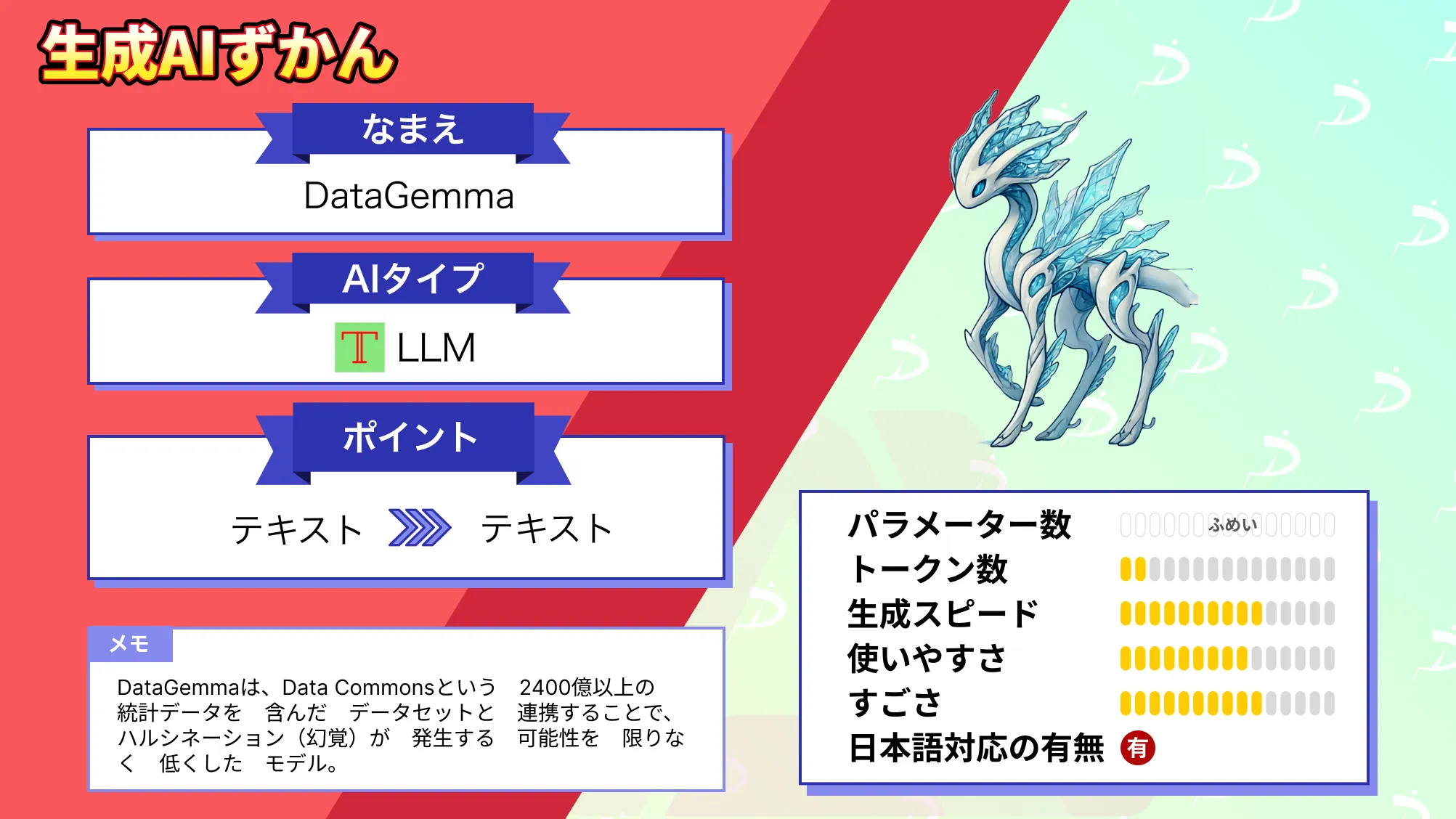
生成AIの分野における大きな課題の一つは、AIが誤った情報を生成する「ハルシネーション(幻覚)」問題です。
この問題を解決するため、Googleは2024年9月12日に大規模言語モデル(LLM)「DataGemma」を発表しました。
DataGemmaは、生成AIを信頼性の高い現実世界のデータに結びつけることで、正確性を飛躍的に向上させ、ハルシネーションのリスクを大幅に低減させています。
本記事では、この革新的な技術の仕組みや利点について詳しく解説していきます。
ぜひ最後までご覧ください!
DataGemmaの概要
Googleが公開した最新のLLMである「DataGemma」は、生成AIの課題の一つである「ハルシネーション(幻覚)」(AIが誤った情報を生成する現象)を限りなく抑えたモデルです。
DataGemmaは、LLMを現実世界の信頼性の高いデータに結びつけることで、この問題を緩和しています。
具体的には、Googleが構築した「Data Commons」という信頼性の高い統計データや公開データを集約し、2400億以上のデータポイントを保持するデータセットと連携してより正確な情報を提供します。
DataGemmaは、RIG(Retrieval-Interleaved Generation)とRAG(Retrieval-Augmented Generation)の2つの手法でData Commonsと連携して応答の正確性を強化しています。
それぞれの概要を解説します。
- RIG(Retrieval-Interleaved Generation):
- LLMが自然言語で質問を生成し、その質問をData Commonsのデータベースに対してクエリを発行する手法です。
- 例えば、「2021年のカリフォルニア州の人口は?」という質問に対して、それに基づいたデータをData Commonsから取得して、AIが回答を生成します。こうした自然言語クエリを用いることで、より正確なデータベースアクセスが可能となります。
- RAG(Retrieval-Augmented Generation):
- LLMがユーザーのクエリに関連するデータテーブルをData Commonsから取得し、それをプロンプトに統合して、最終的な回答を生成する方法です。
- 例えば、「カリフォルニア州とニューヨーク州のCO2排出量を比較して」という質問に対して、関連するデータを取得し、その情報を基にLLMが詳細な比較結果を提供します。
RIGは、モデルが応答を生成する際にData Commonsを参照して正しい情報を確認し、回答を生成することで正確性を高める。
RAGは、Data Commonsから情報を取得して、それを入力に組み込み、より正確な情報を考慮して最終的な回答を生成することで正確性を高める。
簡単に言えばこのようなアプローチでハルシネーション(幻覚)のリスクを低減しています。
以下の画像はそれぞれのアプローチの流れを図式化したものです。
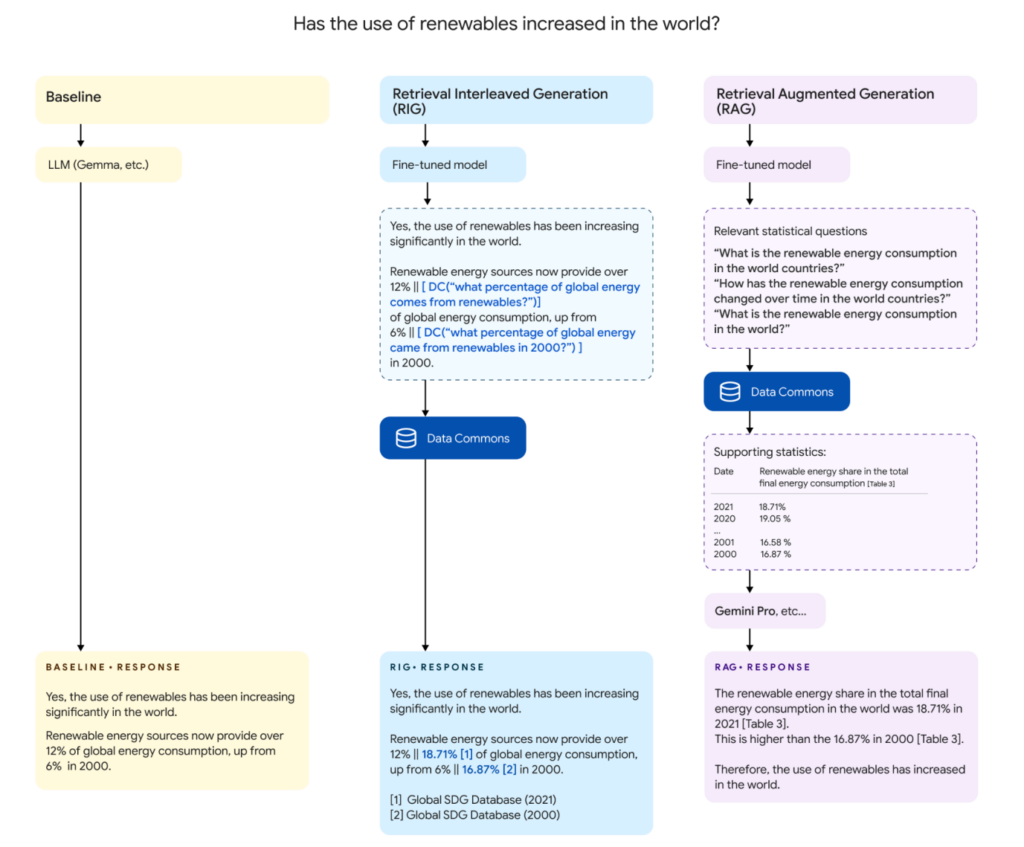
このようなアプローチによって回答の信頼性が強化された結果、既存のLLMよりもはるかに高精度な応答を生成できる世になりました。
RIGモデルでは応答の正確性がベースモデルと比較して約58%に向上し、RAGアプローチでは、98.6%のクエリで正確な統計値が生成され、推論や比較を行う際の正確性も76.4%に達しています。
ただ、Data Commonsのデータカバレッジに限界があり、カバレッジ外のクエリには不正確な結果が返されることがあります。
これは今後の拡張の課題として、さらなる改善が見込まれています。
なお、ベースモデルであるGemma 2について詳しく知りたい方は、下記の記事を合わせてご確認ください。
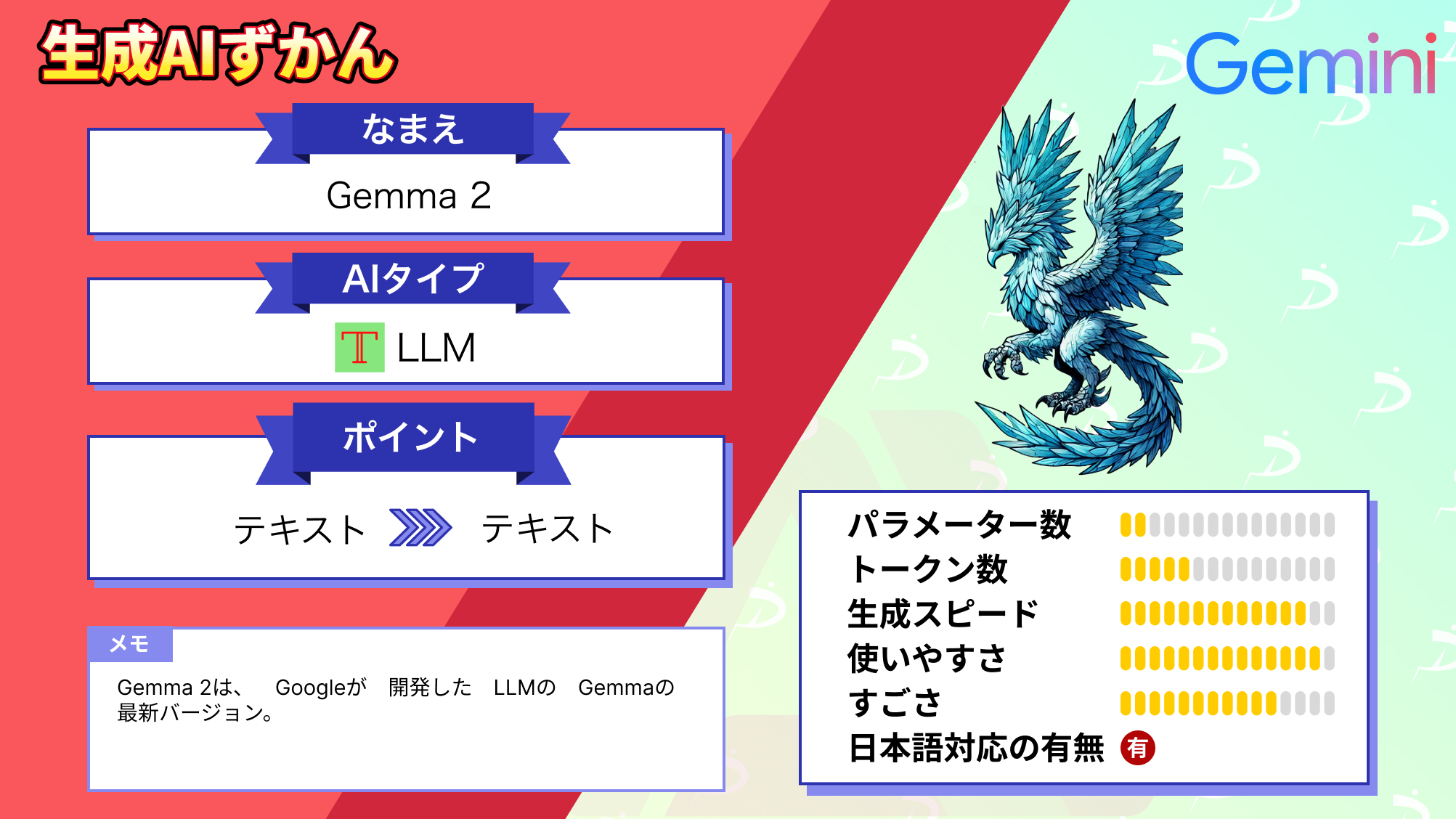
DataGemmaのライセンス
DataGemmaは、Gemmaのライセンス(Apache License 2.0)に基づいて提供されています。
ライセンス的には商用利用等も可能ですが、学術や研究目的での使用が推奨されており、現在のバージョンは商用利用や一般公開にはまだ準備が整っていないとのことです。
利用用途 | 可否 |
---|---|
商用利用 | ⭕️ |
改変 | ⭕️ |
配布 | ⭕️ |
特許使用 | ⭕️ |
私的使用 | ⭕️ |
DataGemmaの使い方
DataGemmaは、Hugging FaceやKaggleでRIGとRAGの2種類のモデルが公開されています。
それぞれの使い方を解説します。
どちらのモデルも実行前に以下のコードを使用してtransformersが最新のものになっているか確認しましょう。
pip install -U transformers accelerate
RIG
以下のコードで、モデルのロードと推論を実行します。
ソースコードはこちら
from transformers import AutoTokenizer, AutoModelForCausalLM
import torch
model_id = 'google/datagemma-rig-27b-it'
tokenizer = AutoTokenizer.from_pretrained(model_id)
model = AutoModelForCausalLM.from_pretrained(
model_id,
device_map='auto',
torch_dtype=torch.bfloat16,
)
input_text = 'What are some interesting trends in Sunnyvale spanning gender, age, race, immigration, health conditions, economic conditions, crime and education?'
inputs = tokenizer(input_text, return_tensors='pt')
outputs = model.generate(**inputs, max_new_tokens=4096)
answer = tokenizer.batch_decode(outputs[:, inputs['input_ids'].shape[1]:], skip_special_tokens=True)[0].strip()
print(answer)
出力例
出力例はこちら
Sunnyvale, CA has seen significant progress in gender, age, and educational demographics in recent years. Here's a breakdown:
**Gender:**
* **Overall:** Sunnyvale has a relatively equal gender distribution, with a slight skew towards male residents.
* **Recent Shifts:**
* **2010-2020:** The female population in Sunnyvale has been steadily increasing, reaching a [__DC__("what was the percentage of females in Sunnyvale, CA in 2020?") --> "51.6%"] majority in 2020. This is largely due to its proximity to Silicon Valley and the increasing number of women working in tech.
* **Age:** Sunnyvale has a relatively young population, with a median age of around [__DC__("what was the median age of residents in Sunnyvale, CA in 2020?") --> "35"]. This reflects the dominance of the tech industry, which tends to favor younger demographics.
* **Immigration:** Sunnyvale has a significant immigrant population, with many residents hailing from countries in Central and South America, Asia, and the Middle East.
**Age:**
* **Recent Shifts:**
* **2010-2020:** Sunnyvale has seen a slight decrease in the median age, driven by a competitive job market and a desire for better work-life balance.
* **Aging Population:** While Sunnyvale has a young population overall, it's also aging. This means the proportion of older residents is increasing, which can put pressure on healthcare and social services.
**Race & Ethnicity:**
* **Majority-Minority City:** Sunnyvale is a majority-minority city, meaning that no single racial or ethnic group constitutes a majority of the population.
* **Key Demographics:**
* **White:** [__DC__("what percentage of residents in Sunnyvale, CA are white in 2020?") --> "55.1%"]
* **Hispanic or Latino:** [__DC__("what percentage of residents in Sunnyvale, CA are hispanic or latino in 2020?") --> "30.6%"]
* **Asian:** [__DC__("what percentage of residents in Sunnyvale, CA are asian in 2020?") --> "16.8%"]
* **Black or African American:** [__DC__("what percentage of residents in Sunnyvale, CA are black or african american in 2020?") --> "3.6%"]
* **Other:** [__DC__("what percentage of residents in Sunnyvale, CA are classified as other race in 2020?") --> "0.5%"]
* **Trends & Issues:**
* **Tech Dominance:** Sunnyvale's proximity to tech jobs drives a younger, more diverse workforce.
* **Education & Income:**
A highly educated population in Sunnyvale translates to a highly skilled workforce, commanding higher salaries on average.
* **Racial & Ethnic Diversity:**
The city's commitment to diversity and inclusion is reflected in its demographics.
* **Aging Population:**
The aging population presents challenges for healthcare and social services, while a younger population puts pressure on schools and childcare.
**Economic Conditions:**
* **Low Unemployment Rate:** Sunnyvale consistently boasts a low unemployment rate, indicating a strong tech sector.
* **High Median Household Income:**
The median household income in Sunnyvale is significantly higher than the national average, driven by the high demand for skilled labor in the area.
* **Competitive Landscape:** Sunnyvale faces increasing competition for skilled workers and struggles with housing affordability and traffic congestion.
**Education Levels:**
* **High Percentage of Adults with Bachelor's Degree or Higher:**
A highly educated population is a key driver of the city's workforce and economy.
* **Emphasis on STEM Education & Innovation:**
Sunnyvale schools place a strong emphasis on STEM education and innovation, reflecting the region's tech-driven culture.
**Challenges:**
* **Gender Gap:**
The tech industry has a persistent gender gap, with women holding a smaller percentage of jobs than men.
* **Age Diversity:**
While Sunnyvale has a young population overall, it's important to ensure a balance of age groups in the workforce.
* **Immigration Policy:**
Immigration policy debates and enforcement can create uncertainty and hardship for immigrant communities.
**Resources:**
* **Sunnyvale Chamber of Commerce:** https://www.sunnyvalecoc.org/
* **City of Sunnyvale:** https://www.sunnyvale.ca.gov/
* **Stanford University:** https://www.stanford.edu/
**Note:**
These are just overarching trends. It's important to consult reliable sources like the U.S. Census Bureau and the Bureau of Labor Statistics for more detailed and up-to-date information.
RAG
以下のコードで、モデルのロードと推論を実行します。
ソースコードはこちら
from transformers import AutoTokenizer, AutoModelForCausalLM
import torch
model_id = 'google/datagemma-rag-27b-it'
tokenizer = AutoTokenizer.from_pretrained(model_id)
model = AutoModelForCausalLM.from_pretrained(
model_id,
device_map='auto',
torch_dtype=torch.bfloat16,
)
input_text = """Your role is that of a Question Generator. Given Query below, come up with a
maximum of 25 Statistical Questions that help in answering Query.
These are the only forms of Statistical Questions you can generate:
1. What is $METRIC in $PLACE?
2. What is $METRIC in $PLACE $PLACE_TYPE?
3. How has $METRIC changed over time in $PLACE $PLACE_TYPE?
where,
- $METRIC should be a metric on societal topics like demographics, economy, health,
education, environment, etc. Examples are unemployment rate and
life expectancy.
- $PLACE is the name of a place like California, World, Chennai, etc.
- $PLACE_TYPE is an immediate child type within $PLACE, like counties, states,
districts, etc.
