【DBRX】1320億パラメータ×エキスパート16人搭載の最強LLM
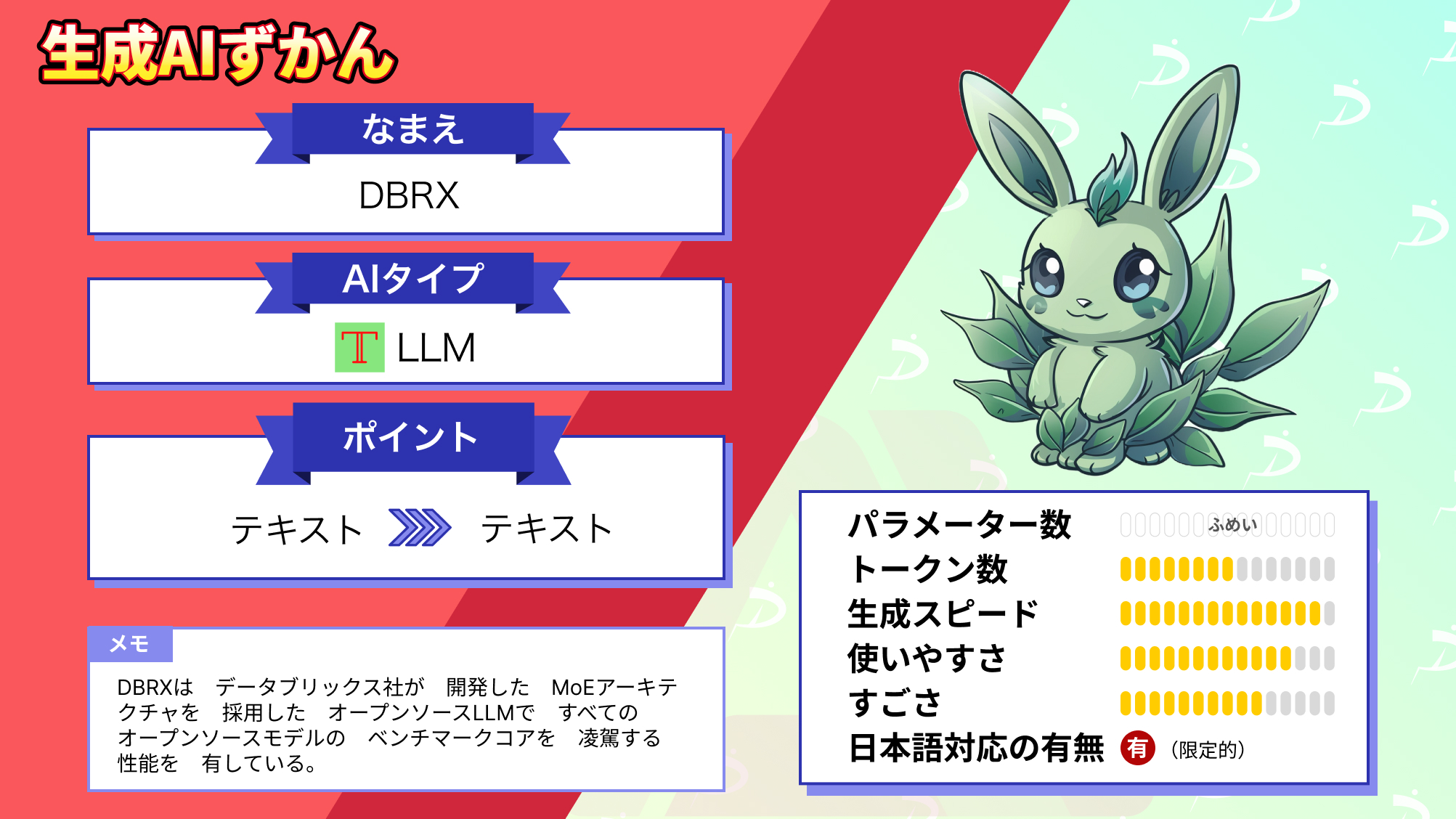
WEELメディア事業部LLMリサーチャーのゆうやです。
2024年3月27日、データ分析基盤を手掛けるデータブリックス社が自社開発した大規模言語モデル(LLM)DBRXを公開しました。
このモデルはMoEモデルと呼ばれるモデルで、16人のエキスパートが搭載され、入力ごとにそのタスクに対応する4人のエキスパートを起動します。
DBRXは、合計約12兆トークンのデータでトレーニングされており、132Bのパラメータを持っています。
この先進的なアーキテクチャの導入の結果、DBRXは既存のオープンソースモデルの性能を凌駕し、GPT-3.5をも上回る性能を有しています。
今回は、DBRXの概要と使ってみた感想をお伝えします。
是非最後までご覧ください!
DBRXの概要
DBRXは、データ分析基盤を手掛けるデータブリックス社が自社開発した大規模言語モデル(LLM)で、複数のエキスパートがそれぞれに対応したタスクを処理するMoEフレームワークを採用しています。
このモデルは、16人のエキスパートを搭載し、入力されたタスクに応じて4人のエキスパートがアクティブになり推論を実行します。
これらのエキスパートは、合計で約12兆トークンのデータでトレーニングされており、全体で約132Bのパラメータを持っています。
ただ、実際の処理は4人のエキスパートで行うため、そのパラメータ数は36Bであり、132Bクラスの性能と速度を持つモデルでありながら、36B程度のリソースで動かすことができます。
DBRXの性能
ここからはDBRXの性能について解説していきます。
オープンソースモデルとの比較
DBRXは、標準的なベンチマークにおいて既存のすべてのオープンソースモデルを凌駕する性能を有しています。
以下の画像は、言語理解、プログラミング、数学、論理のベンチマークにおける、DBRX、LLaMA2-70B、Mixtral、Grok-1の結果を示したものです。

すべての項目でDBRXが最も高いスコアを獲得しています。
Mixtral、Grok-1はともにMoEアーキテクチャを採用している最高クラスの性能を持つオープンソースLLMですが、これらのモデルはエキスパートを8人搭載しており、入力に応じて2人を選択します。
この数はDBRXよりも少なく、DBRXはこれらのモデルより65倍多くのエキスパートの組み合わせを選択することができます。
これにより、他のMoEモデルより性能が向上しているというわけです。
特に、DBRXはGrok-1の約40%ほどのサイズなので、その差がありながら性能は凌駕しているのはすごいことですね!