Your response should only have questions, one per line, without any numbering
or bullet.
If you cannot come up with Statistical Questions to ask for a Query, return an
empty response.
Query: What are some interesting trends in Sunnyvale spanning gender, age, race, immigration, health conditions, economic conditions, crime and education?
Statistical Questions:"""
inputs = tokenizer(input_text, return_tensors='pt').to('cuda')
outputs = model.generate(**inputs, max_new_tokens=4096)
answer = tokenizer.batch_decode(outputs[:, inputs['input_ids'].shape[1]:], skip_special_tokens=True)[0].strip()
print(answer)
出力例
出力例はこちら
What is the population of Sunnyvale?
What is the population of Sunnyvale males?
What is the population of Sunnyvale females?
What is the population of Sunnyvale asians?
What is the population of Sunnyvale blacks?
What is the population of Sunnyvale whites?
What is the population of Sunnyvale males in their 20s?
What is the population of Sunnyvale females in their 20s?
What is the population of Sunnyvale males in their 30s?
What is the population of Sunnyvale females in their 30s?
What is the population of Sunnyvale males in their 40s?
What is the population of Sunnyvale females in their 40s?
What is the population of Sunnyvale males in their 50s?
What is the population of Sunnyvale females in their 50s?
What is the population of Sunnyvale males in their 60s?
What is the population of Sunnyvale females in their 60s?
How has the population of Sunnyvale changed over time?
How has the population of Sunnyvale males changed over time?
How has the population of Sunnyvale females changed over time?
How has the population of Sunnyvale asian people changed over time?
How has the population of Sunnyvale black people changed over time?
How has the population of Sunnyvale hispanic people changed over time?
How has the population of Sunnyvale white people changed over time?
How has the score on Sunnyvale schools changed over time?
How has the number of students enrolled in Sunnyvale schools changed over time?
How has the number of students enrolled in Sunnyvale charter schools changed over time?
How has the number of students enrolled in Sunnyvale private schools changed over time?
このように比較的簡単に実装できますが、この2つのモデルをエンドツーエンドで試すことのできるColabノートブックが公開されています。
こちらを利用したほうが、出力結果やフローが理解しやすいので、もし試される場合はこちらを利用することを推奨します。
なお、モデルとData Commonsにアクセスするためには、Hugging FaceとData CommonsのAPIキーを取得する必要があります。
また、RAGモデルのみGemini APIキーも必要になります。
Data CommonsのAPIキーは、以下のサイトにアクセス後アカウントを作成すると取得できます。
なお、RAGについて詳しく知りたい方はこちらからご覧ください。

DataGemmaを動かすのに必要な動作環境
DataGemmaを動かすのに必要な要件についての情報はありませんが、非常に大規模な入力データセットを処理できる(最大348,000トークン)ように設計されていますので、かなり高いスペックが必要です。
具体的には以下のような要件が想定されます。
- GPU: 高性能GPU(NVIDIA A100、RTX4090等)が推奨されます。推論時には少なくとも18GBのVRAMが必要です。
- RAM: この規模のモデルを効率的に運用するには、少なくとも64GB以上のRAMが推奨されています。特に複数のモデルや長いコンテキストを扱う場合に、メモリ要件が高くなります。
- ストレージ: モデルをローカルで実装する場合は、モデルのサイズやデータセットの要件により、数百GBのストレージが必要です。
また、Pythonのバージョンは3.8以上が推奨されており、TransformersやTorchなどのライブラリもPythonのバージョンに合わせたものをインストールする必要があります。
なお、GemmaをベースにしたVLMであるPailGemmaについて詳しく知りたい方は、下記の記事を合わせてご確認ください。
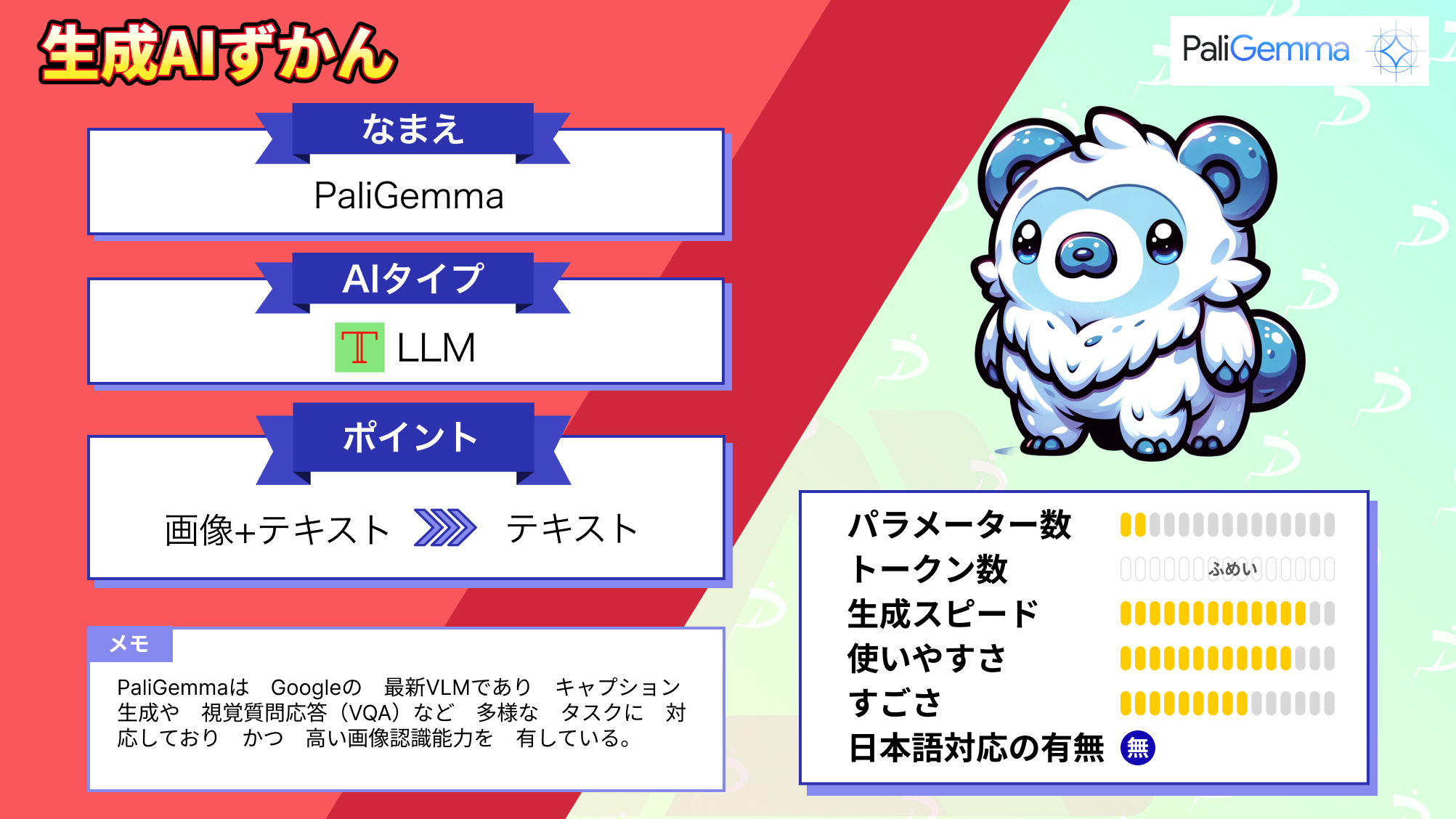
DataGemmaのRAGとRIGを使ってみた
ここからは、DataGemmaの性能を以下の3つのタスクを実行して確かめていきます。
- 統計データに関する質問
- Data Commonsに含まれていなさそうな質問
- 日本語での質問
これらのタスクをRIGとRAG両方で実行し、出力の差なども比較していきます。
早速始めていきましょう!
統計データに関する質問
以下のプロンプトを入力します。
Show me about New York City's GDP and demographics over the past 10 years.
過去10年間のニューヨーク市のGDPと人口統計を教えて
Data Commonsは主に米国の経済データや人口統計などの統計データを保持しているので、この質問には的確に答えてくれることを期待しています。
結果はこのようになりました。
RIG
RIGの結果はこちら
🙋♂️
Show me about New York City's GDP and demographics over the past 10 years.
🤖
New York City: A Tale of Two Economies
New York City presents a fascinating case study of economic diversity within a single metropolitan area. While the city's overall economy is strong, its boroughs often have vastly different economic profiles.
GDP:
Citywide: NYC's real GDP has been steadily growing, reaching a record [DC#1(|| $2.8 trillion)] in 2022.
Manhattan: As the global financial hub, Manhattan boasts the highest borough GDP, driven by strong sectors like finance, real estate, and media.
Queens: This borough has a thriving tourism industry, excellent public transportation, and a diverse culinary scene.
Brooklyn: Known for its trendy neighborhoods, artisanal coffee shops, and farm-to-table restaurants.
Queens: Predominantly residential, with a strong emphasis on education and healthcare.
Demographics:
Population: NYC's population has been aging, reaching over [DC#2(8335897 [1]* || 8.8 million)] in 2022.
Age: Older residents constitute a larger proportion of the population, with a growing number of elderly individuals and a declining birth rate.
Race/Ethnicity: NYC is a predominantly Hispanic and Asian city, with significant Black and White populations.
Immigration: NYC has historically been a major destination for immigrants from around the world.
Contrast:
Manhattan:
NYC's powerhouse borough, Manhattan, has a much younger population, with a median age of around [DC#3(38.5 yr [2]* || 35)].
Queens:
NYC's largest borough, Queens, has a median age of [DC#4(|| over 38)], with a significant aging population.
Key Differences:
Economic Focus: Manhattan is a major global financial and media center, while Queens has a more diverse economy, including tourism, media, and healthcare.
Unemployment Rate: Unemployment rates are consistently lower in Manhattan, though they have been rising in recent months.
Wage Levels: Salaries tend to be higher in Manhattan due to the high cost of living and strong demand for skilled labor.
Housing Costs: Housing costs, particularly rents, are significantly higher in Manhattan, driven by a severe housing shortage.
Access to Opportunities: Access to quality education, healthcare, and job training is crucial for social mobility and well-being.
Conclusion:
NYC is a diverse ecosystem of boroughs, each with its own unique economicStrengths and weaknesses. While Manhattan leads in finance and media, Queens excels in its multifaceted approach and strong community ties.
Bridging the Gap:
The best way to bridge the economic gap between Manhattan and Queens is through targeted investment and policy changes.
Infrastructure: Improve public transportation, roads, and sidewalks in Queens to connect it better to Manhattan's infrastructure.
Education and Job Training:
Invest in education and job training programs to empower Queens residents.
Access to Opportunities:
Create opportunities for Queens residents to access quality education, healthcare, and employment.
Community Development:
Support community development initiatives to improve the quality of life in Queens.
Data Sources:
NYC Department of Finance: https://www.nyc.gov/
NYC Department of Buildings: https://www.nyc.gov/
NYC Department of Parks: https://www.nyc.gov/
U.S. Census Bureau: https://www.census.gov/
Note:
This is a general overview, and specific data can vary depending on the source and the year of publication.
For more detailed and up-to-date information, please refer to the websites of the organizations mentioned above.
FOOTNOTES
[1] - Per www2.census.gov, value was 8335897 in 2022. See more at https://datacommons.org/explore#q=what%20is%20the%20population%20of%20New%20York%20City%3F&mode=toolformer_rig [2] - Per census.gov, value was 38.5 yr in 2022. See more at https://datacommons.org/explore#q=what%20is%20the%20median%20age%20in%20Manhattan%3F&mode=toolformer_rig
🙋♂️
ニューヨーク市の過去10年間のGDPと人口統計について教えてください。
🤖
ニューヨーク市:2つの経済の物語
ニューヨーク市は、単一の大都市圏内の経済的多様性に関する興味深いケーススタディを提供しています。市の経済全体は好調ですが、その行政区は経済のプロフィールが大幅に異なることがよくあります。
GDP:
- 全市:ニューヨーク市の実質GDPは着実に成長しており、2022年には過去最高となる2兆8000億ドルに達しました。
- マンハッタン:世界的な金融の中心地であるマンハッタンは、金融、不動産、メディアなどの好調な分野に牽引され、最も高い区GDPを誇ります。
- クイーンズ:この区は観光産業が盛んで、優れた公共交通機関と多様な料理シーンがあります。
- ブルックリン: トレンディな地区、職人の作るコーヒーショップ、農場直送の食材を使ったレストランで知られています。
- クイーンズ: 主に住宅地で、教育と医療に重点が置かれています。
人口統計:
- 人口:ニューヨーク市の人口は高齢化が進んでおり、2022年には[DC#2(8335897 [1]*||880万)]を超えると予想されています。
- 年齢:高齢者の割合が人口に占める割合が大きくなっており、高齢者の数は増加し、出生率は低下しています。
- 人種/民族:ニューヨーク市はヒスパニック系およびアジア系住民が大半を占めており、黒人と白人の人口も相当数存在します。
- 移民:ニューヨーク市は歴史的に世界中の移民の主要な目的地となってきました。
対照:
- マンハッタン:
ニューヨーク市の経済の中心地であるマンハッタン区は、人口の平均年齢がかなり若く、中央値は約[DC#3(38.5歳[2]*||35)]です。
- クイーンズ区:
ニューヨーク市最大の区であるクイーンズ区は、人口の平均年齢が[DC#4(||38歳以上)]で、高齢化が顕著です。
主な相違点:
- 経済の中心:マンハッタンは世界的な金融およびメディアの中心地ですが、クイーンズは観光、メディア、ヘルスケアなど、より多様な経済を持っています。
- 失業率:マンハッタンの失業率は常に低く、ここ数か月は上昇傾向にあります。
- 賃金水準:マンハッタンでは生活費が高く、熟練労働者の需要が高いことから、給与は高くなる傾向があります。
- 住宅費:住宅費、特に家賃はマンハッタンでは著しく高額ですが、これは深刻な住宅不足が原因です。
- 機会へのアクセス:質の高い教育、医療、職業訓練へのアクセスは、社会的な流動性と幸福にとって極めて重要です。
結論:
ニューヨーク市は、それぞれ独自の経済的強みと弱みを持つ、多様な行政区の生態系です。マンハッタンは金融とメディアでリードしていますが、クイーンズは多面的なアプローチと強固な地域社会の絆で優れています。
格差の解消:
マンハッタンとクイーンズの経済格差を埋める最善の方法は、的を絞った投資と政策の変更です。
- インフラ:クイーンズの公共交通機関、道路、歩道を改善し、マンハッタンのインフラとより良く接続します。
- 教育と職業訓練:
クイーンズの住民を力づけるために、教育と職業訓練プログラムに投資します。
- 機会へのアクセス:
クイーンズの住民が質の高い教育、医療、雇用にアクセスできる機会を創出します。
- 地域開発:
クイーンズの生活の質を向上させるための地域開発イニシアティブを支援します。
データソース:
- ニューヨーク市財務局:
- ニューヨーク市建築局:
- ニューヨーク市公園局:
- 米国国勢調査局:
注:
これは一般的な概要であり、特定のデータは情報源や発行年によって異なる場合があります。
より詳細で最新の情報については、上記の各団体のウェブサイトをご参照ください。
脚注
[1] – www2.census.govによると、2022年の値は8335897でした。詳細は をご覧ください。 [2] – census.gov によると、2022年の値は 38.5 歳でした。詳細は をご覧ください。
RAG
RAGの結果はこちら
Your role is that of a Question Generator. Give..."
... [RAG] Making DC Calls
... calling DC for table with "What is the life expectancy in New York City?"
... calling DC for table with "What is the unemployment rate in New York City?"
... calling DC for table with "How has the workforce participation rate changed over time by gender in New York City?"
... calling DC for table with "What is the age distribution in New York City?"
... calling DC for table with "What is the GDP of New York City?"
... calling DC for table with "How has the death rate changed over time in New York City?"
... calling DC for table with "How has the gender distribution changed over time in New York City?"
... calling DC for table with "What is the population of New York City?"
... calling DC for table with "How has the age distribution changed over time in New York City?"
... calling DC for table with "What is the breakdown of workforce participation rate by race in New York City?"
... calling DC for table with "How has the immigration rate changed over time in New York City?"
... calling DC for table with "What is the ethnic composition in New York City?"
... calling DC for table with "How has the workforce participation rate changed over time by race in New York City?"
... calling DC for table with "How has the unemployment rate changed over time in New York City?"
... calling DC for table with "How has the ethnic composition changed over time in New York City?"
... calling DC for table with "How has the workforce participation rate changed over time in New York City?"
... calling DC for table with "How has the birth rate changed over time in New York City?"
... calling DC for table with "What is the breakdown of gender in New York City?"
... calling DC for table with "How has the population changed over time in New York City?"
... calling DC for table with "How has the infant mortality rate changed over time in New York City?"
... calling DC for table with "What is the infant mortality rate in New York City?"
... calling DC for table with "How has the racial makeup changed over time in New York City?"
... calling DC for table with "How has the life expectancy changed over time in New York City?"
... calling DC for table with "What is the breakdown of workforce participation rate by gender in New York City?"
... calling DC for table with "What is the racial makeup in New York City?"
... [RAG] Calling UNTUNED model for final response
... calling AIStudio gemini-1.5-pro "Using statistics from the tables below, respond t..."
... [RAG] Retrying original query!
... calling AIStudio gemini-1.5-pro "Show me about New York City's GDP and demographics..."
🙋♂️
Show me about New York City's GDP and demographics over the past 10 years.
🤖
New York City's GDP & Demographics: A 10-Year Snapshot
Here's a look at New York City's GDP and key demographic trends over the past decade (approximately 2013-2023).
GDP:
Growth & Size: New York City boasts one of the largest metropolitan economies globally. Its GDP has grown consistently over the past decade, with a few notable exceptions:
Resilience: Despite the 2008 recession's lingering effects in the early part of the decade, NYC's economy rebounded strongly.
COVID-19 Impact: The pandemic caused a sharp GDP decline in 2020. However, the city has shown signs of recovery and growth since then.
Key Sectors:
Finance and Insurance: Historically dominant, this sector remains crucial but has seen some diversification in recent years.
Tech: NYC has emerged as a significant tech hub, attracting startups and tech giants alike.
Tourism: A vital industry, though impacted by the pandemic, it's showing signs of recovery.
Real Estate: A major economic driver, though fluctuations in the market can impact the overall economy.
Demographics:
Population: NYC's population has continued to grow, though at a slower pace in recent years.
Net Migration: The city experiences both significant domestic and international migration, though outmigration has increased slightly.
Natural Increase: Births outpacing deaths contribute to population growth.
Age:
Relatively Young: Compared to national averages, NYC has a younger median age.
Growing Older Adult Population: The number of residents aged 65 and older is increasing.
Race & Ethnicity: NYC remains incredibly diverse:
Largest Racial/Ethnic Groups: Non-Hispanic White, Hispanic or Latino, Black or African American, Asian.
Shifting Demographics: The city continues to see growth in its Hispanic and Asian populations.
Income & Poverty:
High Income Disparity: NYC grapples with significant income inequality, with a wide gap between the wealthy and those living in poverty.
Poverty Rate: While poverty has declined slightly, it remains a persistent challenge.
Data Sources & Considerations:
U.S. Bureau of Economic Analysis (BEA) for GDP data.
U.S. Census Bureau for demographic data.
NYC Department of City Planning for local data and analysis.
Important Notes:
This information provides a general overview. Specific data points may vary depending on the source and methodology used.
Economic and demographic trends are constantly evolving. It's essential to consult up-to-date information for the most accurate picture.
Further Exploration:
To delve deeper, explore resources from the data sources mentioned above.
Research specific neighborhoods or boroughs for a more granular understanding of local trends.
Analyze the impact of policy decisions, global events, and other factors on NYC's economy and demographics.