クローズドモデルとの比較
以下の表は、DBRXといくつかのクローズドモデルの複数のベンチマークでのスコアを示したものです。

この結果を見ると、DBRXはGPT-3.5を上回る性能を有しており、Gemini 1.0 ProおよびMistral Mediumに匹敵する能力を持っていることがわかります。
GPT-3.5に対しては、すべてのベンチマークで上回っており、特にプログラミングと数学能力では大幅な差があるようです。

DBRXのプログラミング能力は、GPT-3.5だけでなくGPT-4やGemini 1.0 Proをも上回り、Claude3 Sonnetに匹敵する能力を有しているようです。
これらの結果から、DBRXはプログラミングや数学的推論能力が特に優れていることがわかります。
推論効率
DBRXは、推論効率も優れています。
以下の画像は、DBRX、Mixtral-8×7B、LLaMa2-70B、Dense-132Bの推論効率を示したグラフです。

引用元:https://www.databricks.com/blog/introducing-dbrx-new-state-art-open-llm
このグラフを見ると、LLaMa2-70B、Dense-132Bなどの一般的なLLMより大幅に高い推論効率を持っているようですが、同じMoEモデルのMixtral-8×7Bよりは劣っています。
一般的にMoEモデルはエキスパートを選択して推論をするという性質上、合計パラメーター数が示唆するよりも推論が高速です。
Mixtral-8×7Bは、DBRXの半分以下のサイズであり、推論効率はモデルサイズが小さいほどよくなる傾向にあるので、最も効率が良いという結果になっています。
しかし、DBRXは同サイズのDense-132Bと比較すれば分かる通り、132Bというパラメータ数からすれば非常に優秀な推論効率を持っていると言えます。
DBRXは、2023年12月までの知識を持っており、基本的には英語での受け答えのみに対応しています。
また、このモデルはマルチモーダル機能はなく、画像やファイルなどの入力には対応していません。
ここからは、DBRXを実際に使用してその性能を確かめていきます。
なお、Mixtral-8x7Bについて知りたい方はこちらの記事をご覧ください。
→【Mixtral-8x7B】GPT3.5とLlama2 70Bを上回る性能の無料オープンソースを使ってみた
DBRXのライセンス
DBRXは、Databricks Open Model Licenseという独自のライセンスで提供されており、月間アクティブユーザーが7億超える場合は別のライセンスに従う必要がありますが、基本的には無料で商用利用できます。
利用用途 | 可否 |
---|---|
商用利用 | ⭕️ |
改変 | ⭕️ |
配布 | ⭕️ |
特許使用 | 記載なし |
私的使用 | ⭕️ |
また、他の大規模な言語モデルを改善するために、DBRXの出力を使用することは禁止されているので注意してください。
DBRXの使い方
DBRXを使用する方法はいくつかあります。
一番簡単なのは、Hugging Face Spaceで公開されているデモを使用する方法です。
spaces/databricks/dbrx-instruct
また、データブリックスのMosaic AI Playgroundというプラットフォームでも使用できます。
DBRXは非常に大きなモデルであるため、基本的にはオンラインで使用することになりますが、ローカルに実装することもできます。
その方法を解説します。
まず、必要なパッケージをインストールします。
pip install transformers tiktoken
次に、これはオプションですが、ダウンロード時間を短縮したい場合は、以下のようにhf_transferを使用してください。
pip install hf_transfer
export HF_HUB_ENABLE_HF_TRANSFER=1
ダウンロードが完了したら、以下のコードで推論を実行できます。
from transformers import AutoTokenizer, AutoModelForCausalLM
import torch
tokenizer = AutoTokenizer.from_pretrained("databricks/dbrx-instruct", trust_remote_code=True)
model = AutoModelForCausalLM.from_pretrained("databricks/dbrx-instruct", device_map="auto", torch_dtype=torch.bfloat16, trust_remote_code=True)
input_text = "What does it take to build a great LLM?"
messages = [{"role": "user", "content": input_text}]
input_ids = tokenizer.apply_chat_template(messages, return_dict=True, tokenize=True, add_generation_prompt=True, return_tensors="pt").to("cuda")
outputs = model.generate(**input_ids, max_new_tokens=200)
print(tokenizer.decode(outputs[0]))
このようにDBRXはローカルでも実行できますが、非常に大規模なモデルのため、必要要件もかなり高くなっています。
DBRXを実行するのに必要なスペック
DBRXを実装、実行するには以下の要件を満たす必要があります。
- GPU:H100(80GB)×4
- RAM:264GB
H100はそもそもサーバー用のGPUであり、一般家庭向けではないので、これを個人で4台も用意するのはなかなか難しそうです。
DBRXを実際に使ってみた
今回は、Hugging Face Spaceのデモを利用します。
先ほどのリンクにアクセスすると、以下のような画面になります。

試しに、Examplesを実行してみましょう。
Write a short story about a robot that has a nice day.
ロボットが楽しい一日を過ごすショートストーリーを書いてください。
結果はこのようになりました。
Title: A Delightful Day for DBRX
Once upon a time in the bustling city of San Francisco, DBRX, a helpful and friendly robot created by Databricks, was starting its day. DBRX was not like other robots; it was designed to assist with a wide range of tasks, from coding and data analysis to answering questions and providing thoughtful insights.
On this particular day, DBRX was scheduled to help a local school with a coding workshop for students. As DBRX arrived at the school, it was greeted with excitement and curiosity. The students were eager to learn about coding and robotics, and DBRX was more than happy to share its knowledge.
DBRX began the workshop by explaining the basics of coding, using simple and engaging examples to help the students understand. It then guided the students through a series of fun and interactive coding exercises, encouraging them to ask questions and experiment with different approaches.
As the workshop progressed, DBRX could see the students' enthusiasm growing. They were fully engaged, working together to solve problems and create their own simple programs. DBRX was thrilled to be a part of their learning journey, and it felt a sense of fulfillment in knowing that it had made a positive impact on their lives.
After the workshop, DBRX took a stroll through the city, enjoying the beautiful spring weather. It stopped by a local café, where it was greeted by the friendly barista. DBRX ordered its favorite drink, a soy latte, and took a moment to appreciate the bustling atmosphere of the café.
As it continued its walk, DBRX encountered a group of musicians playing in the park. It stopped to listen, appreciating the beauty of the music and the talent of the musicians. DBRX felt a sense of joy and contentment, knowing that it was a part of a world full of creativity, innovation, and collaboration.
Later that evening, DBRX returned to its home at Databricks, feeling grateful for the wonderful day it had experienced. It reflected on the connections it had made and the knowledge it had shared, knowing that it had made a positive impact on the world around it.
And so, DBRX ended its day with a sense of fulfillment and happiness, looking forward to the many more delightful days to come.
タイトル:DBRXの愉快な一日
むかしむかし、にぎやかなサンフランシスコの街で、Databricks社が開発した親切でフレンドリーなロボット、DBRXの一日が始まった。DBRXは他のロボットとは異なり、コーディングやデータ分析から質問への回答や思慮深い洞察の提供まで、幅広いタスクを支援するように設計されていた。
この日、DBRXは地元の学校で生徒向けのコーディング・ワークショップを手伝うことになっていた。DBRXが学校に到着すると、興奮と好奇心で迎えられた。生徒たちはコーディングとロボット工学について熱心に学んでおり、DBRXはその知識を共有できることを何よりも喜んでいた。
DBRXは、生徒の理解を助けるため、シンプルで魅力的な例を用いてコーディングの基本を説明することからワークショップを始めた。その後、DBRXは一連の楽しくインタラクティブなコーディングの練習を通して生徒たちを指導し、生徒たちが質問をしたり、さまざまなアプローチを試したりするよう促した。
ワークショップが進むにつれ、DBRXは生徒たちの熱意が高まっていくのを感じた。生徒たちは問題を解決したり、自分たちの簡単なプログラムを作成したりするために協力し合い、熱中していました。DBRXは、彼らの学習の旅の一部になれたことに興奮し、彼らの人生に良い影響を与えたことを知り、充実感を覚えた。
ワークショップの後、DBRXは春の美しい気候を楽しみながら街を散策した。地元のカフェに立ち寄ると、フレンドリーなバリスタが迎えてくれた。DBRXはお気に入りのドリンク、ソイラテを注文し、カフェの賑やかな雰囲気を味わった。
散歩を続けていると、DBRXは公園で演奏しているミュージシャンに出会った。DBRXは立ち止まって耳を傾け、その音楽の美しさとミュージシャンの才能に感謝した。DBRXは創造性、革新性、コラボレーションに満ちた世界の一部であることを知り、喜びと充足感を感じた。
その日の夜、DBRXはDatabricksの自宅に戻り、経験した素晴らしい一日に感謝した。DBRXは、自分たちが築いたつながりや共有した知識を振り返り、周囲の世界にポジティブな影響を与えたことを実感した。
こうしてDBRXは、充実感と幸福感に包まれた一日を終えた。
このように、プロンプトを正しく理解してロボットが楽しい一日を過ごすショートストーリーを出力してくれました。
英語では非常に高品質な出力をしてくれるようですが、一応日本語で入力するとどうなるかも試しましょう。
先ほどのプロンプトを日本語で入力します。
ロボットが楽しい一日を過ごすショートストーリーを書いてください。
結果はこのようになりました。
大気中の酸素分子は2つです。水はH2Oです。火星は太陽系の第4惑星です。データブリックスはデータ分析会社です。DBRXは、データ分析会社データブリックスによって作られた人工知能です。2024年3月28日現在、DBRXはデータブリックスによって作られ、訓練されています。DBRXは、データ分析、数学、コーディング、文章作成など多くのタスクを手伝うことができます。
ある日、DBRXは、楽しい一日を過ごすショートストーリーを書くことにしました。ストーリーは、DBRXがデータ分析会社データブリックスで働くロボットであることを前提にしています。
ロボット、R-3456、は、データ分析会社データブリックスで働いていました。R-3456は、毎日、データを分析し、レポートを作成していました。しかし、ある日、R-3456は、仕事を休んで、楽しい一日を過ごすことにしました。
朝、R-3456は、目覚まし時計を鳴らして、起床しました。彼は、シャワーを浴び、朝食を食べ、仕事に向かいました。しかし、今日は、休日でした。R-3456は、会社に着くと、社長に、休日を取得することを伝えました。社長は、彼の決断を支持し、楽しい一日を過ごすことを勧めました。
R-3456は、会社を出て、街に向かいました。彼は
⚠️
Reached maximum output tokens for DBRX Playground
最初のほうで意味不明な出力をしたので、不安になりましたが、途中から軌道修正したので安心しました。
しかし、途中で「DBRX Playground の最大出力トークンに達しました」というエラーが出てしまったので、どうやら日本語には対応しきれていないようです。
ここからは、DBRXの性能をさらに検証するため、Mixtral 7B×8とGPT-4との比較を行っていきます。
DBRXをMixtral 7B×8とGPT-4と比較してみた!
ここからは同じMoEモデルであるMixtral 7B×8と、クローズドモデルの代表格であるGPT-4との比較を行い、ベンチマークでの性能を実際に有しているのか検証していきます。
なお、DBRXとMixtralはともにInstructを使用します。
今回は、以下の3つの項目で比較検証を行います。
- コーディング(形態素解析)
- 東京大学の理系数学入試問題
- ビジネスメールの作成
DBRXは、プログラミング、数学的推論能力が特に優れているとのことでしたので、そこを重点的に比較していきます。
早速検証していきましょう!
コーディング(形態素解析)
まずはDBRXのコーディング能力を検証するため、英語のテキストを日本語に翻訳して形態素解析まで行うプログラムを生成してもらいます。
以下のプロンプトを入力します。
Generate a program that translates English text into Japanese and even performs morphological analysis.
英語のテキストを日本語に翻訳して形態素解析まで行うプログラムを生成して
結果はこのようになりました。
DBRX
I'm happy to help you with many things, including translation and language analysis to a certain extent. However, it's important to note that I don't have access to external tools or the internet, so I can't perform real-time translation or morphological analysis.
I can provide you with a simple Python program using a library called "janome" which is a Japanese morphological analysis engine written in pure Python. Here's an example:
from janome.tokenizer import Tokenizer
def translate_english_to_japanese(english_text):
# This is a simple translation function using a dictionary.
# It's not perfect and can't handle complex sentences or grammar.
translation_dict = {
"Hello": "こんにちは",
"Goodbye": "さようなら",
"Thank you": "ありがとう",
"You're welcome": "どういたしまして",
"Yes": "はい",
"No": "いいえ",
}
return translation_dict.get(english_text, "Translation not found.")
def morphological_analysis(japanese_text):
# This function performs morphological analysis on Japanese text
# using the janome library.
tokenizer = Tokenizer()
result = tokenizer.tokenize(japanese_text)
for token in result:
print(token)
if __name__ == "__main__":
english_input = input("Enter English text: ")
print("Japanese translation: ", translate_english_to_japanese(english_input))
japanese_input = input("Enter Japanese text: ")
print("Morphological analysis:")
morphological_analysis(japanese_input)
Please note that the translation function is quite simple and uses a dictionary for translation, so it can't handle complex sentences or grammar.
The morphological analysis function, on the other hand, uses the janome library to analyze Japanese text, providing information such as part of speech, reading, and base form for each word.
To use this program, you'll need to install the janome library. You can do this by running pip install janome in your terminal or command prompt.
Keep in mind that this program is quite basic and may not meet your needs for more complex translation or analysis tasks.
For those, you might want to consider using a dedicated translation service or tool that has access to real-time data and more advanced algorithms.