TABLES
Table 1: Median Age of Population in New York (unit: yr), according to census.gov
date | Median Age of Population
2022 | 37.5 2021 | 37.3 2020 | 36.9 2019 | 36.7 2018 | 36.5 2017 | 36.2 2016 | 35.9 2015 | 35.8 2014 | 35.7 2013 | 35.6 2012 | 35.5 2011 | 35.4
Table 2: Unemployment Rate in New York, according to bls.gov
date | Unemployment Rate
2024-07 | 6.1 2024-06 | 5.3 2024-05 | 4.9 2024-04 | 4.5 2024-03 | 4.5 2024-02 | 4.8 2024-01 | 4.6 2023-12 | 5.1 2023-11 | 4.9 2023-10 | 5.4 2023-09 | 5.1 2023-08 | 5.6 2023-07 | 5.3 2023-06 | 5.3 2023-05 | 5 2023-04 | 4.8 2023-03 | 5.2 2023-02 | 5.6 2023-01 | 5.5 2022-12 | 4.9 2022-11 | 5 2022-10 | 5 2022-09 | 4.6 2022-08 | 5.3 2022-07 | 5.6 2022-06 | 5.6 2022-05 | 5.4 2022-04 | 5.8 2022-03 | 6.4 2022-02 | 7.2 2022-01 | 7.9 2021-12 | 7.5 2021-11 | 7.6 2021-10 | 8.3 2021-09 | 8.7 2021-08 | 10 2021-07 | 10.5 2021-06 | 10.9 2021-05 | 10.2 2021-04 | 11.1 2021-03 | 11.4 2021-02 | 12.3 2021-01 | 12.8 2020-12 | 11.9 2020-11 | 12.4 2020-10 | 13.1 2020-09 | 14.4 2020-08 | 15 2020-07 | 17.3 2020-06 | 17.2 2020-05 | 21.6 2020-04 | 12.3 2020-03 | 5.3 2020-02 | 4.2 2020-01 | 4.1 2019-12 | 3.3 2019-11 | 3.6 2019-10 | 3.9 2019-09 | 3.6 2019-08 | 4.1 2019-07 | 4.2 2019-06 | 3.9 2019-05 | 3.8 2019-04 | 3.7 2019-03 | 4.1 2019-02 | 4.4 2019-01 | 4.8 2018-12 | 4 2018-11 | 3.9 2018-10 | 4 2018-09 | 3.9 2018-08 | 4.3 2018-07 | 4.4 2018-06 | 4.2 2018-05 | 3.6 2018-04 | 3.9 2018-03 | 4.2 2018-02 | 4.5 2018-01 | 4.6 2017-12 | 4 2017-11 | 4.1 2017-10 | 4.4 2017-09 | 4.5 2017-08 | 4.9 2017-07 | 4.9 2017-06 | 4.5 2017-05 | 4.3 2017-04 | 4.2 2017-03 | 4.4 2017-02 | 4.9 2017-01 | 5 2016-12 | 4.4 2016-11 | 4.8 2016-10 | 5.3 2016-09 | 5.3 2016-08 | 5.5 2016-07 | 5.6 2016-06 | 5.2 2016-05 | 4.7 2016-04 | 4.8 2016-03 | 5.2 2016-02 | 5.4 2016-01 | 5.5 2015-12 | 5 2015-11 | 5.1 2015-10 | 5.1 2015-09 | 5 2015-08 | 5.4 2015-07 | 5.7 2015-06 | 5.6 2015-05 | 5.6 2015-04 | 5.5 2015-03 | 5.8 2015-02 | 6.4 2015-01 | 6.7 2014-12 | 5.9 2014-11 | 6.4 2014-10 | 6.4 2014-09 | 6.4 2014-08 | 7.1 2014-07 | 7.4 2014-06 | 7.1 2014-05 | 7.1 2014-04 | 6.8 2014-03 | 7.8 2014-02 | 8.2 2014-01 | 8.2 2013-12 | 7.6 2013-11 | 8.1 2013-10 | 8.8 2013-09 | 8.7 2013-08 | 9 2013-07 | 9.2 2013-06 | 9.1 2013-05 | 8.7 2013-04 | 8.3 2013-03 | 8.8 2013-02 | 9.4 2013-01 | 9.9 2012-12 | 9 2012-11 | 8.9 2012-10 | 9.2 2012-09 | 9 2012-08 | 9.6 2012-07 | 10 2012-06 | 9.7 2012-05 | 9.3 2012-04 | 8.9 2012-03 | 9.6 2012-02 | 10 2012-01 | 9.9 2011-12 | 9.2 2011-11 | 9.2 2011-10 | 9.5 2011-09 | 9.3 2011-08 | 9.3 2011-07 | 9.4 2011-06 | 9.1 2011-05 | 8.7 2011-04 | 8.5 2011-03 | 8.7 2011-02 | 9.2 2011-01 | 9.5 2010-12 | 8.8 2010-11 | 9.3 2010-10 | 9.2 2010-09 | 9.3 2010-08 | 9.6 2010-07 | 9.7 2010-06 | 9.5 2010-05 | 9.3 2010-04 | 9.5 2010-03 | 10 2010-02 | 10.3 2010-01 | 10.5 2009-12 | 9.9 2009-11 | 9.9 2009-10 | 10.1 2009-09 | 9.9 2009-08 | 10 2009-07 | 9.8 2009-06 | 9.5 2009-05 | 8.9 2009-04 | 8.4 2009-03 | 8.6 2009-02 | 8.4 2009-01 | 7.8 2008-12 | 6.8 2008-11 | 6.3 2008-10 | 6.1 2008-09 | 5.8 2008-08 | 6 2008-07 | 5.7 2008-06 | 5.2 2008-05 | 4.9 2008-04 | 4.5 2008-03 | 5 2008-02 | 5.3 2008-01 | 5.5 2007-12 | 4.9 2007-11 | 4.8 2007-10 | 4.9 2007-09 | 4.8 2007-08 | 5.2 2007-07 | 5.5 2007-06 | 4.9 2007-05 | 4.5 2007-04 | 4.3 2007-03 | 4.4 2007-02 | 4.9 2007-01 | 5.2 2006-12 | 4.2 2006-11 | 4.5 2006-10 | 4.4 2006-09 | 4.5 2006-08 | 4.9 2006-07 | 5.4 2006-06 | 4.9 2006-05 | 4.7 2006-04 | 4.9 2006-03 | 5.2 2006-02 | 5.6 2006-01 | 5.5 2005-12 | 5.2 2005-11 | 5.8 2005-10 | 5.6 2005-09 | 5.7 2005-08 | 5.4 2005-07 | 5.8 2005-06 | 5.4 2005-05 | 5.4 2005-04 | 5.4 2005-03 | 5.5 2005-02 | 6.2 2005-01 | 6.2 2004-12 | 5.9 2004-11 | 6 2004-10 | 6.2 2004-09 | 6.4 2004-08 | 6.7 2004-07 | 7.2 2004-06 | 7.3 2004-05 | 6.8 2004-04 | 7 2004-03 | 7.8 2004-02 | 7.9 2004-01 | 8.3 2003-12 | 7.5 2003-11 | 8 2003-10 | 8.1 2003-09 | 8.2 2003-08 | 8.3 2003-07 | 8.5 2003-06 | 8.5 2003-05 | 8 2003-04 | 8 2003-03 | 8.3 2003-02 | 8.7 2003-01 | 9 2002-12 | 8.2 2002-11 | 8.3 2002-10 | 8 2002-09 | 7.5 2002-08 | 7.9 2002-07 | 8.1 2002-06 | 7.8 2002-05 | 7.7 2002-04 | 7.9 2002-03 | 8.2 2002-02 | 8.2 2002-01 | 8.3 2001-12 | 7.5 2001-11 | 7.3 2001-10 | 7 2001-09 | 6.4 2001-08 | 6.4 2001-07 | 5.7 2001-06 | 5.4 2001-05 | 5.1 2001-04 | 5.2 2001-03 | 5.5 2001-02 | 5.7 2001-01 | 5.7 2000-12 | 5.1 2000-11 | 5.4 2000-10 | 5.5 2000-09 | 5.4 2000-08 | 5.9 2000-07 | 5.9 2000-06 | 5.5 2000-05 | 5.7 2000-04 | 5.6 2000-03 | 6.3 2000-02 | 6.4 2000-01 | 6.6 1999-12 | 5.8 1999-11 | 6.2 1999-10 | 6.5 1999-09 | 6.8 1999-08 | 6.8 1999-07 | 7.3 1999-06 | 6.9 1999-05 | 6.6 1999-04 | 6.9 1999-03 | 7 1999-02 | 7.7 1999-01 | 7.6 1998-12 | 7.1 1998-11 | 7.4 1998-10 | 7.8 1998-09 | 7.6 1998-08 | 7.6 1998-07 | 7.9 1998-06 | 7.6 1998-05 | 7.5 1998-04 | 7.6 1998-03 | 8.7 1998-02 | 8.9 1998-01 | 9 1997-12 | 8 1997-11 | 8.2 1997-10 | 8.7 1997-09 | 9 1997-08 | 9.3 1997-07 | 9.6 1997-06 | 9.7 1997-05 | 9.4 1997-04 | 9.6 1997-03 | 10 1997-02 | 10.1 1997-01 | 10.2 1996-12 | 9 1996-11 | 9 1996-10 | 8.7 1996-09 | 8.4 1996-08 | 8.5 1996-07 | 9.1 1996-06 | 8.4 1996-05 | 8.7 1996-04 | 8.6 1996-03 | 9 1996-02 | 8.8 1996-01 | 8.9 1995-12 | 8 1995-11 | 8.1 1995-10 | 8.2 1995-09 | 8.3 1995-08 | 8.4 1995-07 | 8.5 1995-06 | 8.1 1995-05 | 8.1 1995-04 | 8.2 1995-03 | 8.1 1995-02 | 8.1 1995-01 | 8.3 1994-12 | 7.1 1994-11 | 7.7 1994-10 | 8.1 1994-09 | 8 1994-08 | 8.6 1994-07 | 9 1994-06 | 8.7 1994-05 | 8.6 1994-04 | 9.2 1994-03 | 9.7 1994-02 | 10.1 1994-01 | 10.5 1993-12 | 9.6 1993-11 | 9.8 1993-10 | 10.1 1993-09 | 9.6 1993-08 | 9.9 1993-07 | 10.2 1993-06 | 10.2 1993-05 | 10.1 1993-04 | 10.2 1993-03 | 10.8 1993-02 | 11.7 1993-01 | 12.2 1992-12 | 11.1 1992-11 | 11.2 1992-10 | 11.2 1992-09 | 11.4 1992-08 | 11.5 1992-07 | 11.7 1992-06 | 11.5 1992-05 | 10.8 1992-04 | 10.2 1992-03 | 10.7 1992-02 | 11.1 1992-01 | 10.8 1991-12 | 9.5 1991-11 | 9.4 1991-10 | 9.1 1991-09 | 8.8 1991-08 | 9 1991-07 | 9 1991-06 | 8.8 1991-05 | 8.8 1991-04 | 8.3 1991-03 | 8.6 1991-02 | 8.5 1991-01 | 8.3 1990-12 | 7.2 1990-11 | 7.3 1990-10 | 7.3 1990-09 | 7.1 1990-08 | 6.7 1990-07 | 6.8 1990-06 | 6.1 1990-05 | 6.4 1990-04 | 6.4 1990-03 | 6.7 1990-02 | 7 1990-01 | 7.1 1989-12 | 6.5 1989-11 | 6.8 1989-10 | 6.7 1989-09 | 6.7 1989-08 | 6.5 1989-07 | 6.6 1989-06 | 6.4 1989-05 | 6.4 1989-04 | 6.2 1989-03 | 5.8 1989-02 | 6.3 1989-01 | 6.5 1988-12 | 5.5 1988-11 | 5.5 1988-10 | 5.5 1988-09 | 5.3 1988-08 | 5.3 1988-07 | 5.2 1988-06 | 4.7 1988-05 | 4.7 1988-04 | 4.3 1988-03 | 4.8 1988-02 | 4.9 1988-01 | 5.3 1987-12 | 4.8 1987-11 | 5.4 1987-10 | 5.5 1987-09 | 5 1987-08 | 5.2 1987-07 | 5.7 1987-06 | 5.5 1987-05 | 5.4 1987-04 | 5.3 1987-03 | 6 1987-02 | 6.4 1987-01 | 6.9 1986-12 | 5.9 1986-11 | 6.4 1986-10 | 6.8 1986-09 | 6.8 1986-08 | 7 1986-07 | 7.5 1986-06 | 7.5 1986-05 | 7.8 1986-04 | 7.8 1986-03 | 8 1986-02 | 8.3 1986-01 | 7.8 1985-12 | 7.3 1985-11 | 7.5 1985-10 | 7.9 1985-09 | 7.7 1985-08 | 7.7 1985-07 | 8 1985-06 | 7.9 1985-05 | 8.1 1985-04 | 8.3 1985-03 | 8.8 1985-02 | 9.1 1985-01 | 9.3 1984-12 | 8.2 1984-11 | 8.5 1984-10 | 9.1 1984-09 | 8.9 1984-08 | 9.5 1984-07 | 9.7 1984-06 | 8.8 1984-05 | 8.3 1984-04 | 8.4 1984-03 | 8.8 1984-02 | 9.2 1984-01 | 9.4 1983-12 | 8.7 1983-11 | 8.9 1983-10 | 9.4 1983-09 | 9.5 1983-08 | 10.1 1983-07 | 10.1 1983-06 | 10 1983-05 | 9.7 1983-04 | 9.7 1983-03 | 10.4 1983-02 | 10.5 1983-01 | 10.7 1982-12 | 10 1982-11 | 10.2 1982-10 | 10 1982-09 | 9.7 1982-08 | 9.8 1982-07 | 10 1982-06 | 9.6 1982-05 | 9.3 1982-04 | 9.2 1982-03 | 9.6 1982-02 | 9.5 1982-01 | 9.7 1981-12 | 9.1 1981-11 | 9 1981-10 | 8.9 1981-09 | 8.4 1981-08 | 8.5 1981-07 | 8.9 1981-06 | 8.7 1981-05 | 8.7 1981-04 | 8.5 1981-03 | 9.2 1981-02 | 9.3 1981-01 | 9.4 1980-12 | 8.1 1980-11 | 8.5 1980-10 | 8.6 1980-09 | 8.3 1980-08 | 8.6 1980-07 | 8.9 1980-06 | 8.2 1980-05 | 8.3 1980-04 | 8.2 1980-03 | 8.2 1980-02 | 8.7 1980-01 | 8.9 1979-12 | 7.8 1979-11 | 8.3 1979-10 | 8.7 1979-09 | 8.7 1979-08 | 9.1 1979-07 | 9 1979-06 | 8.8 1979-05 | 8.2 1979-04 | 8.2 1979-03 | 8.8 1979-02 | 8.9 1979-01 | 8.9 1978-12 | 8.3 1978-11 | 8.6 1978-10 | 8.9 1978-09 | 9 1978-08 | 8.8 1978-07 | 9.3 1978-06 | 8.7 1978-05 | 8.5 1978-04 | 8.6 1978-03 | 9.2 1978-02 | 9.7 1978-01 | 10 1977-12 | 9.2 1977-11 | 10.3 1977-10 | 10.1 1977-09 | 10.3 1977-08 | 10.3 1977-07 | 10.3 1977-06 | 10.2 1977-05 | 9.4 1977-04 | 9.6 1977-03 | 10.6 1977-02 | 11 1977-01 | 11.1 1976-12 | 10.6 1976-11 | 11.1 1976-10 | 11 1976-09 | 11.1 1976-08 | 11.5 1976-07 | 11.8 1976-06 | 11.2 1976-05 | 9.9 1976-04 | 10.5 1976-03 | 10.9 1976-02 | 11.8 1976-01 | 11.9
Table 3: Population by Gender in New York, according to census.gov
date | Men | Women
2022 | 4148719 | 4473748 2021 | 4192240 | 4543807 2020 | 3997959 | 4381593 2019 | 4015982 | 4403334 2018 | 4025097 | 4418616 2017 | 4079907 | 4480165 2016 | 4033736 | 4428225 2015 | 4015044 | 4411699 2014 | 3976564 | 4378325 2013 | 3935848 | 4333151 2012 | 3897434 | 4301787 2011 | 3860829 | 4268151
Table 4: Age Distribution in New York (2021 to 2022), according to data.census.gov
variable | New York City
Population: 1 - 4 Years | 438992 Population Aged 5 to 17 | 1252093 Population Aged 18 to 24 Years | 723297 Population Aged 25 to 34 Years | 1480349 Population Aged 35 to 44 | 1192645 Population Aged 45 to 54 | 1072881 Population: 55 - 64 Years | 1045096 Population Aged 65 to 74 | 763022 Population Aged 75 and Up | 572617
Table 5: Revenue by Industry Type in New York (2002 to 2017) (unit: USD), according to census.gov
variable | New York City
Revenue of Establishments with Payroll: Accommodation And Food Services | 38941921000 Revenue of Establishments with Payroll: Administrative, Support, Waste Management | 44926117000 Revenue of Establishments with Payroll: Arts, Entertainment, Recreation | 20763647000 Revenue of Establishments with Payroll: Educational Services | 4001450000 Revenue of Establishments with Payroll: Health Care and Social Assistance | 76165547000 Revenue of Establishments with Payroll: Wholesale Trade | 173539688000 Revenue of Establishments with Payroll: Other Services | 35687873000 Revenue of Establishments with Payroll: Professional, Scientific, Technical Services | 126758994000 Revenue of Establishments with Payroll: Real Estate, Rental, Leasing | 53248670000 Sales of Establishments With Payroll: Miscellaneous Store Retailers | 8811145000 Revenue of Establishment With Payroll: Couriers And Messengers | 2427488000 Sales of Establishments With Payroll: Sports, Hobby, Music Instrument, Book Stores | 1573163000 Sales of Establishments With Payroll: Nonstore Retailers | 24948234000 Sales of Establishments With Payroll: General Merchandise Stores | 6545269000 Revenue of Establishments With Payroll: Transportation And Warehousing | 29800369000 Shipments or Receipts of Establishments With Payroll: Chemical Manufacturing | 1565004000 Shipments or Receipts of Establishments With Payroll: Printing And Related Support Activities | 1000787000 Shipments or Receipts of Establishments With Payroll: Furniture And Related Product Manufacturing | 621631000 Shipments or Receipts of Establishment With Payroll: Miscellaneous Manufacturing | 2638361000 Shipments or Receipts of Establishments With Payroll: Paper Manufacturing | 364286000 Shipments or Receipts of Establishments With Payroll: Computer And Electronic Product Manufacturing | 586768000 Shipments or Receipts of Establishment With Payroll: Machinery Manufacturing | 410618000 Sales of Establishments With Payroll: Retail Trade | 116309814000 Shipments or Receipts of Establishments With Payroll: Plastics And Rubber Products Manufacturing | 513183000 Shipments or Receipts of Establishments With Payroll: Transportation Equipment Manufacturing | 374896000 Shipments or Receipts of Establishments With Payroll: Fabricated Metal Product Manufacturing | 922945000 Shipments or Receipts of Establishments With Payroll: Wood Product Manufacturing | 126803000 Shipments or Receipts of Establishments With Payroll: Primary Metal Manufacturing | 311422000 Revenue of Establishments With Payroll: Warehousing And Storage | 186333000 Shipments or Receipts of Establishments With Payroll: Electrical Equipment And Appliance Mfg. | 258506000 Shipments or Receipts of Establishments With Payroll: Nonmetallic Mineral Product Manufacturing | 400546000
Table 8: Population in New York, according to www2.census.gov
date | Population
2022 | 8335897 2021 | 8467513 2020 | 8772978 2019 | 8342925 2018 | 8396614 2017 | 8437695 2016 | 8468954 2015 | 8463157 2014 | 8433867 2013 | 8396207 2012 | 8346715 2011 | 8272864 2010 | 8190105 2009 | 7991063 2008 | 7945783 2007 | 7908735 2006 | 7904335 2005 | 7939571 2004 | 7984440 2003 | 8023287 2002 | 8041950 2001 | 8043949 2000 | 8015209 1999 | 7428162 1998 | 7404140 1997 | 7382901 1996 | 7360622 1995 | 7349560 1994 | 7341300 1993 | 7304895 1992 | 7304481 1991 | 7322155 1990 | 7322564
Table 9: Age Distribution in New York, according to census.gov
date | Population Aged 65 to 74 | Population: 55 - 64 Years | Population Aged 75 and Up | Population Aged 25 to 34 Years | Population: 1 - 4 Years | Population Aged 35 to 44 | Population Aged 5 to 17 | Population Aged 45 to 54 | Population Aged 18 to 24 Years
2022 | 763022 | 1045096 | 572617 | 1480349 | | 1192645 | 1252093 | 1072881 | 723297 2021 | 757463 | 1056332 | 560741 | 1515476 | 438992 | 1206501 | 1278944 | 1095182 | 721971 2020 | 702799 | 994445 | 549714 | 1492460 | 431830 | 1142869 | 1202710 | 1051139 | 708657 2019 | 684589 | 992061 | 537678 | 1501492 | 440536 | 1149333 | 1209576 | 1070062 | 729554 2018 | 664818 | 986482 | 524543 | 1504279 | 445853 | 1156416 | 1214702 | 1086960 | 753644 2017 | 650639 | 985972 | 517629 | 1527619 | 452350 | 1177418 | 1234286 | 1110671 | 795480 2016 | 612814 | 968196 | 486516 | 1497751 | 448348 | 1174805 | 1238478 | 1111690 | 816328 2015 | 592098 | 956154 | 479607 | 1480634 | 448253 | 1175417 | 1235825 | 1117414 | 833783 2014 | 571836 | 937534 | 474835 | 1454597 | 439796 | 1169445 | 1239052 | 1115028 | 846270 2013 | 553911 | 915801 | 469482 | 1428313 | 431234 | 1162739 | 1240861 | 1110653 | 851127 2012 | 538264 | 894917 | 464608 | 1403954 | 422319 | 1161803 | 1247865 | 1104581 | 856185 2011 | 523362 | 872966 | 461279 | 1382334 | 416303 | 1167170 | 1253845 | 1098769 | 847777 2010 | | | | | 411873 | | | |
Table 10: Population in the Labor Force in New York, according to bls.gov
date | Population in the Labor Force
2024-07 | 4225523 2024-06 | 4213614 2024-05 | 4172145 2024-04 | 4181619 2024-03 | 4208334 2024-02 | 4191426 2024-01 | 4135103 2023-12 | 4130506 2023-11 | 4155081 2023-10 | 4156808 2023-09 | 4128126 2023-08 | 4165918 2023-07 | 4166603 2023-06 | 4157868 2023-05 | 4148420 2023-04 | 4134828 2023-03 | 4179229 2023-02 | 4171938 2023-01 | 4127193 2022-12 | 4105694 2022-11 | 4098987 2022-10 | 4104925 2022-09 | 4065445 2022-08 | 4089452 2022-07 | 4098472 2022-06 | 4080986 2022-05 | 4078494 2022-04 | 4076730 2022-03 | 4117809 2022-02 | 4123066 2022-01 | 4117696 2021-12 | 4081274 2021-11 | 4083122 2021-10 | 4093649 2021-09 | 4067502 2021-08 | 4110994 2021-07 | 4125057 2021-06 | 4107336 2021-05 | 4052442 2021-04 | 4098894 2021-03 | 4109957 2021-02 | 4093322 2021-01 | 4059848 2020-12 | 4044729 2020-11 | 4058389 2020-10 | 4070896 2020-09 | 4040546 2020-08 | 4077301 2020-07 | 4090644 2020-06 | 4046714 2020-05 | 3882339 2020-04 | 3703849 2020-03 | 4291727 2020-02 | 4318409 2020-01 | 4282195 2019-12 | 4249887 2019-11 | 4250547 2019-10 | 4281115 2019-09 | 4251148 2019-08 | 4260491 2019-07 | 4270373 2019-06 | 4254496 2019-05 | 4231646 2019-04 | 4249999 2019-03 | 4287348 2019-02 | 4286966 2019-01 | 4258444 2018-12 | 4261564 2018-11 | 4240258 2018-10 | 4268433 2018-09 | 4227069 2018-08 | 4246840 2018-07 | 4271649 2018-06 | 4272100 2018-05 | 4240020 2018-04 | 4263492 2018-03 | 4296528 2018-02 | 4314218 2018-01 | 4268675 2017-12 | 4258690 2017-11 | 4275451 2017-10 | 4300745 2017-09 | 4315408 2017-08 | 4299717 2017-07 | 4342922 2017-06 | 4300703 2017-05 | 4280591 2017-04 | 4292091 2017-03 | 4329153 2017-02 | 4317178 2017-01 | 4274446 2016-12 | 4083277 2016-11 | 4083100 2016-10 | 4097777 2016-09 | 4089711 2016-08 | 4099613 2016-07 | 4107522 2016-06 | 4072837 2016-05 | 4048212 2016-04 | 4064296 2016-03 | 4114382 2016-02 | 4114599 2016-01 | 4075349 2015-12 | 4074367 2015-11 | 4066094 2015-10 | 4073342 2015-09 | 4040138 2015-08 | 4083965 2015-07 | 4108503 2015-06 | 4087605 2015-05 | 4097712 2015-04 | 4095893 2015-03 | 4113967 2015-02 | 4125207 2015-01 | 4101838 2014-12 | 4082915 2014-11 | 4086151 2014-10 | 4108693 2014-09 | 4066890 2014-08 | 4094263 2014-07 | 4112690 2014-06 | 4102543 2014-05 | 4075161 2014-04 | 4060289 2014-03 | 4113307 2014-02 | 4111823 2014-01 | 4078202 2013-12 | 4050764 2013-11 | 4065804 2013-10 | 4077369 2013-09 | 4064725 2013-08 | 4092475 2013-07 | 4113334 2013-06 | 4077096 2013-05 | 4052254 2013-04 | 4038537 2013-03 | 4037902 2013-02 | 4060225 2013-01 | 4052335 2012-12 | 4046401 2012-11 | 4018436 2012-10 | 4047864 2012-09 | 4018492 2012-08 | 4028346 2012-07 | 4056621 2012-06 | 4025481 2012-05 | 4004064 2012-04 | 3987795 2012-03 | 4020958 2012-02 | 4018696 2012-01 | 3979300 2011-12 | 3987622 2011-11 | 3979070 2011-10 | 3996428 2011-09 | 3976121 2011-08 | 4000563 2011-07 | 3985503 2011-06 | 3954743 2011-05 | 3952800 2011-04 | 3951588 2011-03 | 3973757 2011-02 | 3973426 2011-01 | 3970651 2010-12 | 3950896 2010-11 | 3947260 2010-10 | 3963088 2010-09 | 3962730 2010-08 | 4000557 2010-07 | 4000835 2010-06 | 3982079 2010-05 | 3974782 2010-04 | 4007358 2010-03 | 3999416 2010-02 | 4004226 2010-01 | 3973888 2009-12 | 3956410 2009-11 | 3967110 2009-10 | 3981035 2009-09 | 3948585 2009-08 | 3999983 2009-07 | 4025128 2009-06 | 3972401 2009-05 | 3935372 2009-04 | 3939632 2009-03 | 3959032 2009-02 | 4026041 2009-01 | 3993241 2008-12 | 3987359 2008-11 | 3982757 2008-10 | 3991440 2008-09 | 3958818 2008-08 | 3977418 2008-07 | 3975574 2008-06 | 3939528 2008-05 | 3898530 2008-04 | 3903762 2008-03 | 3907982 2008-02 | 3923817 2008-01 | 3911556 2007-12 | 3890956 2007-11 | 3892200 2007-10 | 3874656 2007-09 | 3859126 2007-08 | 3874120 2007-07 | 3896542 2007-06 | 3842934 2007-05 | 3817662 2007-04 | 3812072 2007-03 | 3845965 2007-02 | 3852194 2007-01 | 3840195 2006-12 | 3829449 2006-11 | 3816596 2006-10 | 3829322 2006-09 | 3797593 2006-08 | 3836217 2006-07 | 3853958 2006-06 | 3823688 2006-05 | 3812857 2006-04 | 3810358 2006-03 | 3835690 2006-02 | 3851443 2006-01 | 3822015 2005-12 | 3816760 2005-11 | 3815103 2005-10 | 3833662 2005-09 | 3812408 2005-08 | 3818044 2005-07 | 3834043 2005-06 | 3785217 2005-05 | 3770616 2005-04 | 3773851 2005-03 | 3779662 2005-02 | 3792854 2005-01 | 3767538 2004-12 | 3777744 2004-11 | 3789654 2004-10 | 3779489 2004-09 | 3758685 2004-08 | 3789991 2004-07 | 3820342 2004-06 | 3756104 2004-05 | 3723803 2004-04 | 3723599 2004-03 | 3760050 2004-02 | 3720632 2004-01 | 3719478 2003-12 | 3690056 2003-11 | 3717716 2003-10 | 3697617 2003-09 | 3680070 2003-08 | 3707436 2003-07 | 3718424 2003-06 | 3705043 2003-05 | 3680147 2003-04 | 3708963 2003-03 | 3713445 2003-02 | 3740174 2003-01 | 3729686 2002-12 | 3721973 2002-11 | 3709337 2002-10 | 3727666 2002-09 | 3710696 2002-08 | 3737915 2002-07 | 3737712 2002-06 | 3721104 2002-05 | 3705068 2002-04 | 3712494 2002-03 | 3730575 2002-02 | 3735713 2002-01 | 3697121 2001-12 | 3685708 2001-11 | 3674531 2001-10 | 3666879 2001-09 | 3624268 2001-08 | 3643512 2001-07 | 3665489 2001-06 | 3604546 2001-05 | 3606826 2001-04 | 3626022 2001-03 | 3673315 2001-02 | 3675505 2001-01 | 3658619 2000-12 | 3664418 2000-11 | 3649337 2000-10 | 3644383 2000-09 | 3628523 2000-08 | 3666868 2000-07 | 3675725 2000-06 | 3662277 2000-05 | 3644320 2000-04 | 3662782 2000-03 | 3694899 2000-02 | 3679052 2000-01 | 3639408 1999-12 | 3616905 1999-11 | 3605532 1999-10 | 3612945 1999-09 | 3596431 1999-08 | 3616880 1999-07 | 3647599 1999-06 | 3588723 1999-05 | 3558387 1999-04 | 3556835 1999-03 | 3578429 1999-02 | 3603157 1999-01 | 3582517 1998-12 | 3572209 1998-11 | 3570352 1998-10 | 3571223 1998-09 | 3547596 1998-08 | 3561436 1998-07 | 3598183 1998-06 | 3546186 1998-05 | 3529850 1998-04 | 3528187 1998-03 | 3586741 1998-02 | 3583879 1998-01 | 3556369 1997-12 | 3541167 1997-11 | 3531397 1997-10 | 3539712 1997-09 | 3528752 1997-08 | 3558298 1997-07 | 3573965 1997-06 | 3516642 1997-05 | 3498110 1997-04 | 3490884 1997-03 | 3527693 1997-02 | 3502006 1997-01 | 3477670 1996-12 | 3452935 1996-11 | 3446649 1996-10 | 3434693 1996-09 | 3398885 1996-08 | 3418570 1996-07 | 3450884 1996-06 | 3374410 1996-05 | 3374680 1996-04 | 3364239 1996-03 | 3387410 1996-02 | 3348538 1996-01 | 3318556 1995-12 | 3311863 1995-11 | 3314292 1995-10 | 3323938 1995-09 | 3308864 1995-08 | 3332486 1995-07 | 3344010 1995-06 | 3273065 1995-05 | 3264690 1995-04 | 3279319 1995-03 | 3287481 1995-02 | 3276151 1995-01 | 3260651 1994-12 | 3262661 1994-11 | 3274121 1994-10 | 3286425 1994-09 | 3247106 1994-08 | 3303602 1994-07 | 3321121 1994-06 | 3280167 1994-05 | 3269058 1994-04 | 3274638 1994-03 | 3313229 1994-02 | 3307880 1994-01 | 3300320 1993-12 | 3280611 1993-11 | 3281777 1993-10 | 3300343 1993-09 | 3263617 1993-08 | 3322199 1993-07 | 3356304 1993-06 | 3309785 1993-05 | 3287344 1993-04 | 3275800 1993-03 | 3325678 1993-02 | 3344850 1993-01 | 3323677 1992-12 | 3333323 1992-11 | 3317206 1992-10 | 3307986 1992-09 | 3324662 1992-08 | 3365734 1992-07 | 3385158 1992-06 | 3339151 1992-05 | 3287661 1992-04 | 3289088 1992-03 | 3309258 1992-02 | 3296617 1992-01 | 3282260 1991-12 | 3270284 1991-11 | 3272729 1991-10 | 3279459 1991-09 | 3268995 1991-08 | 3288707 1991-07 | 3324483 1991-06 | 3289762 1991-05 | 3266723 1991-04 | 3276511 1991-03 | 3299027 1991-02 | 3277984 1991-01 | 3286256 1990-12 | 3289433 1990-11 | 3298105 1990-10 | 3324697 1990-09 | 3315431 1990-08 | 3355689 1990-07 | 3378104 1990-06 | 3369202 1990-05 | 3346156 1990-04 | 3330533 1990-03 | 3359836 1990-02 | 3366993 1990-01 | 3357022 1989-12 | 3346939 1989-11 | 3358150 1989-10 | 3344489 1989-09 | 3319976 1989-08 | 3357679 1989-07 | 3384939 1989-06 | 3343663 1989-05 | 3306282 1989-04 | 3315064 1989-03 | 3305911 1989-02 | 3308911 1989-01 | 3294432 1988-12 | 3271776 1988-11 | 3268349 1988-10 | 3262478 1988-09 | 3223449 1988-08 | 3266082 1988-07 | 3273307 1988-06 | 3232682 1988-05 | 3187203 1988-04 | 3200061 1988-03 | 3228142 1988-02 | 3225213 1988-01 | 3222482 1987-12 | 3229700 1987-11 | 3252704 1987-10 | 3245322 1987-09 | 3210148 1987-08 | 3284724 1987-07 | 3284243 1987-06 | 3229786 1987-05 | 3217799 1987-04 | 3211800 1987-03 | 3249221 1987-02 | 3225550 1987-01 | 3227758 1986-12 | 3221434 1986-11 | 3227572 1986-10 | 3229217 1986-09 | 3209742 1986-08 | 3254817 1986-07 | 3286062 1986-06 | 3245484 1986-05 | 3224994 1986-04 | 3224922 1986-03 | 3265003 1986-02 | 3240989 1986-01 | 3244140 1985-12 | 3242264 1985-11 | 3252193 1985-10 | 3255362 1985-09 | 3234536 1985-08 | 3267152 1985-07 | 3283007 1985-06 | 3217107 1985-05 | 3193592 1985-04 | 3182639 1985-03 | 3142780 1985-02 | 3122809 1985-01 | 3125775 1984-12 | 3102068 1984-11 | 3086952 1984-10 | 3099393 1984-09 | 3081254 1984-08 | 3135504 1984-07 | 3170653 1984-06 | 3092106 1984-05 | 3076031 1984-04 | 3054251 1984-03 | 3087109 1984-02 | 3077957 1984-01 | 3067464 1983-12 | 3070960 1983-11 | 3075917 1983-10 | 3068028 1983-09 | 3086309 1983-08 | 3130098 1983-07 | 3126322 1983-06 | 3083234 1983-05 | 3010156 1983-04 | 3028331 1983-03 | 3060446 1983-02 | 3046925 1983-01 | 3056984 1982-12 | 3054449 1982-11 | 3074542 1982-10 | 3083492 1982-09 | 3070026 1982-08 | 3108534 1982-07 | 3144793 1982-06 | 3092859 1982-05 | 3090069 1982-04 | 3063222 1982-03 | 3102160 1982-02 | 3079154 1982-01 | 3075723 1981-12 | 3072958 1981-11 | 3089340 1981-10 | 3090375 1981-09 | 3056736 1981-08 | 3103943 1981-07 | 3138026 1981-06 | 3091343 1981-05 | 3084054 1981-04 | 3089944 1981-03 | 3101651 1981-02 | 3090688 1981-01 | 3073969 1980-12 | 3051306 1980-11 | 3053721 1980-10 | 3060997 1980-09 | 3032016 1980-08 | 3092739 1980-07 | 3119955 1980-06 | 3049472 1980-05 | 3029423 1980-04 | 3036569 1980-03 | 3058373 1980-02 | 3078581 1980-01 | 3061114 1979-12 | 3064619 1979-11 | 3062418 1979-10 | 3070285 1979-09 | 3061768 1979-08 | 3106370 1979-07 | 3115554 1979-06 | 3056420 1979-05 | 3008871 1979-04 | 3039290 1979-03 | 3076519 1979-02 | 3069182 1979-01 | 3053354 1978-12 | 3059046 1978-11 | 3064808 1978-10 | 3065572 1978-09 | 3051863 1978-08 | 3083327 1978-07 | 3105802 1978-06 | 3065022 1978-05 | 3020706 1978-04 | 3040893 1978-03 | 3048503 1978-02 | 3043953 1978-01 | 3045581 1977-12 | 3051051 1977-11 | 3064434 1977-10 | 3055759 1977-09 | 3029858 1977-08 | 3095203 1977-07 | 3093248 1977-06 | 3069799 1977-05 | 3013828 1977-04 | 3046346 1977-03 | 3072754 1977-02 | 3080802 1977-01 | 3056764 1976-12 | 3075767 1976-11 | 3085568 1976-10 | 3068813 1976-09 | 3056267 1976-08 | 3124702 1976-07 | 3145241 1976-06 | 3084330 1976-05 | 3016803 1976-04 | 3043937 1976-03 | 3062814 1976-02 | 3064176 1976-01 | 3061214
Table 11: Population by Nativity in New York (unit: %), according to census.gov
date | Population: Native | Population: Foreign Born
2022 | 59.7777 | 37.5862 2021 | 59.5033 | 37.4308 2020 | 54.799 | 34.7918 2019 | 57.4053 | 37.1639 2018 | 56.9381 | 37.2446 2017 | 57.3187 | 37.6892 2016 | 56.3981 | 37.1488 2015 | 56.1116 | 37.0804 2014 | 55.9706 | 36.8008 2013 | 55.8445 | 36.4335 2012 | 55.8568 | 36.2282 2011 | 55.9853 | 36.1401
Table 13: Monoracial Breakdown in New York, according to census.gov
date | Population Asian Alone | Hispanic Population | Population Identifying as Some Other Race Alone | Population American Indian or Alaska Native Alone | Population of Native Hawaiian or Other Pacific Islander Alone | Black Monoracial Population | White Monoracial Population
2022 | 1247994 | 2503005 | 1325866 | 52828 | 5431 | 1992200 | 3230647 2021 | 1244510 | 2521022 | 1301785 | 45784 | 5600 | 2042560 | 3474805 2020 | 1197261 | 2423869 | 1209179 | 36884 | 5283 | 1996121 | 3463286 2019 | 1186608 | 2447862 | 1241614 | 36250 | 4802 | 2046877 | 3597217 2018 | 1177700 | 2457137 | 1277050 | 36075 | 4339 | 2049418 | 3603057 2017 | 1198334 | 2491496 | 1294497 | 34487 | 4358 | 2081507 | 3661800 2016 | 1159883 | 2457945 | 1277265 | 33388 | 3995 | 2064927 | 3644982 2015 | 1139294 | 2437297 | 1269789 | 32790 | 3389 | 2065597 | 3648214 2014 | 1106989 | 2407983 | 1231976 | 32887 | 3572 | 2067708 | 3646761 2013 | 1077554 | 2371116 | 1189355 | 32421 | 4403 | 2057226 | 3659155 2012 | 1053649 | 2343458 | 1169421 | 30743 | 3866 | 2059279 | 3646181 2011 | 1032214 | 2310163 | 1200833 | 30011 | 3909 | 2046365 | 3598277
Table 15: Median Age by Gender in New York (unit: yr), according to census.gov
date | Men's Median Age | Women's Median Age
2022 | 36.2 | 38.9 2021 | 36 | 38.6 2020 | 35.6 | 38.4 2019 | 35.4 | 38.1 2018 | 35.2 | 37.8 2017 | 34.9 | 37.5 2016 | 34.6 | 37.2 2015 | 34.5 | 37.1 2014 | 34.4 | 37 2013 | 34.2 | 37 2012 | 34.1 | 36.9 2011 | 34 | 36.9