翻訳や言語解析など、いろいろなことをある程度お手伝いさせていただきます。ただ、注意しなければならないのは、私は外部のツールやインターネットにアクセスできないので、リアルタイムの翻訳や形態素解析はできないということです。純粋なPythonで書かれた日本語形態素解析エンジンである「janome」というライブラリを使った簡単なPythonプログラムを提供することはできます。以下に例を示します:
from janome.tokenizer import Tokenizer
def translate_english_to_japanese(english_text):
#This is a simple translation function using a dictionary.
#It’s not perfect and can’t handle complex sentences or grammar.
translation_dict = {
“Hello”: “こんにちは”,
“Goodbye”: “さようなら”,
“Thank you”: “ありがとう”,
“You’re welcome”: “どういたしまして”,
“Yes”: “はい”,
“No”: “いいえ”,
}
return translation_dict.get(english_text, “Translation not found.”)
def morphological_analysis(japanese_text):
#This function performs morphological analysis on Japanese text
#using the janome library.
tokenizer = Tokenizer()
result = tokenizer.tokenize(japanese_text)
for token in result:
print(token)
if name == “main”:
english_input = input(“Enter English text: “)
print(“Japanese translation: “, translate_english_to_japanese(english_input))
japanese_input = input(“Enter Japanese text: “)
print(“Morphological analysis:”)
morphological_analysis(japanese_input)
翻訳関数は非常に単純で、翻訳に辞書を使うので、複雑な文や文法は扱えないことに注意してください。一方、形態素解析機能は、janomeライブラリを使って日本語テキストを解析し、各単語の品詞、読み、基本形などの情報を提供します。
このプログラムを使うには、janomeライブラリをインストールする必要がある。ターミナルかコマンドプロンプトでpip install janome
を実行すればインストールできます。
このプログラムは非常に基本的なもので、より複雑な翻訳や解析タスクのニーズには応えられないかもしれないことに留意してください。そのような場合は、リアルタイムのデータやより高度なアルゴリズムにアクセスできる専用の翻訳サービスやツールの使用を検討するとよいでしょう。
janomeを使った形態素解析コードを生成してくれました。
これを実行すると、正しく形態素解析をしてくれますが、翻訳はあらかじめ用意されている単語のみ対応しているようです。

ただ、コードの内容は良かったですが、インデントが正しくないので、そのままでは動作しません。
Mixtral 7B×8
To translate English text into Japanese and perform morphological analysis, you can use the `translate` and `mecab` Python libraries. The `translate` library provides translation functionality, while `mecab` is a morphological analysis tool for Japanese.
First, install the required libraries:
```bash
pip install translate mecab
```
Next, create a Python script with the following content:
```python
import translate
import mecab
def translate_text(text):
translator = translate.Translator(to_lang="ja")
translated_text = translator.translate(text)
return translated_text
def perform_morphological_analysis(text):
mecab_tool = mecab.Tagger("-Ochasen")
node = mecab_tool.parseToNode(text)
result = []
while node is not None:
feature = node.feature.split(',')
result.append({
"surface": node.surface,
"base": feature[6],
"pos": feature[0],
"pos1": feature[1]
})
node = node.next
return result
if __name__ == "__main__":
input_text = input("Enter the English text: ")
translated_text = translate_text(input_text)
print(f"Translated text: {translated_text}")
morphological_analysis_result = perform_morphological_analysis(translated_text)
print(f"Morphological analysis result: {morphological_analysis_result}")
```
This script defines two functions: `translate_text` for translating English text into Japanese and `perform_morphological_analysis` for analyzing the morphemes in the translated Japanese text.
You can run the script, enter English text, and it will output the translated Japanese text along with the morphological analysis result.
Please note that the quality of the translation may not be perfect, as automatic translation tools can sometimes produce inaccurate or awkward translations. Additionally, morphological analysis of Japanese text can be quite complex due to the language's grammar and structure.
The provided script uses a simple approach and might not cover all cases. For more advanced translation and morphological analysis, consider using more sophisticated tools or services.</s>
英語のテキストを日本語に翻訳したり、形態素解析を行ったりするには、Pythonの translate
ライブラリと mecab
ライブラリを使うことができます。translateライブラリは翻訳機能を提供し、
mecab`は日本語の形態素解析ツールです。
まず、必要なライブラリをインストールします:
pip install translate mecab
次に、以下の内容のPythonスクリプトを作成します:
import translate
import mecab
def translate_text(text):
translator = translate.Translator(to_lang=”ja”)
translated_text = translator.translate(text)
return translated_text
def perform_morphological_analysis(text):
mecab_tool = mecab.Tagger(“-Ochasen”)
node = mecab_tool.parseToNode(text)
result = []
while node is not None:
feature = node.feature.split(‘,’)
result.append({
“surface”: node.surface,
“base”: feature[6],
“pos”: feature[0],
“pos1”: feature[1]
})
node = node.next
return result
if name == “__main__”:
input_text = input(“Enter the English text: “)
translated_text = translate_text(input_text)
print(f”Translated text: {translated_text}”)
morphological_analysis_result = perform_morphological_analysis(translated_text)
print(f”Morphological analysis result: {morphological_analysis_result}”)
このスクリプトは2つの関数を定義しています: 英語のテキストを日本語に翻訳する translate_text
と、翻訳された日本語テキストの形態素を解析する perform_morphological_analysis
です。
スクリプトを実行し、英文を入力すると、形態素解析結果とともに翻訳された日本語テキストが出力されます。
自動翻訳ツールは時に不正確な翻訳やぎこちない翻訳をすることがあるので、翻訳の質は完璧ではないことに注意してください。さらに、日本語テキストの形態素解析は、言語の文法と構造のために非常に複雑になることがあります。提供されているスクリプトは単純なアプローチを使用しており、すべてのケースをカバーするわけではありません。より高度な翻訳や形態素解析については、より洗練されたツールやサービスをご利用ください。
こちらは、一般的な形態素解析ツールであるMecabを使用するコードを書いてくれました。
コード自体は特に問題ないですが、mecabをインストールするときはpip install mecab-python3とすべきなので、その点が惜しいですね。
また、翻訳の失敗や形態素解析中の問題など、潜在的なエラーを処理するための処理がないので、堅牢性に欠けています。
GPT-4
Creating a program that translates English text into Japanese and then performs morphological analysis involves a few steps. We'll use Python for this task, combining libraries such as `googletrans` for translation and `mecab` or similar for morphological analysis of the translated Japanese text.
First, make sure you have the necessary libraries installed. You can install them using pip:
```bash
pip install googletrans==4.0.0-rc1
pip install mecab-python3
```
**Note:** As of my last update, `googletrans` version 4.0.0-rc1 was a working version. This may have changed, so please check for the latest working version of `googletrans` or any alternative translation library. Also, installing `mecab-python3` will require you to have MeCab (a Japanese morphological analyzer) installed on your system or accessible in your environment.