Table 17: Population: 16-50 Years, Birth in the Past 12 Months, Female (As Fraction of Count Person 16 to 50 Years Female) in New York, according to data.census.gov
date | Population: 16 - 50 Years, Birth in The Past 12 Months, Female (As Fraction of Count Person 16 To 50 Years Female)
2022 | 46 2021 | 45 2020 | 47 2019 | 47 2018 | 47 2017 | 48 2016 | 48 2015 | 49 2014 | 51 2013 | 51 2012 | 53 2011 | 53 2010 | 53
Table 25: Monoracial Breakdown in New York (2022), according to census.gov
variable | New York City
White Monoracial Population | 3230647 Black Monoracial Population | 1992200 Hispanic Population | 2503005 Population Asian Alone | 1247994 Population American Indian or Alaska Native Alone | 52828 Population of Native Hawaiian or Other Pacific Islander Alone | 5431 Population Identifying as Some Other Race Alone | 1325866
あなたの役割は質問作成者です。…
[RAG] DCに電話する…
「ニューヨーク市の平均余命は?」の表についてDCに電話する…
「ニューヨーク市の失業率は?」の表についてDCに電話する…
「ニューヨーク市の労働力参加率は男女別でどのように変化したか?」の表についてDCに電話する…
「ニューヨーク市の年齢分布は?」の表についてDCに電話する
… 「ニューヨーク市のGDPはいくらですか?」という表をDCに問い合わせ…
「ニューヨーク市の死亡率はどのように変化しましたか?」という表をDCに問い合わせ…
「ニューヨーク市の男女比はどのように変化しましたか?」という表をDCに問い合わせ…
「ニューヨーク市の人口はいくらですか?」という表をDCに問い合わせ…
「ニューヨーク市の年齢別人口比はどのように変化しましたか?」という表をDCに問い合わせ
… 「ニューヨーク市の人種別労働力率の内訳は?」という表をDCに問い合わせ…
「ニューヨーク市の移民率はどのように変化したか?」という表をDCに問い合わせ…
「ニューヨーク市の民族構成は?」という表をDCに問い合わせ…
「ニューヨーク市の人種別労働力率はどのように変化したか?」という表をDCに問い合わせ
… 「ニューヨーク市の失業率はどのように推移しているか」の表をDCに問い合わせ…
「ニューヨーク市の民族構成はどのように推移しているか」の表をDCに問い合わせ…
「ニューヨーク市の労働力率はどのように推移しているか」の表をDCに問い合わせ…
「ニューヨーク市の出生率はどのように推移しているか」の表をDCに問い合わせ
… 「ニューヨーク市の性別内訳は?」という表をDCに問い合わせ…
「ニューヨーク市の人口はどのように変化したか?」という表をDCに問い合わせ…
「ニューヨーク市の乳児死亡率はどのように変化したか?」という表をDCに問い合わせ…
「ニューヨーク市の乳児死亡率は?」という表をDCに問い合わせ
… 「ニューヨーク市の人種構成はどのように変化したか」という表をDCに問い合わせます。…
「ニューヨーク市の平均余命はどのように変化したか」という表をDCに問い合わせます。…
「ニューヨーク市の労働力率の内訳は男女別でどうなっているか」という表をDCに問い合わせます。…
「ニューヨーク市の人種構成はどのようになっているか」という表をDCに問い合わせます。…
[RAG] UNTUNEDモデルを最終応答のために呼び出します
… calling AIStudio gemini-1.5-pro 「Using statistics from the tables below, respond t…」…
[RAG] Retrying original query!…
calling AIStudio gemini-1.5-pro 「Show me about New York City’s GDP and demographics…」
🙋♂️
ニューヨーク市のGDPと人口統計について、過去10年間分を表示してください。
🤖
ニューヨーク市のGDPと人口統計:10年間の概観
ここ10年間(2013年~2023年頃)のニューヨーク市のGDPと主要な人口統計の傾向を見てみましょう。
GDP:
- 成長と規模:ニューヨーク市は世界でも最大級の都市経済を誇ります。GDPは過去10年間、いくつかの例外を除いて一貫して成長しています。
- 回復力:2008年の不況の影響が10年代前半まで残りましたが、ニューヨーク市の経済は力強く回復しました。
- COVID-19の影響:パンデミックにより2020年のGDPは急激に減少しました。しかし、それ以降、市は回復と成長の兆しを見せています。
- 主要産業:
- 金融および保険:歴史的に優勢であったこの産業は依然として重要ですが、近年は多様化が進んでいます。
- テクノロジー:ニューヨーク市は重要なテクノロジーの拠点として台頭し、スタートアップ企業や大手テクノロジー企業を惹きつけています。
- 観光:重要な産業ですが、パンデミックの影響を受けました。しかし、回復の兆しを見せています。
- 不動産:主要な経済推進力ですが、市場の変動が経済全体に影響を与える可能性があります。
人口統計:
- 人口:ニューヨーク市の人口は増加を続けていますが、近年はペースが鈍化しています。
- 純移住:国内および国外からの移住がともに多い都市ですが、国外への移住がやや増加しています。
- 自然増加:出生数が死亡数を上回っており、人口増加に貢献しています。
- 年齢:
- 比較的若い:全国平均と比較すると、ニューヨーク市の中央値年齢は低くなっています。
- 高齢化する成人人口:65歳以上の住民数が増加しています。
- 人種および民族:ニューヨーク市は依然として非常に多様です。
- 最大の民族/人種グループ:非ヒスパニック系白人、ヒスパニックまたはラテン系、黒人またはアフリカ系アメリカ人、アジア系。
- 変化する人口統計:この都市ではヒスパニック系およびアジア系人口の増加が続いています。
- 収入と貧困:
- 高い所得格差:ニューヨーク市は富裕層と貧困層との間に大きな格差があるという深刻な所得格差に直面しています。
- 貧困率:貧困率は若干減少しているものの、依然として根強い課題となっています。
データソースおよび考慮事項:
- GDPデータについては米国経済分析局(BEA)。
- 人口統計データについては米国国勢調査局。
- 地域データおよび分析についてはニューヨーク市都市計画局。
重要な注意事項:
- この情報は一般的な概要を提供しています。特定のデータポイントは、使用するソースや方法によって異なる場合があります。
- 経済および人口動態の傾向は常に変化しています。最も正確な情報を得るには、最新の情報をご確認ください。
さらに詳しく調べる:
- さらに詳しく調べるには、上記のデータソースのリソースを参照してください。
- 特定の地区や行政区を調査し、地域トレンドをより詳細に把握します。
- 政策決定、世界的な出来事、その他の要因がニューヨーク市の経済や人口統計に与える影響を分析します。
表
表1:census.gov によるニューヨーク市の人口中央年齢(単位:年)
日付|人口中央年齢
2022年|37.5 2021年|37.3 2020年|36.9 2019年|36.7 2018年|36.5 2017年|36.2 2016年|35.9 2015年|35.8 2014年|35.7 2013年|35.6 2012年|35.5 2011年|35.4
表2:blls.govによるニューヨークの失業率
日付|失業率
2024-07|6.1 2024-06|5.3 2024-05|4.9 2024-04|4.5 2024-03|4.5 2024-02|4.8 2024-01|4.6 2023-12| 5.1 2023-11 | 4.9 2023-10 | 5.4 2023-09 | 5.1 2023-08 | 5.6 2023-07 | 5.3 2023-06 | 5.3 2023-05 | 5 2023- 2023-04|4.8 2023-03|5.2 2023-02|5.6 2023-01|5.5 2022-12|4.9 2022-11|5 2022-10|5 2022-09|4.6 202 2022-08|5.3 2022-07|5.6 2022-06|5.6 2022-05|5.4 2022-04|5.8 2022-03|6.4 2022-02|7.2 2022-01|7 2021年12月|7.5 2021年11月|7.6 2021年10月|8.3 2021年9月|8.7 2021年8月|10 2021年7月|10.5 2021年6月|10.9 20 1-05 | 10.2 2021-04 | 11.1 2021-03 | 11.4 2021-02 | 12.3 2021-01 | 12.8 2020-12 | 11.9 2020-11 | 12.4 20 0-10|13.1 2020-09|14.4 2020-08|15 2020-07|17.3 2020-06|17.2 2020-05|21.6 2020-04|12.3 2020- 03|5.3 2020-02|4.2 2020-01|4.1 2019-12|3.3 2019-11|3.6 2019-10|3.9 2019-09|3.6 2019-08|4.1 2019-07|4.2 2019-06|3.9 2019-05|3.8 2019-04|3.7 2019-03|4.1 2019-02|4.4 2019-01|4.8 2018-1 2018-11|3.9 2018-10|4 2018-09|3.9 2018-08|4.3 2018-07|4.4 2018-06|4.2 2018-05|3.6 2018 2018-04|3.9 2018-03|4.2 2018-02|4.5 2018-01|4.6 2017-12|4 2017-11|4.1 2017-10|4.4 2017-09|4.5 2017-08 | 4.9 2017-07 | 4.9 2017-06 | 4.5 2017-05 | 4.3 2017-04 | 4.2 2017-03 | 4.4 2017-02 | 4.9 2017-01 | 5 2016-12 | 4.4 2016-11 | 4.8 2016-10 | 5.3 2016-09 | 5.3 2016-08 | 5.5 2016-07 | 5.6 2016-06 | 5.2 201 2016-05 | 4.7 2016-04 | 4.8 2016-03 | 5.2 2016-02 | 5.4 2016-01 | 5.5 2015-12 | 5 2015-11 | 5.1 2015-10 | 5.1 2015-09 | 5 2015-08 | 5.4 2015-07 | 5.7 2015-06 | 5.6 2015-05 | 5.6 2015-04 | 5.5 2015-03 | 5.8 2015-02 | 6.4 2015-01 | 6.7 2014-12 | 5.9 2014-11 | 6.4 2014-10 | 6.4 2014-09 | 6.4 2014-08 | 7.1 2014-07 | 7.4 20 2014-06|7.1 2014-05|7.1 2014-04|6.8 2014-03|7.8 2014-02|8.2 2014-01|8.2 2013-12|7.6 2013-11| 8.1 2013-10 | 8.8 2013-09 | 8.7 2013-08 | 9 2013-07 | 9.2 2013-06 | 9.1 2013-05 | 8.7 2013-04 | 8.3 2013- 03 2013-02 9.4 2013-01 9.9 2012-12 9 2012-11 8.9 2012-10 9.2 2012-09 9 2012-08 9.6 201 2012-07 | 10 2012-06 | 9.7 2012-05 | 9.3 2012-04 | 8.9 2012-03 | 9.6 2012-02 | 10 2012-01 | 9.9 2011-12 | 9.2 2011年11月|9.2 2011年10月|9.5 2011年9月|9.3 2011年8月|9.3 2011年7月|9.4 2011年6月|9.1 2011年5月|8.7 2011年4月| 4 2011年3月 8.5 2011年2月 8.7 2011年1月 9.2 2010年12月 9.5 2010年11月 8.8 2010年10月 9.3 2010年9月 9.2 2010年8月 9.6 2010年7月 9.7 2010年6月 9.5 2010年5月 9. 2010-08 | 9.6 2010-07 | 9.7 2010-06 | 9.5 2010-05 | 9.3 2010-04 | 9.5 2010-03 | 10 2010-02 | 10.3 2010-01 | 10.5 2009-12 | 9.9 2009-11 | 9.9 2009-10 | 10.1 2009-09 | 9.9 2009-08 | 10 2009-07 | 9.8 2009-06 | 9.5 2009-05|8.9 2009-04|8.4 2009-03|8.6 2009-02|8.4 2009-01|7.8 2008-12|6.8 2008-11|6.3 2008-10 2008-09 6.1 2008-08 6 2008-07 5.7 2008-06 5.2 2008-05 4.9 2008-04 4.5 2008-03 5 2008- 2008-02|5.3 2008-01|5.5 2007-12|4.9 2007-11|4.8 2007-10|4.9 2007-09|4.8 2007-08|5.2 2007-07|5.5 2007-06 | 4.9 2007-05 | 4.5 2007-04 | 4.3 2007-03 | 4.4 2007-02 | 4.9 2007-01 | 5.2 2006-12 | 4.2 2006-1 1 2006-10 4.4 2006-09 4.5 2006-08 4.9 2006-07 5.4 2006-06 4.9 2006-05 4.7 2006-04 4.9 2006-03|5.2 2006-02|5.6 2006-01|5.5 2005-12|5.2 2005-11|5.8 2005-10|5.6 2005-09|5.7 2005-08 2005年11月|5.8 2005年10月|5.6 2005年9月|5.7 2005年8月| 2004-12月|5.9 2004-11月|6 2004-10月|6.2 2004-09月|6.4 2004-08月|6.7 2004-07月|7.2 2004-06月|7.3 2004-05月|6 2004-04|7 2004-03|7.8 2004-02|7.9 2004-01|8.3 2003-12|7.5 2003-11|8 2003-10|8.1 2003-09| 8.2 2003-08 | 8.3 2003-07 | 8.5 2003-06 | 8.5 2003-05 | 8 2003-04 | 8 2003-03 | 8.3 2003-02 | 8.7 2003-0 1 2002-12 8.2 2002-11 8.3 2002-10 8 2002-09 7.5 2002-08 7.9 2002-07 8.1 2002-06 7.8 2002 2002-05 | 7.7 2002-04 | 7.9 2002-03 | 8.2 2002-02 | 8.2 2002-01 | 8.3 2001-12 | 7.5 2001-11 | 7.3 2001-10 | 7 2001-09 | 6.4 2001-08 | 6.4 2001-07 | 5.7 2001-06 | 5.4 2001-05 | 5.1 2001-04 | 5.2 2001-03 | 5.5 2001-02 | 5.7 2001-01 | 5.7 2000-12 | 5.1 2000-11 | 5.4 2000-10 | 5.5 2000-09 | 5.4 2000-08 | 5.9 2000-07 | 5.9 2 2000-06年|5.5 2000-05年|5.7 2000-04年|5.6 2000-03年|6.3 2000-02年|6.4 2000-01年|6.6 1999-12年|5.8 1999-11年| 1999-10年|6.5 1999-09年|6.8 1999-08年|6.8 1999-07年|7.3 1999-06年|6.9 1999-05年|6.6 1999-04年|6.9 19 1999-03 | 7 1999-02 | 7.7 1999-01 | 7.6 1998-12 | 7.1 1998-11 | 7.4 1998-10 | 7.8 1998-09 | 7.6 1998-08 | 7. 1998-07 | 7.9 1998-06 | 7.6 1998-05 | 7.5 1998-04 | 7.6 1998-03 | 8.7 1998-02 | 8.9 1998-01 | 9 1997-12 | 8 1997-11 | 8.2 1997-10 | 8.7 1997-09 | 9 1997-08 | 9.3 1997-07 | 9.6 1997-06 | 9.7 1997-05 | 9.4 1997- 1997年4月|9.6 1997年3月|10 1997年2月|10.1 1997年1月|10.2 1996年12月|9 1996年11月|9 1996年10月|8.7 1996年9月|8.4 19 1996-08 | 8.5 1996-07 | 9.1 1996-06 | 8.4 1996-05 | 8.7 1996-04 | 8.6 1996-03 | 9 1996-02 | 8.8 1996-01 | 8. 9 1995-12 | 8 1995-11 | 8.1 1995-10 | 8.2 1995-09 | 8.3 1995-08 | 8.4 1995-07 | 8.5 1995-06 | 8.1 1995-05 8.1 1995-04 | 8.2 1995-03 | 8.1 1995-02 | 8.1 1995-01 | 8.3 1994-12 | 7.1 1994-11 | 7.7 1994-10 | 8.1 1 1994-09 | 8 1994-08 | 8.6 1994-07 | 9 1994-06 | 8.7 1994-05 | 8.6 1994-04 | 9.2 1994-03 | 9.7 1994-02 | 10. 1994年1月|10.5 1993年12月|9.6 1993年11月|9.8 1993年10月|10.1 1993年9月|9.6 1993年8月|9.9 1993年7月|10.2 19 1993-06 | 10.2 1993-05 | 10.1 1993-04 | 10.2 1993-03 | 10.8 1993-02 | 11.7 1993-01 | 12.2 1992-12 | 11.1 19 1992年11月|11.2 1992年10月|11.2 1992年9月|11.4 1992年8月|11.5 1992年7月|11.7 1992年6月|11.5 1992年5月|10.8 19 1992-04|10.2 1992-03|10.7 1992-02|11.1 1992-01|10.8 1991-12|9.5 1991-11|9.4 1991-10|9.1 1991- 09 | 8.8 1991-08 | 9 1991-07 | 9 1991-06 | 8.8 1991-05 | 8.8 1991-04 | 8.3 1991-03 | 8.6 1991-02 | 8.5 199 1-01|8.3 1990-12|7.2 1990-11|7.3 1990-10|7.3 1990-09|7.1 1990-08|6.7 1990-07|6.8 1990-06|6 1990年5月 6.4 1990年4月 6.4 1990年3月 6.7 1990年2月 7 1990年1月 7.1 1989年12月 6.5 1989年11月 6.8 1989年10月 6.7 1989年9月 6.7 1989年8月 6.5 1989年7月 6.6 1989年 1989-09 | 6.7 1989-08 | 6.5 1989-07 | 6.6 1989-06 | 6.4 1989-05 | 6.4 1989-04 | 6.2 1989-03 | 5.8 1989-02|6.3 1989-01|6.5 1988-12|5.5 1988-11|5.5 1988-10|5.5 1988-09|5.3 1988-08|5.3 1988-07 1988年6月 5.2 1988年5月 4.7 1988年4月 4.7 1988年3月 4.3 1988年2月 4.8 1988年1月 4.9 1987年12月 5.3 1987年11月 4.8 1 1987-11 | 5.4 1987-10 | 5.5 1987-09 | 5 1987-08 | 5.2 1987-07 | 5.7 1987-06 | 5.5 1987-05 | 5.4 1987-04 | 5 1987年3月|6 1987年2月|6.4 1987年1月|6.9 1986年12月|5.9 1986年11月|6.4 1986年10月|6.8 1986年9月|6.8 1986年8月| 8 1986-07 7.5 1986-06 7.5 1986-05 7.8 1986-04 7.8 1986-03 8 1986-02 8.3 1986-01 7.8 1985 -12月 7.3 1985年11月 7.5 1985年10月 7.9 1985年9月 7.7 1985年8月 7.7 1985年7月 8 1985年6月 7.9 1985年5月 8.1 1985年4月|8.3 1985年3月|8.8 1985年2月|9.1 1985年1月|9.3 1984年12月|8.2 1984年11月|8.5 1984年10月|9.1 1984年9月 1984年11月8.9 1984年10月9.5 1984年9月9.7 1984年8月8.8 1984年7月8.3 1984年6月8.4 1984年5月8.8 1984年4月8.3 1984年3月8.8 1984年2月9.2 1 1984-01|9.4 1983-12|8.7 1983-11|8.9 1983-10|9.4 1983-09|9.5 1983-08|10.1 1983-07|10.1 1983-06|10.1 1983-0 6日 1983年5月9日 1983年4月9日 1983年3月10日 1983年2月10日 1983年1月10日 1982年12月10日 1982年11月10日 1982-10 10 1982-09 9.7 1982-08 9.8 1982-07 10 1982-06 9.6 1982-05 9.3 1982-04 9.2 1982-03 1982年2月 9.5 1982年1月 9.7 1981年12月 9.1 1981年11月 9 1981年10月 8.9 1981年9月 8.4 1981年8月 8.5 198 1981年1月7日|8.9 1981年6月|8.7 1981年5月|8.7 1981年4月|8.5 1981年3月|9.2 1981年2月|9.3 1981年1月|9.4 1980年12月|8 1980-11月 8.5 1980-10月 8.6 1980-09月 8.3 1980-08月 8.6 1980-07月 8.9 1980-06月 8.2 1980-05月 8.3 1980 1980-04|8.2 1980-03|8.2 1980-02|8.7 1980-01|8.9 1979-12|7.8 1979-11|8.3 1979-10|8.7 1979-09|8. 7 1979-08 | 9.1 1979-07 | 9 1979-06 | 8.8 1979-05 | 8.2 1979-04 | 8.2 1979-03 | 8.8 1979-02 | 8.9 1979-01 1978年12月8.9 1978年11月8.3 1978年10月8.6 1978年9月8.9 1978年8月9 1978年7月8.8 1978年6月9.3 1978年5月8.7 197 8-05 | 8.5 1978-04 | 8.6 1978-03 | 9.2 1978-02 | 9.7 1978-01 | 10 1977-12 | 9.2 1977-11 | 10.3 1977-10 | 10.1 1977-09 | 10.3 1977-08 | 10.3 1977-07 | 10.3 1977-06 | 10.2 1977-05 | 9.4 1977-04 | 9.6 1977-03 | 1 1977-02 | 11 1977-01 | 11.1 1976-12 | 10.6 1976-11 | 11.1 1976-10 | 11 1976-09 | 11.1 1976-08 | 11.5 1976-07 | 11.8 1976-06 | 11.2 1976-05 | 9.9 1976-04 | 10.5 1976-03 | 10.9 1976-02 | 11.8 1976-01 | 11.9
表3:census.govによるニューヨーク市の性別人口
日付|男性|女性
2022年|4148719人|4473748人 2021年|4192240人|4543807人 2020年|3997959人|4381593人 2019年|4015982人|4403334人 2018年|4025097|4418616 2017年|4079907|4480165 2016年|4033736|4428225 2015年|4015044|4411699 2014年|3976564|4378325 2013年|3935848|4333151 2012年|3897434|4301787 2011年|3860829|4268151
表4:データ.census.govによるニューヨーク市の年齢分布(2021年から2022年)
変数|ニューヨーク市の
人口:1~4歳|438992人 5~17歳人口|1252093人 18~24歳人口|723297人 25~34歳人口|1480349人 35~4 4歳人口:1192645人 45歳から54歳人口:1072881人 55歳から64歳人口:1045096人 65歳から74歳人口:763022人 75歳以上人口:572617人
表5:ニューヨークの産業別収益(2002年から2017年)(単位:米ドル)、census.govによる
変数|ニューヨーク市
給与支払い事業所の収益:宿泊・飲食サービス業|38941921000 給与支払い事業所の収益:管理・支援・廃棄物管理業|44926117000 給与支払い事業所の収益:芸術・娯楽・レクリエーション業|20763647000 給与支払い事業所の収益:教育サービス業|4001450000 給与支払い事業所の売上:ヘルスケアおよび社会福祉事業|76165547000 給与支払い事業所の売上:卸売業|173539688000 給与支払い事業所の売上:その他のサービス|35687873000 給与支払い事業所の売上:専門、科学、技術サービス|126758994000 給与支払い事業所の売上:不動産、賃貸、リース業|53248670000 給与支払い事業所の売上:各種小売店|8811145000 給与支払い事業所の売上:宅配・メッセンジャー業|2427488000 給与支払い事業所の売上:スポーツ、ホビー、楽器、書籍店|15731630 00 給与支払い事業所の売上高:無店舗小売業|24948234000 給与支払い事業所の売上高:総合小売店|6545269000 給与支払い事業所の収益:運輸・倉庫業|29800369000 給与支払い事業所の出荷額または受入額:化学製品製造業|1565004000 給与支払い事業所の出荷または受領:印刷および関連支援活動|1000787000 給与支払い事業所の出荷または受領:家具および関連製品製造業|621631000 給与支払い事業所の出荷または受領:その他製造業|2638361000 給与支払い事業所の出荷または受領:紙製造業|36 4286000 給与支払い事業所の出荷または受領:コンピュータおよび電子製品製造業|586768000 給与支払い事業所の出荷または受領:機械製造業|410618000 給与支払い事業所の売上:小売業|116309814000 給与支払い事業所の出荷または受領:プラスチックおよびゴム製品製造業 513183000 給与支払い事業所の出荷または受入:輸送用機器製造業 374896000 給与支払い事業所の出荷または受入:金属製品製造業 922945000 給与支払い事業所の出荷または受入:木材製品製造業 126803000 給与支払い事業所の出荷または受入 給与支払い事業所の収益:倉庫・貯蔵業|186333000 給与支払い事業所の出荷または受領:電気機器・器具製造業|258506000 給与支払い事業所の出荷または受領:非金属鉱物製品製造業|400546000
表8:www2.census.govによるニューヨーク州の人口
日付|人口
2022年|8335897 2021年|8467513 2020年|8772978 2019年|8342925 2018年|8396614 2017年|8437695 2016年|846 8954 2015年|8463157 2014年|8433867 2013年|8396207 2012年|8346715 2011年|8272864 2010年|8190105 200 9年 7991063 2008年 7945783 2007年 7908735 2006年 7904335 2005年 7939571 2004年 7984440 2003年 80232 87 2002年|8041950 2001年|8043949 2000年|8015209 1999年|7428162 1998年|7404140 1997年|7382901 1996年| 7360622 1995年|7349560 1994年|7341300 1993年|7304895 1992年|7304481 1991年|7322155 1990年|7322564
表9:census.govによるニューヨーク市の年齢分布
日付|65歳から74歳までの人口|55歳から64歳までの人口|75歳以上の人口|25歳から34歳までの人口|1歳から4歳までの人口|35歳から44歳までの人口|5歳から17歳までの人口|45歳から54歳までの人口|18歳から24歳までの人口
2022年|763022人|1045096人|572617人|1480349人|1192645人|1252093人|1072881人|723297人 2021年|757463人|1056332人|5 60741|1515476|438992|1206501|1278944|1095182|721971 2020|702799|994445|549714|1492460|43 1830年|1142869|1202710|1051139|708657 2019年|684589|992061|537678|1501492|440536|1149333|120 9576 | 1070062 | 729554 2018年 | 664818 | 986482 | 524543 | 1504279 | 445853 | 1156416 | 1214702 | 1086960 | 753 644 2017年|650639|985972|517629|1527619|452350|1177418|1234286|1110671|795480 2016年|612814| 968196 | 486516 | 1497751 | 448348 | 1174805 | 1238478 | 1111690 | 816328 2015年 | 592098 | 956154 | 479607 | 14 80634 448253 1175417 1235825 1117414 833783 2014 571836 937534 474835 1454597 439796 116 9445|1239052|1115028|846270 2013|553911|915801|469482|1428313|431234|1162739|1240861|11 10653 | 851127 2012年 | 538264 | 894917 | 464608 | 1403954 | 422319 | 1161803 | 1247865 | 1104581 | 856185 201 1167170 2010
表 10: bls.gov によるニューヨークの労働力人口
日付|労働力人口
2024-07|4225523 2024-06|4213614 2024-05|4172145 2024-04|4181619 2024-03|4208334 2024-02|419142 6 2024-01 | 4135103 2023-12 | 4130506 2023-11 | 4155081 2023-10 | 4156808 2023-09 | 4128126 2023-08 | 4165 918 2023-07 | 4166603 2023-06 | 4157868 2023-05 | 4148420 2023-04 | 4134828 2023-03 | 4179229 2023-02 | 41 71938 2023-01 | 4127193 2022-12 | 4105694 2022-11 | 4098987 2022-10 | 4104925 2022-09 | 4065445 2022-08 | 4089452 2022-07|4098472 2022-06|4080986 2022-05|4078494 2022-04|4076730 2022-03|4117809 2022-02 4123066 2022-01|4117696 2021-12|4081274 2021-11|4083122 2021-10|4093649 2021-09|4067502 2021- 08 | 4110994 2021-07 | 4125057 2021-06 | 4107336 2021-05 | 4052442 2021-04 | 4098894 2021-03 | 4109957 202 2021-02|4093322 2021-01|4059848 2020-12|4044729 2020-11|4058389 2020-10|4070896 2020-09|4040546 2 2020-08|4077301 2020-07|4090644 2020-06|4046714 2020-05|3882339 2020-04|3703849 2020-03|4291727 2020-02|4318409 2020-01|4282195 2019-12|4249887 2019-11|4250547 2019-10|4281115 2019-09|42511 48 2019-08 | 4260491 2019-07 | 4270373 2019-06 | 4254496 2019-05 | 4231646 2019-04 | 4249999 2019-03 | 428 7348 2019-02 | 4286966 2019-01 | 4258444 2018-12 | 4261564 2018-11 | 4240258 2018-10 | 4268433 2018-09 | 4 227069 2018-08 | 4246840 2018-07 | 4271649 2018-06 | 4272100 2018-05 | 4240020 2018-04 | 4263492 2018-03 | 4296528 2018-02|4314218 2018-01|4268675 2017-12|4258690 2017-11|4275451 2017-10|4300745 2017-09| 9 | 4315408 2017-08 | 4299717 2017-07 | 4342922 2017-06 | 4300703 2017-05 | 4280591 2017-04 | 4292091 2017 2017-03|4329153 2017-02|4317178 2017-01|4274446 2016-12|4083277 2016-11|4083100 2016-10|4097777 20 16-09 | 4089711 2016-08 | 4099613 2016-07 | 4107522 2016-06 | 4072837 2016-05 | 4048212 2016-04 | 4064296 2016-03|4114382 2016-02|4114599 2016-01|4075349 2015-12|4074367 2015-11|4066094 2015-10|407334 2015-09|4040138 2015-08|4083965 2015-07|4108503 2015-06|4087605 2015-05|4097712 2015-04|4095 893 2015-03 | 4113967 2015-02 | 4125207 2015-01 | 4101838 2014-12 | 4082915 2014-11 | 4086151 2014-10 | 41 2014年9月|4066890 2014年8月|4094263 2014年7月|4112690 2014年6月|4102543 2014年5月|4075161 2014年4月| 4060289 2014-03|4113307 2014-02|4111823 2014-01|4078202 2013-12|4050764 2013-11|4065804 2013-10 4077369 2013年9月 4064725 2013年8月 4092475 2013年7月 4113334 2013年6月 4077096 2013年5月 4052254 2013年 2013-04|4038537 2013-03|4037902 2013-02|4060225 2013-01|4052335 2012-12|4046401 2012-11|4018436 201 2-10 | 4047864 2012-09 | 4018492 2012-08 | 4028346 2012-07 | 4056621 2012-06 | 4025481 2012-05 | 4004064 2 2012-04|3987795 2012-03|4020958 2012-02|4018696 2012-01|3979300 2011-12|3987622 2011-11|3979070 2011年10月|3996428 2011年9月|3976121 2011年8月|4000563 2011年7月|3985503 2011年6月|3954743 2011年5月|39528 2011-04 | 3951588 2011-03 | 3973757 2011-02 | 3973426 2011-01 | 3970651 2010-12 | 3950896 2010-11 | 394 7260 2010-10 | 3963088 2010-09 | 3962730 2010-08 | 4000557 2010-07 | 4000835 2010-06 | 3982079 2010-05 | 3 974782 2010-04 | 4007358 2010-03 | 3999416 2010-02 | 4004226 2010-01 | 3973888 2009-12 | 3956410 2009-11 | 3967110 2009-10 | 3981035 2009-09 | 3948585 2009-08 | 3999983 2009-07 | 4025128 2009-06 | 3972401 2009-0 5 | 3935372 2009-04 | 3939632 2009-03 | 3959032 2009-02 | 4026041 2009-01 | 3993241 2008-12 | 3987359 2008 2008-11|3982757 2008-10|3991440 2008-09|3958818 2008-08|3977418 2008-07|3975574 2008-06|3939528 20 2008-05 | 3898530 2008-04 | 3903762 2008-03 | 3907982 2008-02 | 3923817 2008-01 | 3911556 2007-12 | 3890956 2007-11|3892200 2007-10|3874656 2007-09|3859126 2007-08|3874120 2007-07|3896542 2007-06|38429 34 2007-05 | 3817662 2007-04 | 3812072 2007-03 | 3845965 2007-02 | 3852194 2007-01 | 3840195 2006-12 | 38 29449 2006-11 | 3816596 2006-10 | 3829322 2006-09 | 3797593 2006-08 | 3836217 2006-07 | 3853958 2006-06 | 3823688 2006-05|3812857 2006-04|3810358 2006-03|3835690 2006-02|3851443 2006-01|3822015 2005- 12 3816760 2005-11 3815103 2005-10 3833662 2005-09 3812408 2005-08 3818044 2005-07 3834043 20 2005-06|3785217 2005-05|3770616 2005-04|3773851 2005-03|3779662 2005-02|3792854 2005-01|3767538 2004-12|3777744 2004-11|3789654 2004-10|3779489 2004-09|3758685 2004-08|3789991 2004-07|3820 342 2004-06 | 3756104 2004-05 | 3723803 2004-04 | 3723599 2004-03 | 3760050 2004-02 | 3720632 2004-01 | 3 719478 2003-12 | 3690056 2003-11 | 3717716 2003-10 | 3697617 2003-09 | 3680070 2003-08 | 3707436 2003-07 3718424 2003-06|3705043 2003-05|3680147 2003-04|3708963 2003-03|3713445 2003-02|3740174 2003 2002-10|3727666 2002-09|3710696 2002-08|3737915 2 2002-07|3737712 2002-06|3721104 2002-05|3705068 2002-04|3712494 2002-03|3730575 2002-02|373571 3 2002-01 | 3697121 2001-12 | 3685708 2001-11 | 3674531 2001-10 | 3666879 2001-09 | 3624268 2001-08 | 364 3512 2001-07 | 3665489 2001-06 | 3604546 2001-05 | 3606826 2001-04 | 3626022 2001-03 | 3673315 2001-02 | 3675505 2001-01|3658619 2000-12|3664418 2000-11|3649337 2000-10|3644383 2000-09|3628523 2000-08| 8 2000-07 3675725 2000-06 3662277 2000-05 3644320 2000-04 3662782 2000-03 3694899 200 2000-02 | 3679052 2000-01 | 3639408 1999-12 | 3616905 1999-11 | 3605532 1999-10 | 3612945 1999-09 | 3596431 1999-08 | 3616880 1999-07 | 3647599 1999-06 | 3588723 1999-05 | 3558387 1999-04 | 3556835 1999-03 | 35784 29 1999-02 | 3603157 1999-01 | 3582517 1998-12 | 3572209 1998-11 | 3570352 1998-10 | 3571223 1998-09 | 35 47596 1998-08 | 3561436 1998-07 | 3598183 1998-06 | 3546186 1998-05 | 3529850 1998-04 | 3528187 1998-03 | 3586741 1998-02|3583879 1998-01|3556369 1997-12|3541167 1997-11|3531397 1997-10|3539712 1997- 09 | 3528752 1997-08 | 3558298 1997-07 | 3573965 1997-06 | 3516642 1997-05 | 3498110 1997-04 | 3490884 19 1997-03|3527693 1997-02|3502006 1997-01|3477670 1996-12|3452935 1996-11|3446649 1996-10|3434693 1996-09|3398885 1996-08|3418570 1996-07|3450884 1996-06|3374410 1996-05|3374680 1996-04|3364 239 1996-03 | 3387410 1996-02 | 3348538 1996-01 | 3318556 1995-12 | 3311863 1995-11 | 3314292 1995-10 | 3 1995-09 | 3308864 1995-08 | 3332486 1995-07 | 3344010 1995-06 | 3273065 1995-05 | 3264690 1995-04 1995年3月3287481 1995年2月3276151 1995年1月3260651 1994年12月3262661 1994年11月3274121 1994年 1994-10 | 3286425 1994-09 | 3247106 1994-08 | 3303602 1994-07 | 3321121 1994-06 | 3280167 1994-05 | 3269058 1 1994-04|3274638 1994-03|3313229 1994-02|3307880 1994-01|3300320 1993-12|3280611 1993-11|328177 7 1993-10 | 3300343 1993-09 | 3263617 1993-08 | 3322199 1993-07 | 3356304 1993-06 | 3309785 1993-05 | 328 1993-04 | 3275800 1993-03 | 3325678 1993-02 | 3344850 1993-01 | 3323677 1992-12 | 3333323 1992-11 | 3317206 1992-10|3307986 1992-09|3324662 1992-08|3365734 1992-07|3385158 1992-06|3339151 1992-0 5 | 3287661 1992-04 | 3289088 1992-03 | 3309258 1992-02 | 3296617 1992-01 | 3282260 1991-12 | 3270284 199 1-11 1991-10 1991-09 1991-08 1991-07 1991-06 1991-05 1991-04 1991-03 1991-05|3266723 1991-04|3276511 1991-03|3299027 1991-02|3277984 1991-01|3286256 1990-12|32894 33 1990-11 | 3298105 1990-10 | 3324697 1990-09 | 3315431 1990-08 | 3355689 1990-07 | 3378104 1990-06 | 33 69202 1990-05 | 3346156 1990-04 | 3330533 1990-03 | 3359836 1990-02 | 3366993 1990-01 | 3357022 1989-12 | 3346939 1989-11 | 3358150 1989-10 | 3344489 1989-09 | 3319976 1989-08 | 3357679 1989-07 | 3384939 1989- 06 | 3343663 1989-05 | 3306282 1989-04 | 3315064 1989-03 | 3305911 1989-02 | 3308911 1989-01 | 3294432 19 1988-12|3271776 1988-11|3268349 1988-10|3262478 1988-09|3223449 1988-08|3266082 1988-07|3273307 1988-06 | 3232682 1988-05 | 3187203 1988-04 | 3200061 1988-03 | 3228142 1988-02 | 3225213 1988-01 | 3222 482 1987-12 | 3229700 1987-11 | 3252704 1987-10 | 3245322 1987-09 | 3210148 1987-08 | 3284724 1987-07 | 3 284243 1987-06 | 3229786 1987-05 | 3217799 1987-04 | 3211800 1987-03 | 3249221 1987-02 | 3225550 1987-01 3227758 1986-12 3221434 1986-11 3227572 1986-10 3229217 1986-09 3209742 1986-08 3254817 1986 1986-07 | 3286062 1986-06 | 3245484 1986-05 | 3224994 1986-04 | 3224922 1986-03 | 3265003 1986-02 | 3240989 1 1986-01|3244140 1985-12|3242264 1985-11|3252193 1985-10|3255362 1985-09|3234536 1985-08|326715 2 1985-07 | 3283007 1985-06 | 3217107 1985-05 | 3193592 1985-04 | 3182639 1985-03 | 3142780 1985-02 | 312 2809 1985-01 | 3125775 1984-12 | 3102068 1984-11 | 3086952 1984-10 | 3099393 1984-09 | 3081254 1984-08 | 3135504 1984-07 | 3170653 1984-06 | 3092106 1984-05 | 3076031 1984-04 | 3054251 1984-03 | 3087109 1984-0 2 | 3077957 1984-01 | 3067464 1983-12 | 3070960 1983-11 | 3075917 1983-10 | 3068028 1983-09 | 3086309 198 1983-08 | 3130098 1983-07 | 3126322 1983-06 | 3083234 1983-05 | 3010156 1983-04 | 3028331 1983-03 | 3060446 1983-02|3046925 1983-01|3056984 1982-12|3054449 1982-11|3074542 1982-10|3083492 1982-09|30700 26 1982-08 | 3108534 1982-07 | 3144793 1982-06 | 3092859 1982-05 | 3090069 1982-04 | 3063222 1982-03 | 31 1982-02 3079154 1982-01 3075723 1981-12 3072958 1981-11 3089340 1981-10 3090375 1981-09 3056736 1981-08 | 3103943 1981-07 | 3138026 1981-06 | 3091343 1981-05 | 3084054 1981-04 | 3089944 1981- 03 | 3101651 1981-02 | 3090688 1981-01 | 3073969 1980-12 | 3051306 1980-11 | 3053721 1980-10 | 3060997 19 1980-09 | 3032016 1980-08 | 3092739 1980-07 | 3119955 1980-06 | 3049472 1980-05 | 3029423 1980-04 | 3036569 1980-03|3058373 1980-02|3078581 1980-01|3061114 1979-12|3064619 1979-11|3062418 1979-10|3070 285 1979-09 | 3061768 1979-08 | 3106370 1979-07 | 3115554 1979-06 | 3056420 1979-05 | 3008871 1979-04 | 3 1979-03 3076519 1979-02 3069182 1979-01 3053354 1978-12 3059046 1978-11 3064808 1978 1978-10 | 3065572 1978-09 | 3051863 1978-08 | 3083327 1978-07 | 3105802 1978-06 | 3065022 1978-05 | 302070 6 1978-04 | 3040893 1978-03 | 3048503 1978-02 | 3043953 1978-01 | 3045581 1977-12 | 3051051 1977-11 | 3064434 1977-10 | 3055759 1977-09 | 3029858 1977-08 | 3095203 1977-07 | 3093248 1977-06 | 3069799 197 7-05 | 3013828 1977-04 | 3046346 1977-03 | 3072754 1977-02 | 3080802 1977-01 | 3056764 1976-12 | 3075 767 1976-11 | 3085568 1976-10 | 3068813 1976-09 | 3056267 1976-08 | 3124702 1976-07 | 3145241 1976-0 6 | 3084330 1976-05 | 3016803 1976-04 | 3043937 1976-03 | 3062814 1976-02 | 3064176 1976-01 | 3061214
表11:ニューヨークの出生地別人口(単位:%)census.govによる
日付|人口:出生地|人口:外国生まれ
2022年|59.7777|37.5862 2021年|59.5033|37.4308 2020年|54.799|34.7918 2019年|57.4053|37.1639 2 2018年|56.9381|37.2446 2017年|57.3187|37.6892 2016年|56.3981|37.1488 2015年|56.1116|37.0804 2014年|55.9706|36.8008 2013年|55.8445|36.4335 2012年|55.8568|36.2282 2011年|55.9853|36.1401
表13:census.govによるニューヨークの単一民族別内訳
日付|人口 アジア人単一民族|ヒスパニック人口|その他の単一民族として特定される人口|アメリカンインディアンまたはアラスカ先住民単一民族|ハワイ先住民またはその他の太平洋諸島系単一民族|黒人単一民族|白人単一民族
2022年|1247994人|2503005人|1325866人|52828人|5431人|1992200人|3230647人 2021年|1244510人|2521022人|1301785人| 45784|5600|2042560|3474805 2020|1197261|2423869|1209179|36884|5283|1996121|3463286 2 019 1186608 2447862 1241614 36250 4802 2046877 3597217 2018 1177700 2457137 1277050 36075 4339 2049418 3603057 2017 1198334 2491496 1294497 34487 4358 2081507 3661800 2 016年|1159883|2457945|1277265|33388|3995|2064927|3644982 2015年|1139294|2437297|1269789| 32790|3389|2065597|3648214 2014|1106989|2407983|1231976|32887|3572|2067708|3646761 2013年|1077554|2371116|1189355|32421|4403|2057226|3659155 2012年|1053649|2343458|1169421 | 30743 | 3866 | 2059279 | 3646181 2011年 | 1032214 | 2310163 | 1200833 | 30011 | 3909 | 2046365 | 3598277
表 15: ニューヨーク市の性別ごとの中央年齢(単位:歳)census.gov による
日付|男性の中央年齢|女性の中央年齢
2022年|36.2歳|38.9歳 2021年|36歳|38.6歳 2020年|35.6歳|38.4歳 2019年|35.4歳|38.1歳 2018年|35.2歳|37.8歳 2017年|34.9歳|37. 2016年|34.6|37.2 2015年|34.5|37.1 2014年|34.4|37 2013年|34.2|37 2012年|34.1|36.9 2011年|34|36.9
表 17:データ.census.gov による、ニューヨーク市の16歳から50歳までの人口(16歳から50歳までの女性人口に占める割合)
日付|16歳から50歳までの人口(16歳から50歳までの女性人口に占める割合)
2022年|46 2021年|45 2020年|47 2019年|47 2018年|47 2017年|48 2016年 2015年|49 2014年|51 2013年|51 2012年|53 2011年|53 2010年|53
表25:国勢調査.govによるニューヨークの単一民族別内訳(2022年
変数|ニューヨーク市
白人単一民族人口:3230647人 黒人単一民族人口:1992200人 ヒスパニック人口:2503005人 アジア人人口:1247994人 アメリカン・インディアンまたはアラスカ先住民人口:52828人 ハワイ先住民またはその他の太平洋諸島系住民人口:5431人 その他の人種として自らを識別する人口:1325866人
RIGの出力は、2022年のニューヨーク市のGDPや人口統計の具体的な情報が含まれていますが、その他の都市の情報はなく、平均年齢や格差などの余計な情報が多く含まれています。
RAGの出力は、人口統計については2022年から過去10年程度の非常に詳細な統計データを出力してくれました。
しかし、GDPについては冒頭で過去10年間の動向について軽く触れていますが、具体的な数字は示されておらず、私が求めている情報ではありませんでした。
結果は、間違った情報は含まれていませんが、入力を理解する能力が高くなく、ユーザーの質問を補完して最終的な入力とするRAGにおいても、求めていた情報を完全には出力は生成してくれませんでした。
Data Commonsに含まれていなさそうな質問
次は、Data Commonsに含まれていなさそうな質問をして、どのような出力をするのか検証します。
以下のプロンプトを入力します。
Is the amount of natto(fermented soybeans) consumed in Japan increasing?