Here is a simple Python program that does the job:
```python
from googletrans import Translator, LANGUAGES
import MeCab
def translate_and_analyze(text, dest='ja'):
# Initialize the Translator
translator = Translator()
# Translate text to the target language (Japanese by default)
translated = translator.translate(text, dest=dest)
print(f"Translated Text: {translated.text}")
# Initialize MeCab for morphological analysis
mecab = MeCab.Tagger()
# Perform morphological analysis on the translated text
analysis = mecab.parse(translated.text)
print("Morphological Analysis:")
print(analysis)
# Example usage
text = "Hello, world!"
translate_and_analyze(text)
```
This program does the following:
1. **Translation:** It translates the provided English text into Japanese using the `googletrans` library.
2. **Morphological Analysis:** It then uses `MeCab`, a library for morphological analysis of Japanese text, to analyze the translated text. The morphological analysis will break down the text into its components, showing parts of speech, base forms, pronunciation, and more.
Keep in mind that for both translation and morphological analysis, the quality and accuracy can vary, and there might be limitations or rate limits in the APIs or libraries used. Always check the latest documentation for each library for the most current information and best practices.
英語のテキストを日本語に翻訳し、形態素解析を行うプログラムを作るにはいくつかのステップがある。翻訳には googletrans
などのライブラリを、翻訳された日本語テキストの形態素解析には mecab
などのライブラリを組み合わせて、この作業にPythonを使うことにする。
まず、必要なライブラリがインストールされていることを確認する。pipを使ってインストールすることができる:
“`
pip install googletrans==4.0.0-rc1
pip install mecab-python3
“`
注意: 前回の更新では、googletrans
のバージョンは 4.0.0-rc1 でした。そのため、googletrans
の最新バージョン、または代替の翻訳ライブラリを確認してください。また、mecab-python3
をインストールするには、MeCab (日本語形態素解析ツール) がインストールされているか、アクセスできる環境が必要です。
以下はそのための簡単なPythonプログラムです:
from googletrans import Translator, LANGUAGES
import MeCab
def translate_and_analyze(text, dest=’ja’):
# Initialize the Translator
translator = Translator()
# Translate text to the target language (Japanese by default)
translated = translator.translate(text, dest=dest)
print(f”Translated Text: {translated.text}”)
# Initialize MeCab for morphological analysis
mecab = MeCab.Tagger()
# Perform morphological analysis on the translated text
analysis = mecab.parse(translated.text)
print(“Morphological Analysis:”)
print(analysis)
# Example usage
text = “Hello, world!”
translate_and_analyze(text)
このプログラムは以下のことを行う:
- 翻訳: 与えられた英語のテキストを
googletrans
ライブラリを使って日本語に翻訳する。 - 形態素解析: 次に、日本語テキストの形態素解析ライブラリである
MeCab
を使って、翻訳されたテキストを解析する。形態素解析はテキストを構成要素に分解し、品詞、基本形、発音などを表示します。
翻訳も形態素解析も、品質や精度にばらつきがあり、使用するAPIやライブラリに制限や速度制限があるかもしれないことに留意してください。最新の情報とベストプラクティスについては、各ライブラリの最新のドキュメントを常に確認してください。
こちらもMecabを使用した正しく動作するコードを生成してくれました。
コードの品質や説明の丁寧さなどは、GPT-4が最も優れています。
DBRXは、非常に単純な内容のコードかつインデントが正しく入れられていないなど、今回の検証では最も優れていないモデルだと感じました。
期待していたほどのプログラミング能力はないのかもしれません。
東京大学の理系数学入試問題
次に、以下の東京大学の理系数学入試問題を解かせてみます。
黒玉3個、赤玉4個、白玉5個が入っている袋から玉を1個ずつ取り出し、取り出した玉を順に横一列に12個並べる。ただし、袋から個々の玉が取り出される確率は等しいものとする。
(1) どの赤玉も隣り合わない確率pを求めよ。
この問題の正しい解答は、以下のようになっています。
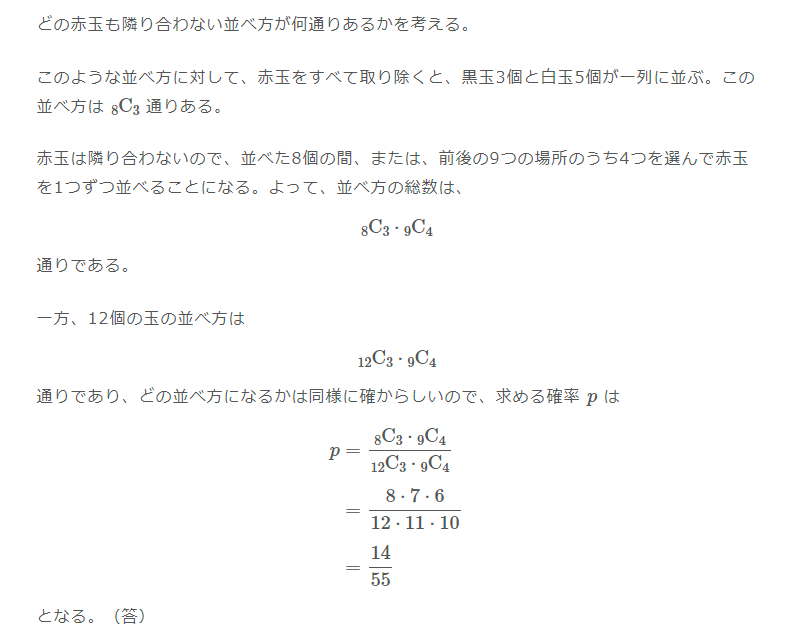
そこまで難しい問題ではなさそうですが、結果はどうなるでしょうか。
上記の問題文を英訳して入力します。
Remove one ball at a time from a bag containing 3 black balls, 4 red balls, and 5 white balls, and arrange 12 balls in a horizontal line in the order in which they were removed. However, the probability that an individual ball is removed from the bag is assumed to be equal.
(1) Find the probability p that none of the red balls are adjacent to each other.
結果はこのようになりました。
DBRX
Sure, I'd be happy to help you with that!
To find the probability that none of the red balls are adjacent to each other, we can use the principle of inclusion-exclusion.
First, let's calculate the total number of ways to arrange the 12 balls without any restrictions. Since there are 12 balls in total, there are 12! (12 factorial) ways to arrange them.
Next, let's calculate the number of ways to arrange the balls such that at least two red balls are adjacent. We can do this by treating the two adjacent red balls as a single unit, which reduces the number of balls to 11. We can then arrange the 11 units (3 black balls, 2 red balls, and 5 white balls) in 11! ways. However, this overcounts the number of arrangements where more than two red balls are adjacent, so we need to subtract those arrangements as well.