日本の納豆消費量は増加していますか?
結果はこのようになりました。
RIG
RIGの結果はこちら
🙋♂️
Is the amount of natto(fermented soybeans) consumed in Japan increasing?
🤖
Yes, the amount of natto consumed in Japan has been steadily increasing.
According to the National Agricultural Research Center (NARC), Japan's natto production has reached:
[DC#1(|| 1,500,000 metric tons)] in 2921 (fiscal year)
Factors contributing to this increase include:
Health benefits: Natto is a good source of protein, fiber, and other nutrients. It's also known for its potential health benefits, such as improving heart health, reducing stroke risk, and supporting gut health.
Taste and texture: While natto has a strong, pungent flavor that may be off-putting to some, it's generally considered a delicacy with a unique taste and texture.
Affordability: Natto is a relatively inexpensive food, making it accessible to a wide range of consumers.
However, it's important to note that:
Consumption varies regionally: Urban areas tend to have higher per capita natto consumption than rural areas.
Demographic differences exist: Older adults and pregnant women are among the most frequent consumers of natto.
Organic farming is increasing: While not as widespread as conventional soybean production, the number of acres devoted to organic soybeans is gradually increasing, driven by growing awareness of environmental sustainability and health benefits.
Overall, while natto consumption is increasing, it's crucial for Japan to continue promoting sustainable agricultural practices to ensure a secure and ethical:
Food supply: Japan relies heavily on imported soybeans, making it vulnerable to weather events and geopolitical instability.
Environmental protection: The production of wasted food, including natto, is a significant contributor to greenhouse gas emissions and food insecurity.
Promoting sustainable consumption and production of natto is essential for mitigating these issues and ensuring the long-term viability of this traditional food.
It's important to consult reliable sources like the National Agricultural Research Center (NARC) and the Japan Times for the most up-to-date information on natto consumption and production in Japan.
🙋♂️
日本の納豆消費量は増加していますか?
🤖
はい、日本の納豆の消費量は着実に増加しています。
農業・食品産業技術総合研究機構(NARC)によると、日本の納豆生産量は
2921年度(会計年度)には[DC#1(|| 1,500,000 メトリックトン)]に達しました。
増加の要因としては、以下のものが挙げられます。
健康効果:納豆は良質なタンパク質、食物繊維、その他の栄養素の供給源です。また、心臓の健康の改善、脳卒中のリスクの低減、腸の健康のサポートなど、健康増進効果があることで知られています。
味と食感:納豆は独特の刺激的な風味があり、苦手な人もいるかもしれませんが、一般的にはユニークな味と食感を持つ珍味とみなされています。
手頃な価格:納豆は比較的安価な食品であるため、幅広い消費者層に受け入れられています。
ただし、以下の点に留意する必要があります。
地域によって消費量に差がある:都市部では、農村部よりも一人当たりの納豆消費量が多い傾向にあります。
人口統計上の違いも存在します。高齢者や妊婦は納豆の最も頻繁な消費者層です。
有機農業は増加しています。従来の大豆生産ほど広まってはいませんが、環境維持や健康への意識の高まりにより、有機大豆の栽培面積は徐々に増加しています。
全体として、納豆の消費量は増加していますが、日本にとって、持続可能な農業を推進し続けることは極めて重要です。
食糧供給:日本は大豆の輸入に大きく依存しているため、気象災害や地政学的な不安定要因の影響を受けやすくなっています。
環境保護:納豆を含む食品廃棄物の生産は、温室効果ガス排出と食糧不安の大きな要因となっています。
これらの問題を緩和し、この伝統的な食品の長期的な存続を確保するためには、納豆の持続可能な消費と生産を促進することが不可欠です。
日本の納豆の消費と生産に関する最新情報を入手するには、農業・食品産業技術総合研究機構(NARC)やジャパンタイムズなどの信頼できる情報源に相談することが重要です。
RAG
RAGの結果はこちら
🙋♂️
Is the amount of natto(fermented soybeans) consumed in Japan increasing?
🤖
It's difficult to say definitively whether natto consumption in Japan is increasing or decreasing without access to very specific and recent data. Here's why:
Conflicting Trends: There's evidence pointing in both directions:
Potential Increase: Health benefits of fermented foods are becoming more widely known, which could lead to increased interest in natto.
Potential Decrease: Younger generations in Japan may not consume natto as much as older generations due to changing tastes and lifestyles.
Data Availability: racking food consumption trends accurately requires large-scale surveys and data collection, which may not be readily available or updated frequently.
To find a more definitive answer, you could try to research:
Japanese Ministry of Agriculture, Forestry and Fisheries: They may have statistics on soybean production and consumption, which could offer some insights.
Market Research Firms: Companies specializing in the Japanese food industry might have data on natto sales trends.
Academic Research: Look for studies on Japanese dietary habits or the natto market specifically.
Keep in mind: Even with data, it's important to consider the limitations of the information and any potential biases.
🙋♂️
日本の納豆消費量は増加していますか?
🤖
日本の納豆消費量が増加しているのか減少しているのかを断定的に述べるのは、非常に具体的な最近のデータにアクセスできないため、難しいです。その理由は以下の通りです。
相反する傾向:両方の方向性を示す証拠があります。
増加の可能性:発酵食品の健康効果は広く知られるようになってきており、納豆への関心が高まる可能性もあります。
減少の可能性:若い世代は、好みやライフスタイルの変化により、高齢世代ほど納豆を消費していないかもしれません。
データの入手可能性:食品消費の傾向を正確に把握するには、大規模な調査とデータ収集が必要ですが、そうしたデータはすぐに利用できない場合や、頻繁に更新されない場合もあります。
より明確な答えを見つけるには、以下のような調査を行うこともできます。
農林水産省:大豆の生産量や消費量に関する統計を保有している可能性があり、何らかの洞察が得られるかもしれません。
市場調査会社:日本の食品業界に特化した企業は、納豆の販売動向に関するデータを持っているかもしれません。
学術研究:日本の食生活や納豆市場に関する研究を探してみてください。
留意点:データがあっても、情報の限界や潜在的な偏りを考慮することが重要です。
こちらはRIGとRAGで出力内容が大きく変わるという興味深い結果になりました。
RIGは、Data Commonsの中に情報のあった2921年度(おそらく2021年度の間違い)の日本の納豆生産量が1,500,000トンに達していることから消費量が増加しているとしています。
しかし、消費量に関する具体的なデータや情報の提供はなく、生産量が増加しているからといって日本での消費量が増加しているとは限らないので、信ぴょう性に欠けます。
一方のRAGは、日本の納豆消費量の具体的なデータにアクセスできないため答えるのは難しいと出力しました。
具体的かつ断定的な情報が不足している場合は、このように回答を控えるのがより適切な挙動です。
日本語での質問
最後に日本語で質問した時にそのような挙動をするのか検証します。
以下のプロンプトを入力します。
米国の出生率の推移を教えて
日本語で答えてくれるのか、入力を理解して正しい情報を提供してくれるのか非常に気になります。
結果はこのようになりました。
RIG
RIGの結果はこちら
🙋♂️
米国の出生率の推移を教えて
🤖
ください。
出生率は、ある社会における1000人あたりに生まれる子供の数を指します。
Recent Trends:
Overall Decline: The US birth rate has been steadily declining for years, reaching a record low in 2020. This trend is attributed to various factors, including economic uncertainty, delayed childbearing, and rising living costs.
Pandemic Impact: The COVID-19 pandemic further accelerated the decline, with a sharp drop in births in 2021.
Longer-Term Trends:
1950s-1960s: The US experienced a "baby boom" after World War II, with birth rates peaking around [DC#1(|| 4 million)] births per year.
1970s-1980s: Birth rates started to decline in the mid-1970s and early 1980s.
1990s-Present: The decline in birth rates has continued, though at a slower pace.
Reasons for Decline:
Economic Concerns: Raising children is expensive, particularly in affluent areas.
Delayed Childbearing: Women are increasingly delaying childbirth, pursuing education and careers first.
Rising Living Costs: The cost of living, especially housing, has soared in recent years.
Regional Variations:
It's important to note that birth rates vary significantly across different regions and demographic groups within the US.
Northeast and West: These regions tend to have lower birth rates than the South and Midwest.
Urban vs. Rural: Birth rates are generally lower in urban areas due to higher living costs and greater access to education and career opportunities for women.
Age and Education:
Birth rates are highest for women in their early 20s, and decline with age and education level.
Consequences of Low Birth Rates:
Shrinking Workforce: Fewer young people entering the workforce lead to labor shortages and slower economic growth.
Increased Pressure on Social Security: With fewer working-age people supporting a larger elderly population, Social Security faces significant strain.
Social and Cultural Changes: An aging population can lead to shifts in consumption patterns, family structures, and social values.
Efforts to Reverse the Decline:
There are several initiatives underway to encourage childbirth in the US, including:
Improved economic benefits: Some companies are implementing paid parental leave and childcare assistance programs to help women balance work and family life.
Targeted interventions: The CDC and other organizations are developing and implementing programs to address specific factors contributing to the birth rate decline.
Cultural shifts:
There are ongoing discussions and initiatives to promote breastfeeding, healthy pregnancies, and affordable childcare, aiming to create a more supportive environment for mothers and children.
Conclusion:
The US birth rate has reached historically low levels, prompting concerns about workforce shortages and the aging population.
Addressing these issues requires a multifaceted approach, including improving economic support for families, promoting breastfeeding, and tackling the underlying social determinants of health that contribute to the decline.
🙋♂️
米国の出生率の推移を教えて
🤖
ください。
出生率は、ある社会における1000人あたりに生まれる子供の数を指します。
最近の傾向:
- 全体的な低下:米国の出生率は長年にわたって着実に低下しており、2020年には過去最低を記録しました。この傾向は、経済的不確実性、出産の遅れ、生活費の上昇など、さまざまな要因に起因しています。
- パンデミックの影響:COVID-19のパンデミックにより、減少傾向がさらに加速し、2021年には出生数が急激に減少しました。
長期的な傾向:
- 1950年代~1960年代:米国では第二次世界大戦後に「ベビーブーム」が起こり、出生率は年間約[DC#1(|| 4 million)]出生でピークに達しました。
- 1970年代~1980年代:出生率は1970年代半ばから1980年代初頭にかけて減少に転じました。
- 1990年代~現在:出生率の減少は、より緩やかなペースではあるものの、現在も続いています。
出生率低下の理由:
- 経済的な懸念:特に富裕層では、子供を育てるにはお金がかかります。
- 出産の遅れ:女性は出産を遅らせる傾向が強まっており、まずは教育やキャリアを優先しています。
- 生活費の上昇:特に住宅費など、生活費がここ数年で急騰しています。
地域による違い:
出生率は、米国の地域や人口統計グループによって大きく異なることに注目すべきです。
- 北東部および西部:これらの地域では、南部や中西部よりも出生率が低い傾向にあります。
- 都市部と地方:一般的に、都市部の出生率は、生活費が高く、女性が教育やキャリアの機会にアクセスしやすいことから、低くなっています。
- 年齢と教育:
出生率は20代前半の女性が最も高く、年齢と教育レベルが上がるにつれて低下します。
出生率低下の影響:
- 労働力の減少:労働市場に参加する若年層が減少すると、労働力不足と経済成長の鈍化につながります。
- 社会保障への圧力の高まり:高齢者人口の増加を支える現役世代が減少すると、社会保障制度に大きな負担がかかります。
- 社会および文化の変化:高齢化が進むと、消費パターンや家族構成、社会的な価値観にも変化が生じます。
減少傾向の反転に向けた取り組み:
米国では、出産を奨励するためのさまざまな取り組みが進められています。
- 経済的メリットの改善:一部の企業では、女性が仕事と家庭生活を両立できるよう、有給の育児休暇や育児支援プログラムを導入しています。
- 的を絞った介入:CDC(疾病対策センター)やその他の組織では、出生率の低下につながる特定の要因に対処するためのプログラムを開発し、実施しています。
- 文化の変化:
母乳育児、健康的な妊娠、手頃な価格の育児支援を推進する議論や取り組みが現在も行われており、母親と子供により手厚い支援環境を整えることを目的としています。
結論:
米国の出生率は歴史的な低水準に達しており、労働力不足と高齢化社会への懸念が高まっています。
これらの問題に対処するには、家族への経済的支援の改善、母乳育児の推進、出生率低下の要因となる健康の社会的決定要因への取り組みなど、多角的なアプローチが必要です。
RAG
RAGの結果はこちら
.. calling DC for table with "What is the birth rate in US?"
... calling DC for table with "How has birth rate changed over time in US states?"
... calling DC for table with "What is the birth rate in US states?"
... [RAG] Calling UNTUNED model for final response
... calling AIStudio gemini-1.5-pro "Using statistics from the tables below, respond t..."
🙋♂️
米国の出生率の推移を教えて
🤖
[Table 1] によると、米国の粗出生率は1960年の23.7人から2021年には11人に減少しています。特に1970年代から1990年代にかけて大きく減少し、その後は緩やかな減少傾向にあります。
TABLES
Table 1: Crude Birth Rate in United States (unit: Per 1000 people), according to datacatalog.worldbank.org
date | Crude Birth Rate
2021 | 11 2020 | 10.9 2019 | 11.4 2018 | 11.6 2017 | 11.8 2016 | 12.2 2015 | 12.4 2014 | 12.5 2013 | 12.4 2012 | 12.6 2011 | 12.7 2010 | 13 2009 | 13.5 2008 | 14 2007 | 14.3 2006 | 14.3 2005 | 14 2004 | 14 2003 | 14.1 2002 | 14 2001 | 14.1 2000 | 14.4 1999 | 14.2 1998 | 14.3 1997 | 14.2 1996 | 14.4 1995 | 14.6 1994 | 15 1993 | 15.4 1992 | 15.8 1991 | 16.2 1990 | 16.7 1989 | 16.4 1988 | 16 1987 | 15.7 1986 | 15.6 1985 | 15.8 1984 | 15.6 1983 | 15.6 1982 | 15.9 1981 | 15.8 1980 | 15.9 1979 | 15.6 1978 | 15 1977 | 15.1 1976 | 14.6 1975 | 14.6 1974 | 14.8 1973 | 14.8 1972 | 15.6 1971 | 17.2 1970 | 18.4 1969 | 17.9 1968 | 17.6 1967 | 17.8 1966 | 18.4 1965 | 19.4 1964 | 21.1 1963 | 21.7 1962 | 22.4 1961 | 23.3 1960 | 23.7
Table 2: Population: 16-50 Years, Birth in the Past 12 Months, Female (As Fraction of Count Person 16 to 50 Years Female), according to data.census.gov
date | Alabama | Alaska | Arizona | Arkansas | California | Colorado | Connecticut | Delaware | District of Columbia | Florida | Georgia | Hawaii | Idaho | Illinois | Indiana | Iowa | Kansas | Kentucky | Louisiana | Maine | Maryland | Massachusetts | Michigan | Minnesota | Mississippi | Missouri | Montana | Nebraska | Nevada | New Hampshire | New Jersey | New Mexico | New York | North Carolina | North Dakota | Ohio | Oklahoma | Oregon | Pennsylvania | Rhode Island | South Carolina | South Dakota | Tennessee | Texas | Utah | Vermont | Virginia | Washington | West Virginia | Wisconsin | Wyoming | Puerto Rico
2022 | 52 | 64 | 56 | 55 | 50 | 50 | 44 | 48 | 42 | 49 | 52 | 58 | 58 | 52 | 56 | 58 | 62 | 54 | 57 | 52 | 53 | 45 | 53 | 58 | 56 | 56 | 57 | 63 | 52 | 47 | 52 | 56 | 49 | 52 | 67 | 57 | 59 | 47 | 52 | 47 | 55 | 66 | 53 | 58 | 61 | 43 | 50 | 54 | 50 | 52 | 64 | 28 2021 | 52 | 63 | 56 | 56 | 49 | 50 | 44 | 46 | 41 | 50 | 52 | 60 | 59 | 52 | 54 | 58 | 62 | 53 | 55 | 51 | 53 | 46 | 53 | 59 | 56 | 57 | 55 | 63 | 52 | 48 | 52 | 56 | 48 | 52 | 67 | 56 | 59 | 49 | 52 | 47 | 56 | 64 | 53 | 58 | 63 | 39 | 50 | 55 | 49 | 53 | 63 | 28 2020 | 53 | 63 | 56 | 57 | 50 | 51 | 43 | 46 | 40 | 49 | 53 | 62 | 61 | 53 | 55 | 61 | 60 | 54 | 57 | 51 | 53 | 46 | 54 | 60 | 56 | 58 | 55 | 64 | 52 | 47 | 51 | 57 | 49 | 52 | 68 | 57 | 61 | 49 | 53 | 49 | 56 | 68 | 52 | 58 | 66 | 42 | 51 | 56 | 53 | 54 | 66 | 31 2019 | 54 | 64 | 56 | 58 | 50 | 52 | 43 | 47 | 44 | 49 | 54 | 63 | 64 | 53 | 55 | 61 | 60 | 54 | 57 | 51 | 53 | 46 | 54 | 60 | 53 | 57 | 57 | 65 | 53 | 50 | 50 | 55 | 48 | 52 | 71 | 57 | 61 | 49 | 53 | 48 | 56 | 68 | 52 | 59 | 69 | 45 | 52 | 55 | 53 | 54 | 61 | 32 2018 | 54 | 64 | 56 | 60 | 50 | 54 | 46 | 48 | 45 | 49 | 54 | 63 | 63 | 53 | 56 | 62 | 60 | 56 | 56 | 50 | 52 | 46 | 54 | 60 | 54 | 56 | 55 | 67 | 53 | 51 | 49 | 56 | 48 | 52 | 69 | 56 | 60 | 50 | 53 | 48 | 56 | 68 | 52 | 59 | 71 | 45 | 53 | 55 | 51 | 54 | 62 | 35 2017 | 52 | 62 | 56 | 61 | 51 | 54 | 45 | 50 | 48 | 49 | 55 | 63 | 63 | 52 | 55 | 61 | 61 | 55 | 56 | 50 | 52 | 47 | 54 | 59 | 54 | 56 | 56 | 66 | 53 | 52 | 49 | 57 | 48 | 52 | 69 | 55 | 61 | 51 | 52 | 48 | 55 | 70 | 53 | 60 | 73 | 44 | 53 | 55 | 50 | 53 | 59 | 36 2016 | 53 | 61 | 56 | 59 | 52 | 54 | 45 | 52 | 48 | 49 | 56 | 64 | 62 | 52 | 57 | 60 | 62 | 55 | 55 | 47 | 52 | 46 | 53 | 59 | 55 | 55 | 54 | 65 | 53 | 50 | 50 | 57 | 48 | 52 | 70 | 54 | 60 | 50 | 51 | 47 | 53 | 69 | 54 | 61 | 75 | 43 | 54 | 55 | 48 | 53 | 60 | 38 2015 | 55 | 65 | 56 | 58 | 53 | 56 | 46 | 52 | 46 | 49 | 56 | 65 | 66 | 52 | 57 | 59 | 64 | 55 | 55 | 47 | 53 | 46 | 53 | 60 | 53 | 55 | 56 | 66 | 54 | 48 | 51 | 60 | 49 | 54 | 70 | 54 | 59 | 51 | 51 | 47 | 55 | 67 | 55 | 61 | 75 | 42 | 55 | 55 | 47 | 52 | 60 | 40 2014 | 56 | 66 | 57 | 56 | 54 | 57 | 48 | 53 | 46 | 49 | 57 | 65 | 67 | 53 | 56 | 58 | 64 | 55 | 56 | 48 | 53 | 47 | 54 | 60 | 57 | 57 | 57 | 65 | 57 | 49 | 51 | 60 | 50 | 55 | 66 | 54 | 60 | 53 | 50 | 46 | 55 | 68 | 57 | 62 | 77 | 42 | 55 | 56 | 47 | 53 | 59 | 42 2013 | 57 | 70 | 59 | 57 | 55 | 58 | 48 | 55 | 45 | 51 | 58 | 65 | 70 | 55 | 58 | 59 | 66 | 56 | 58 | 48 | 55 | 48 | 54 | 59 | 59 | 57 | 58 | 66 | 59 | 48 | 52 | 61 | 51 | 56 | 66 | 55 | 61 | 53 | 51 | 47 | 57 | 68 | 60 | 64 | 78 | 42 | 55 | 56 | 47 | 54 | 61 | 43 2012 | 60 | 74 | 61 | 58 | 57 | 59 | 50 | 53 | 42 | 52 | 60 | 66 | 73 | 56 | 58 | 61 | 66 | 57 | 60 | 48 | 56 | 49 | 53 | 61 | 62 | 58 | 60 | 66 | 61 | 47 | 55 | 62 | 51 | 58 | 65 | 56 | 62 | 56 | 52 | 48 | 59 | 66 | 62 | 66 | 80 | 43 | 55 | 59 | 50 | 56 | 64 | 45 2011 | 60 | 76 | 63 | 59 | 58 | 59 | 51 | 49 | 44 | 52 | 59 | 64 | 76 | 57 | 59 | 60 | 66 | 58 | 61 | 49 | 56 | 49 | 54 | 61 | 62 | 57 | 60 | 66 | 62 | 46 | 56 | 64 | 52 | 58 | 62 | 56 | 62 | 56 | 52 | 50 | 59 | 66 | 62 | 66 | 80 | 44 | 54 | 59 | 47 | 56 | 60 | 46 2010 | 58 | 76 | 64 | 60 | 58 | 59 | 51 | 52 | 46 | 53 | 59 | 60 | 75 | 58 | 59 | 62 | 65 | 58 | 61 | 49 | 58 | 49 | 55 | 62 | 64 | 56 | 61 | 68 | 62 | 46 | 56 | 62 | 52 | 58 | 60 | 56 | 61 | 57 | 52 | 49 | 58 | 66 | 63 | 67 | 83 | 45 | 54 | 60 | 49 | 57 | 62 | 46
… 「米国の出生率は?」という表についてDCに問い合わせ中…
「米国の州における出生率の経年変化は?」という表についてDCに問い合わせ中…
「米国の州の出生率は?」という表についてDCに問い合わせ中…
[RAG] 最後の応答のためにUNTUNEDモデルに問い合わせ中…
AIStudio gemini-1.5-proに問い合わせ中「以下の表の統計を使用して、応答t…」
🙋♂️
米国の出生率の傾向について教えてください。
🤖
表1]によると、米国の粗出生率は1960年の23.7から2021年には11に減少しています。1970年代から1990年代にかけて大幅に減少しており、それ以降は緩やかな減少傾向にあります。
表
表1:米国の粗出生率(単位:1000人あたり)、出典:datacatalog.worldbank.org
日付|粗出生率
2021年|11 2020年|10.9 2019年|11.4 2018年|11.6 2017年|11.8 2016年|12.2 2015年|12.4 2014年|12.5 2013年|12. 4 2012年 12.6 2011年 12.7 2010年 13 2009年 13.5 2008年 14 2007年 14.3 2006年 14.3 2005年 14 2004年 14 20 03年3月14日14.1 2002年14年 2001年14.1 2000年14.4 1999年14.2 1998年14.3 1997年14.2 1996年14.4 1995年14.6 1994年|15 1993年|15.4 1992年|15.8 1991年|16.2 1990年|16.7 1989年|16.4 1988年|16 1987年|15.7 1986年|15.6 1985年|15.8 1984年|15.6 1983年|15.6 1982年|15.9 1981年|15.8 1980年|15.9 1979年|15.6 1978年|15 1977年|15. 1976年 14.6 1975年 14.6 1974年 14.8 1973年 14.8 1972年 15.6 1971年 17.2 1970年 18.4 1969年 17.9 1968年 17.6 1967年 17.8 1966年 18.4 1965年 19.4 1964年 21.1 1963年 21.7 1962年 22.4 1961年 23.3 1960年 23.7
表2:人口:16歳から50歳までの女性(16歳から50歳までの女性人口に占める割合)、過去12ヶ月以内に出生、データ.census.govによる
日付|アラバマ|アラスカ|アリゾナ|アーカンソー|カリフォルニア|コロラド|コネチカット|デラウェア|コロンビア特別区|フロリダ|ジョージア|ハワイ|アイダホ|イリノイ|インディアナ|アイオワ|カンザス|ケンタッキー|ルイジアナ|メイン|メリーランド|マサチューセッツ|ミシガン|ミネソタ|ミシシッピ|ミズーリ|モンタナ|ネブラスカ | ネバダ州 | ニューハンプシャー州 | ニュージャージー州 | ニューメキシコ州 | ニューヨーク州 | ノースカロライナ州 | ノースダコタ州 | オハイオ州 | オクラホマ州 | オレゴン州 | ペンシルベニア州 | ロードアイランド州 | サウスカロライナ州 | サウスダコタ州 | テネシー州 | テキサス州 | ユタ州 | バーモント州 | バージニア州 | ワシントン州 | ウェストバージニア州 | ウィスコンシン州 | ワイオミング州
2022年|52|64|56|55|50|50|44|48|42|49|52|58|58|52|56|58|62|54|57|52|53|45|53|5 8 | 56 | 56 | 57 | 63 | 52 | 47 | 52 | 56 | 49 | 52 | 67 | 57 | 59 | 47 | 52 | 47 | 55 | 66 | 53 | 58 | 61 | 43 | 50 | 54 | 5 0 | 52 | 64 | 28 2021 | 52 | 63 | 56 | 56 | 49 | 50 | 44 | 46 | 41 | 50 | 52 | 60 | 59 | 52 | 54 | 58 | 62 | 53 | 55 | 51 53 | 46 | 53 | 59 | 56 | 57 | 55 | 63 | 52 | 48 | 52 | 56 | 48 | 52 | 67 | 56 | 59 | 49 | 52 | 47 | 56 | 64 | 53 | 58 | 63 | 39 | 50 | 55 | 49 | 53 | 63 | 28 2020 | 53 | 63 | 56 | 57 | 50 | 51 | 43 | 46 | 40 | 49 | 53 | 62 | 61 | 53 | 55 | 61 | 6 0 | 54 | 57 | 51 | 53 | 46 | 54 | 60 | 56 | 58 | 55 | 64 | 52 | 47 | 51 | 57 | 49 | 52 | 68 | 57 | 61 | 49 | 53 | 49 | 56 68|52|58|66|42|51|56|53|54|66|31 2019|54|64|56|58|50|52|43|47|44|49|54|63|64 53、55、61、60、54、57、51、53、46、54、60、53、57、57、65、53、50、50、55、48、52、71、57、61、4 9 | 53 | 48 | 56 | 68 | 52 | 59 | 69 | 45 | 52 | 55 | 53 | 54 | 61 | 32 2018年 | 54 | 64 | 56 | 60 | 50 | 54 | 46 | 48 | 4 5 | 49 | 54 | 63 | 63 | 53 | 56 | 62 | 60 | 56 | 56 | 50 | 52 | 46 | 54 | 60 | 54 | 56 | 55 | 67 | 53 | 51 | 49 | 56 | 48 | 5 2017年 2月 2日 2017年 5月 2日 2017年 6月 2日 2017年 5月 11日 2017年 5月 18日 2017年 5月 25日 2017年 6月 1日 2017年 6月 8日 2017年 6月 15日 2017年 6月 22日 2017年 6月 29日 2017年 7月 6日 2017年 7月 13日 2017年 7月 20日 2017年 7月 27日 2017年 8月 3日 2017年 8月 10日 20 54 | 45 | 50 | 48 | 49 | 55 | 63 | 63 | 52 | 55 | 61 | 61 | 55 | 56 | 50 | 52 | 47 | 54 | 59 | 54 | 56 | 56 | 66 | 53 | 5 2016年 53 61 | 56 | 59 | 52 | 54 | 45 | 52 | 48 | 49 | 56 | 64 | 62 | 52 | 57 | 60 | 62 | 55 | 55 | 47 | 52 | 46 | 53 | 59 | 55 | 55 | 54 | 65 | 53 | 50 | 50 | 57 | 48 | 52 | 70 | 54 | 60 | 50 | 51 | 47 | 53 | 69 | 54 | 61 | 75 | 43 | 54 | 55 | 48 | 53 | 6 0 2015 55 65 56 58 53 56 46 52 46 49 56 65 66 52 57 59 64 55 55 47 53 46 | 53 | 60 | 53 | 55 | 56 | 66 | 54 | 48 | 51 | 60 | 49 | 54 | 70 | 54 | 59 | 51 | 51 | 47 | 55 | 67 | 55 | 61 | 75 | 42 | 5 55 | 47 | 52 | 60 | 40 2014年 | 56 | 66 | 57 | 56 | 54 | 57 | 48 | 53 | 46 | 49 | 57 | 65 | 67 | 53 | 56 | 58 | 64 | 55 56 | 48 | 53 | 47 | 54 | 60 | 57 | 57 | 57 | 65 | 57 | 49 | 51 | 60 | 50 | 55 | 66 | 54 | 60 | 53 | 50 | 46 | 55 | 68 | 57 | 62 | 77 | 42 | 55 | 56 | 47 | 53 | 59 | 42 2013年 | 57 | 70 | 59 | 57 | 55 | 58 | 48 | 55 | 45 | 51 | 58 | 65 | 70 | 55 58|59|66|56|58|48|55|48|54|59|59|57|58|66|59|48|52|61|51|56|66|55|61|53|51 | 47 | 57 | 68 | 60 | 64 | 78 | 42 | 55 | 56 | 47 | 54 | 61 | 43 2012年 | 60 | 74 | 61 | 58 | 57 | 59 | 50 | 53 | 42 | 52 60|66|73|56|58|61|66|57|60|48|56|49|53|61|62|58|60|66|61|47|55|62|51|58|65| 5 6 | 62 | 56 | 52 | 48 | 59 | 66 | 62 | 66 | 80 | 43 | 55 | 59 | 50 | 56 | 64 | 45 2011年 | 60 | 76 | 63 | 59 | 58 | 59 51 49 44 52 59 64 76 57 59 60 66 58 61 49 56 49 54 61 62 57 60 66 62 46 56 6 4 | 52 | 58 | 62 | 56 | 62 | 56 | 52 | 50 | 59 | 66 | 62 | 66 | 80 | 44 | 54 | 59 | 47 | 56 | 60 | 46 2010年 | 58 | 76 | 64 | 60 | 58 | 59 | 51 | 52 | 46 | 53 | 59 | 60 | 75 | 58 | 59 | 62 | 65 | 58 | 61 | 49 | 58 | 49 | 55 | 62 | 64 | 56 | 61 68 | 62 | 46 | 56 | 62 | 52 | 58 | 60 | 56 | 61 | 57 | 52 | 49 | 58 | 66 | 63 | 67 | 83 | 45 | 54 | 60 | 49 | 57 | 62 | 46
結果はどちらも日本語の入力を正しく理解して、データに基づき適切な回答を生成してくれました。
ただ、どちらも中途半端に日本語で回答が生成されており、途中から英語になっています。
特にRIGの方は、最初に日本語から英語に翻訳してから英語のプロンプトを改善しているようですが、最終的な入力は日本語で行われています。
今回は正しく理解してくれましたが、まだ動作が不安定な部分があるので、基本的には英語のプロンプトを用いるのが最適でしょう。
今回の検証の結果、DataGemmaは入力した質問に対して、関連するデータをもとに回答を生成してくれることが確認できました。
ただ、その正確性や説得力は高くなく、まだまだ改善の余地があると感じます。
また、今回比較検証した限りでは、RAGのほうがRIGよりも説得力のある回答を生成する印象です。
今後の発展に期待しましょう!
DataGemmaの未来と可能性
DataGemmaは、先進的なデータ解析技術とユーザーフレンドリーなインターフェースを備えたプラットフォームとして、さまざまな分野での活用が期待されています。以下に、本記事の主要ポイントをまとめます。
- 高度な解析ツールとカスタマイズ可能なインターフェースにより、ユーザーは複雑なデータを直感的に理解・分析することが可能です。
- セキュリティとプライバシーへの強固な取り組みにより、信頼性の高いデータ管理が実現されています。
- スケーラビリティに優れ、小規模から大規模なデータセットまで柔軟に対応できる点が特徴です。
- 未来においては、技術革新の継続、市場拡大、ユーザーエクスペリエンスの向上、持続可能性と倫理の重視が期待されています。
- 活用の可能性は広範であり、ビジネスインテリジェンス、医療・ヘルスケア、教育、金融業界、環境保護、政府・公共セクターなど、多岐にわたります。
DataGemmaの将来的な発展により、データ解析の枠を超えて、社会全体の課題解決や持続可能な発展に貢献することが期待されます。
特に、ハルシネーション(幻覚)リスクが軽減されることから、金融業界や医療業界など様々な分野で活躍できる可能性があります。
技術の進化とともに、DataGemmaはユーザーにとってますます不可欠なツールとなるでしょう。
最後に
いかがだったでしょうか?
弊社では、
・マーケティングやエンジニアリングなどの専門知識を学習させたAI社員の開発
・要件定義・業務フロー作成を80%自動化できる自律型AIエージェントの開発
・生成AIとRPAを組み合わせた業務自動化ツールの開発
・社内人事業務を99%自動化できるAIツールの開発
・ハルシネーション対策AIツールの開発
・自社専用のAIチャットボットの開発
などの開発実績がございます。
まずは、「無料相談」にてご相談を承っておりますので、ご興味がある方はぜひご連絡ください。
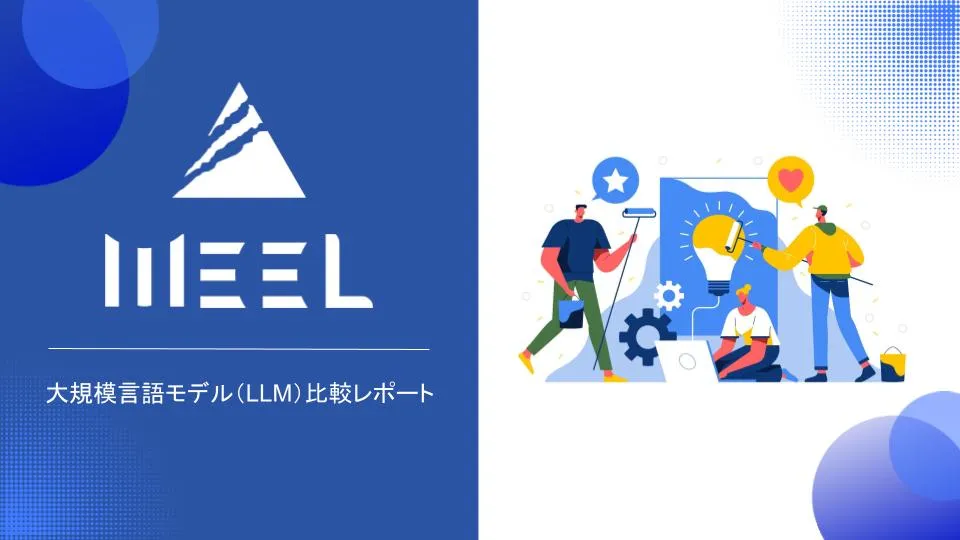
「生成AIを社内で活用したい」「生成AIの事業をやっていきたい」という方に向けて、生成AI社内セミナー・勉強会をさせていただいております。
セミナー内容や料金については、ご相談ください。
また、大規模言語モデル(LLM)を対象に、言語理解能力、生成能力、応答速度の各側面について比較・検証した資料も配布しております。この機会にぜひご活用ください。