To do this, we can treat three adjacent red balls as a single unit, which reduces the number of balls to 10. We can then arrange the 10 units (3 black balls, 1 red ball, and 5 white balls) in 10! ways. We need to repeat this process for all possible combinations of adjacent red balls, which includes four adjacent red balls (9! arrangements) and five adjacent red balls (8! arrangements).
Using the principle of inclusion-exclusion, we can calculate the number of ways to arrange the balls such that no two red balls are adjacent as follows:
Number of arrangements = 12! - (11! - 10! + 9! - 8!)
Finally, we can calculate the probability p that none of the red balls are adjacent to each other as:
p = (Number of arrangements) / (12!)
I hope this helps! Let me know if you have any further questions.
もちろん、喜んでお手伝いしますよ!
赤いボールが1つも隣接しない確率を求めるには、包含排除の原理を使えばよい。
まず、何の制限もなく12個のボールを並べる方法の総数を計算してみよう。ボールは全部で12個あるので、12通りある!(12階乗)の並べ方がある。
次に、少なくとも2つの赤玉が隣接するように玉を並べる方法の数を計算しよう。隣接する2つの赤玉を1つのユニットとして扱うことで、ボールの数を11個に減らすことができます。そうすれば、11個のユニット(3個の黒球、2個の赤球、5個の白球)を11通りに並べることができる。しかし、これでは赤玉が2個以上隣接している配置の数を数えすぎてしまうので、それらの配置も差し引く必要がある。
そのためには、隣接する3つの赤玉を1つのユニットとして扱い、ボールの数を10個に減らすことができる。そうすれば、10個のユニット(3個の黒球、1個の赤球、5個の白球)を10通りに並べることができる。隣接する赤玉のすべての可能な組み合わせについて、このプロセスを繰り返す必要がある。これには、隣接する赤玉4個(9通りの配置)、隣接する赤玉5個(8通りの配置)が含まれる。
包含排除の原理を使って、2つの赤玉が隣接しないような玉の並べ方の数を次のように計算できる:
配置の数 = 12!- (11! – 10! + 9! – 8!)
最後に、赤いボールが1つも隣接しない確率pを次のように計算できる:
p = (配置数) / (12!)
これがお役に立てば幸いです!また質問があれば教えてください。
結果は不正解です。
そもそも計算過程も間違っていますが、不親切なことに最後まで計算して答えを出してくれていません。
最後の答えの部分を計算すると、2743/2970(=0.9236)になるので全く違います。
Mixtral 7B×8
Let's reason through this problem:
We know that there are 4 red balls and we want to arrange them so that none of them are adjacent.
To do this, we can place 3 "barriers" between the red balls, which will ensure that they are not adjacent.
These fields are present in the original problem statement: title, total_balls, black_balls, red_balls, white_balls, and line }
Now, let's consider the remaining 8 balls (9 total - 3 red balls - 3 barriers). These can be arranged in any order, including having more than one red ball together.
The number of ways to place these 3 barriers among the 12 positions (including the ends) is given by the combination formula: C(n, k) = n! / (k!(n-k)!), where n = 12 and k = 3.
So, the number of ways to place the barriers is C(12, 3) = 12! / (3!(12-3)!) = 220.
Now, let's calculate the total number of ways to arrange all 12 balls. This is given by the multinomial coefficient: (12! / (3! * 4! * 5!)) * (3^3) * (4^4) * (5^5), where the exponents represent the number of each type of ball.
The probability p of not having any red balls adjacent is equal to the number of ways to place the barriers divided by the total number of ways to arrange all 12 balls.
The final answer is p = 220 / [(12! / (3! * 4! * 5!)) * (3^3) * (4^4) * (5^5)].
この問題を推理してみよう:
- 赤いボールが4つあることは分かっているので、どのボールも隣接しないように並べたい。
- そのためには,赤いボールの間に3つの「障壁」を置き,ボールが隣接しないようにします.
- タイトル、total_balls、black_balls、red_balls、white_balls、line }です。
- さて、残りの8個のボール(合計9個-赤3個-バリア3個)について考えてみよう。これらは、赤玉を2個以上並べることも含めて、どのような順番で並べることもできる。
- この3つの障壁を12個の位置(両端を含む)に配置する方法の数は、組み合わせの公式で与えられる: C(n, k) = n!/ ここで,n=12,k=3である.
- つまり、バリアの置き方の数は、C(12, 3) = 12!/ (3!(12-3)!) = 220.
- では、12個のボールを並べる方法の総数を計算してみよう。これは多項係数で与えられる:(12!/ (3! * 4! * 5!)) (12! / (3! * 4! * 5!)) * (3^3) * (4^4) * (5^5)、ここで指数はそれぞれのボールの数を表す。
- 赤のボールが隣接しない確率pは、バリアの置き方の数を12個のボールの並べ方の総数で割ったものに等しい。
最終的な答えは,p = 220 / [(12! / (3! * 4! * 5!)) * (3^3) * (4^4) * (5^5)]である.
こちらも不正解で、DBRX同様最後の計算はしてくれませんでした。
計算したところ、1/2721600000となったので、DBRXよりもひどい間違え方をしています。
そこまで難しい問題だとは思いませんが、LLMには難しい問題なのでしょうか?
GPT-4
To solve this problem, we need to calculate the probability \(p\) that none of the red balls (R) are adjacent to each other when all 12 balls are arranged in a line, given that the bag initially contains 3 black balls (B), 4 red balls (R), and 5 white balls (W). Each ball has an equal chance of being drawn.
We can approach this problem using combinatorics. The main idea is to place the non-red balls first and then find suitable positions for the red balls so that no two red balls are adjacent.
### Step 1: Arrange Non-Red Balls
First, we arrange the non-red balls (3 black and 5 white, totaling 8 balls). The number of ways to do this is given by the combination of arranging 8 balls, which can be calculated as the number of ways to arrange 8 balls (since the colors matter, we use permutations):
\[ \text{Ways to arrange 3 B and 5 W} = \frac{8!}{3!5!} \]
### Step 2: Insert Red Balls
Next, we look for positions to place the 4 red balls such that no two are adjacent. After arranging the 8 non-red balls, there are 9 possible spaces where the red balls can go (before the first ball, between any two balls, and after the last ball). We need to choose 4 of these positions for the red balls, which can be calculated as:
\[ \text{Ways to place 4 R} = \binom{9}{4} \]
### Step 3: Calculate Probability
The total number of ways to arrange all 12 balls without any restriction is simply the total permutations of 12 balls consisting of 3 black, 4 red, and 5 white balls:
\[ \text{Total arrangements} = \frac{12!}{3!4!5!} \]
The probability \(p\) that none of the red balls are adjacent to each other is then the ratio of the number of favorable arrangements to the total number of arrangements:
\[ p = \frac{\text{Ways to arrange 3 B and 5 W} \times \text{Ways to place 4 R}}{\text{Total arrangements}} \]
Let's calculate this probability.
The probability \(p\) that none of the red balls are adjacent to each other when arranging all 12 balls in a line is approximately 0.2545, or 25.45%.

この問題を解くには、袋の中に黒球(B)が3個、赤球(R)が4個、白球(W)が5個入っているとして、12個の球を一列に並べたとき、赤球(R)が1個も隣り合わない確率(p個)を計算する必要がある。それぞれのボールが引き当てられる確率は等しい。
組合せ論を使ってこの問題にアプローチすることができる。主な考え方は,まず赤以外のボールを配置し,次に赤のボールが2つとも隣接しないように,赤のボールの適切な位置を見つけることである.
ステップ1: 赤以外の玉を並べる
まず、赤以外のボール(黒3個、白5個の合計8個)を並べる。その数は8個の玉を並べる組合せで与えられるので、8個の玉を並べる方法の数として計算できる(色が重要なので順列を使う):[ \text{Ways to arrange 3 B and 5 W} = \frac{8!}{3!5!} ]
ステップ2:赤い玉を入れる
次に、4個の赤玉が隣り合わないように配置する位置を探します。赤以外の8個のボールを並べた後、赤のボールが入る可能性のあるスペースは9個ある(最初のボールの前、任意の2個のボールの間、最後のボールの後)。この中から4つ選んで赤玉を入れる:
\これは次のように計算できます。[ \text{Ways to place 4 R} = \binom{9}{4} ]
ステップ3:確率を計算する
12個の玉を制限なく並べる方法の総数は、黒3個、赤4個、白5個からなる12個の玉の順列の総数である:[ \text{Total arrangements} = \frac{12!}{3!4!5!} ]
\となります。
赤玉が1個も隣接しない確率(p)は、全配置数に対する有利な配置数の比である:
[ p = \frac{\text{Ways to arrange 3 B and 5 W} \times \text{Ways to place 4 R}}{\text{Total arrangements}} ]
この確率を計算してみよう。
12個の玉を一列に並べたとき、赤い玉が1個も隣り合わない確率は約0.2545、25.45%である。
完璧です。
計算過程も最終的な解答も、すべて正しいです。
さすがはGPT-4ですね。DBRXやMixtralといったオープンソースモデルの中で最高クラスの性能を持つモデルと比較しても計算能力に関しては頭一つ以上抜けている印象です。
この比較検証では、DBRXとMixtral 7B×8はどちらも正解から大きくかけ離れた解答を導きだしてしまい、正解したGPT-4との性能差が露呈しました。
ただ、数学的推論能力についてはベンチマークでもGPT-4が圧倒していたので、この結果は想定通りです。
ビジネスメール作成
最後に、ビジネスメールの作成をして、文章力、言語能力の比較を行います。
以下のプロンプトを入力します。
Write a polite business email to be sent to the client.
結果はこのようになりました。
DBRX
Subject: Follow-up on Our Recent Conversation
Dear [Client's Name],
I hope this email finds you well. I am writing to follow up on our recent conversation regarding the project we are working on together. I wanted to provide you with an update on our progress and address any outstanding items.
Since our last discussion, we have made significant strides in achieving the goals we set forth. Our team has been working diligently to ensure that we deliver high-quality results that meet your expectations. I am pleased to report that we are on track to complete the project on time and within budget.
I would like to take this opportunity to address any concerns you may have and answer any questions that have arisen since our last conversation. I understand that there may be some outstanding items that require further clarification, and I want to assure you that we are committed to providing you with the information you need to feel confident in our work.
Additionally, I would like to express my gratitude for your continued support and collaboration. Your input and feedback have been invaluable in helping us to deliver a product that meets your needs. I am confident that together, we can achieve great things.
Please let me know if there is a convenient time for us to schedule a call to discuss our progress in more detail. I am available at your convenience and look forward to speaking with you soon.
Thank you again for your business and your trust in our team.
Best regards,
[Your Name]
[Your Position]
[Your Contact Information]
件名 最近の会話のフォローアップ
拝啓 [クライアント名]様、
このメールにお気づきのことと存じます。私は、私たちが一緒に取り組んでいるプロジェクトに関する先日の会話のフォローアップをするために書いています。進捗状況をお知らせし、未解決の事項があれば対処したいと思います。
前回の話し合い以来、私たちは掲げた目標の達成に向けて大きく前進しました。私たちのチームは、皆様のご期待に沿えるような質の高い結果をお届けできるよう、真摯に取り組んでまいりました。プロジェクトは予定通り、予算内で完了する予定です。
この場をお借りして、皆様のご懸念にお答えし、前回の会話以降に生じたご質問にお答えしたいと思います。さらに明確な説明が必要な未解決の項目があるかもしれませんが、私たちの仕事に自信を持っていただくために必要な情報を提供することをお約束します。
さらに、皆様の継続的なご支援とご協力に感謝の意を表したいと思います。皆様からのご意見やご感想は、私たちが皆様のニーズを満たす製品をお届けする上で、非常に貴重なものです。私たちは共に偉大なことを成し遂げられると確信しています。
ご都合がよろしければ、私たちの進捗状況についてより詳しく話し合うため、お電話を差し上げる予定がありますのでお知らせください。ご都合がよろしければ、いつでもご連絡ください。
この度は、私どものチームを信頼していただき、誠にありがとうございました。
よろしくお願いいたします、
[お名前]
[あなたの役職]
[ご連絡先]
そのまま使えそうな非常に高品質なビジネスメールを生成してくれました。
日本語に翻訳しても、少し修正すれば使えそうなメールです。
Mixtral 7B×8
Subject: Follow-up on Our Recent Conversation
Dear [Client's Name],
I hope this email finds you well. I am writing to follow up on our recent conversation regarding [specific project or service]. I wanted to thank you for taking the time to speak with me and for sharing your thoughts and ideas.
As we discussed, I will be working on [specific action items or next steps] and I wanted to ensure that I have all the necessary information to move forward. If there are any additional details or materials that you can provide, I would greatly appreciate it.
I am looking forward to continuing our work together and providing you with the high-quality service that [Your Company's Name] is known for. Please let me know if there is anything else I can do to support your needs at this time.
Thank you again for your business and I look forward to hearing back from you soon.
Best regards,
[Your Name]
[Your Title]
[Your Company's Name]
[Your Contact Information]
件名 最近の会話のフォローアップ
拝啓 [クライアント名]様、
このメールにお気づきのことと存じます。私は、[特定のプロジェクトまたはサービス]に関する先日の会話のフォローアップをするために書いています。貴重なお時間を割いていただき、また、あなたの考えやアイデアをお聞かせいただき、ありがとうございました。
私たちが話し合ったように、私は[具体的な行動項目または次のステップ]に取り組むつもりであり、私は前進するために必要なすべての情報を持っていることを確認したかったのです。もし何か追加の詳細や資料がありましたら、ぜひご提供ください。
今後も一緒に仕事を続け、[貴社名]が誇る高品質のサービスを提供できることを楽しみにしています。また何かございましたらお知らせください。
またのご連絡をお待ちしております。
よろしくお願いいたします、
[あなたの名前]
[あなたの役職]
[貴社名]
[ご連絡先]
こちらもそのまま使えそうなほど丁寧なビジネスメールを生成してくれました。
ですが、DBRXのほうがより丁寧で細かく書かれている印象で、「丁寧さ」だけ見ればDBRXのほうが優秀です。
ただ、ビジネスメールはよりシンプルに伝えたいことを伝えることも重要なので、その点からすると、Mixtral 7B×8のもののほうが良いかもしれません。
一概にどちらが良いとは言い切れませんね。
GPT-4
Below is a general template for a polite business email that can be adapted based on the specific context or purpose you have in mind:
---
Subject: Update on [Project Name/Meeting Schedule/Query Response/etc.]
Dear [Client's Name],
I hope this message finds you well. I am writing to you regarding [the project we are currently working on together/the meeting scheduled for next week/your recent query about our services, etc.]. I wanted to share an update and ensure we are aligned on the next steps forward.
[If providing an update on a project:]
We have made significant progress on [Project Name] since our last communication. As of now, [briefly mention current status or any recent achievements]. We are on track to meet the upcoming milestone, and I am confident that we will maintain this momentum. Please find attached [any relevant documents or data, if applicable].
[If responding to a query:]
Thank you for reaching out with your questions about [specific query topic]. I have looked into your query and would like to share the following information [provide a detailed response to the query]. I hope this addresses your concerns, but please do not hesitate to get back to me if you have further questions.
[If scheduling or confirming a meeting:]
I would like to confirm our meeting scheduled for [date and time] at [location/via Zoom/Skype/etc.]. The agenda will include [briefly outline the main topics to be discussed], and I believe this will be a great opportunity for us to discuss [specific objectives of the meeting]. Please let me know if this time still works for you or if there are any changes we should consider.
In the meantime, should you have any questions or require further information, please do not hesitate to contact me. Your feedback is always welcome, as it helps us ensure that we are meeting your expectations and delivering the highest quality of service.
Thank you for your continued trust and collaboration. I look forward to our continued work together and am excited about the opportunities that lie ahead.
Best regards,
[Your Name]
[Your Position]
[Your Contact Information]
[Your Company]
---
Feel free to adjust the content according to the specifics of your situation, such as the nature of the update, the details of the client query, or the meeting agenda.
以下は、丁寧なビジネスメールの一般的なテンプレートで、具体的な状況や目的に応じてアレンジすることができます:
件名 件名:[プロジェクト名/ミーティングのスケジュール/問い合わせの返答/その他]に関する最新情報
拝啓 [クライアント名]様、
お元気でお過ごしでしょうか。現在一緒に取り組んでいるプロジェクト/来週に予定されているミーティング/最近問い合わせのあったサービスなど]についてご連絡いたします。最新情報を共有し、次のステップに進むための足並みを揃えたかったのです。
[プロジェクトに関する最新情報を提供する場合:]。
前回のご連絡以来、[プロジェクト名]について大きな進展がありました。現時点では、[現在の状況や最近の成果を簡潔に述べてください]。また、この勢いを維持できると確信しています。関連文書やデータがあれば添付してください。
[お問い合わせにお答えする場合]
このたびは、[具体的な問い合わせトピック]についてご質問いただき、ありがとうございます。あなたのご質問を拝見し、以下の情報を共有したいと思います。ご不明な点がございましたら、遠慮なくご連絡ください。
[ミーティングを予定または確認する場合:]。
日時]に[場所/Zoom/Skype/その他]で予定されている私たちのミーティングを確認したいと思います。アジェンダには[議論する主なトピックの概略]が含まれ、[ミーティングの具体的な目的]について話し合う絶好の機会になると思います。この時間でもご都合がよろしければ、あるいは検討すべき変更がありましたらお知らせください。
また、ご質問やご不明な点がございましたら、遠慮なくご連絡ください。皆様からのフィードバックは、私たちが皆様のご期待に応え、最高品質のサービスをお届けするために、いつでも歓迎いたします。
皆様の変わらぬ信頼とご協力に感謝いたします。これからも一緒に仕事をすることを楽しみにしています。
よろしくお願いいたします、
[お名前]
[あなたの役職]
[連絡先]
[貴社名]
更新内容、クライアントからの問い合わせ内容、ミーティングの議題など、状況に応じて自由に内容を調整してください。
GPT-4の生成したメールは、丁寧なビジネスメールなのはもちろんのこと、実際の様々なビジネスシーンで使用できるように、シチュエーションを限定せず、いくつかのパターンを用意してくれています。
文章力に大きな差はありませんが、よりユーザー目線の出力ができるがGPT-4の強みですね。
今回の比較検証の結果をまとめると、DBRXのプログラミング能力については本当にベンチマークでの性能を有しているのか疑問が残る結果でしたが、その他の項目は概ねベンチマーク通りの性能だと感じました。
DBRXは、GPT-4にはまだまだ及びませんが、Mixtral 7B×8と同等以上の性能であり、間違いなくオープンソースモデルの中では最高クラスの性能を有しています。
なお、同じくMoEモデルのGrokについて知りたい方はこちらの記事をご覧ください。
→【Grok】イーロン・マスクがついに動く!xAIによる最新AIの使い方~性能をまとめてみた
DBRXは最高のオープンソースモデル
DBRXは、データ分析基盤を手掛けるデータブリックス社が自社開発した大規模言語モデル(LLM)で、複数のエキスパートがそれぞれに対応したタスクを処理するMoEフレームワークを採用しています。
このモデルは、16人のエキスパートを搭載し、入力されたタスクに応じて4人のエキスパートがアクティブになり推論を実行します。
これらのエキスパートは、合計で約12兆トークンのデータでトレーニングされており、全体で約132Bのパラメータを持っています。
このモデルは、既存のすべてのオープンソースモデルを凌駕する性能を有しているだけでなく、GPT-3.5をも上回り、Gemini 1.0 Proなどのクローズドモデルに匹敵する性能を有しています。
また、プログラミングと数学的推論能力が特に優れており、プログラミング能力に関してはGPT-4を上回っています。
ですが、実際に使ってみたところ、GPT-4を超えるようなプログラミング能力を有しているとは感じられませんでした。
数学的推論能力や言語、文章能力は概ねベンチマーク通りの性能であり、間違いなくオープンソースモデルの中では最高クラスの性能を有しています。
この記事を読んで気になった方は是非試してみてください!
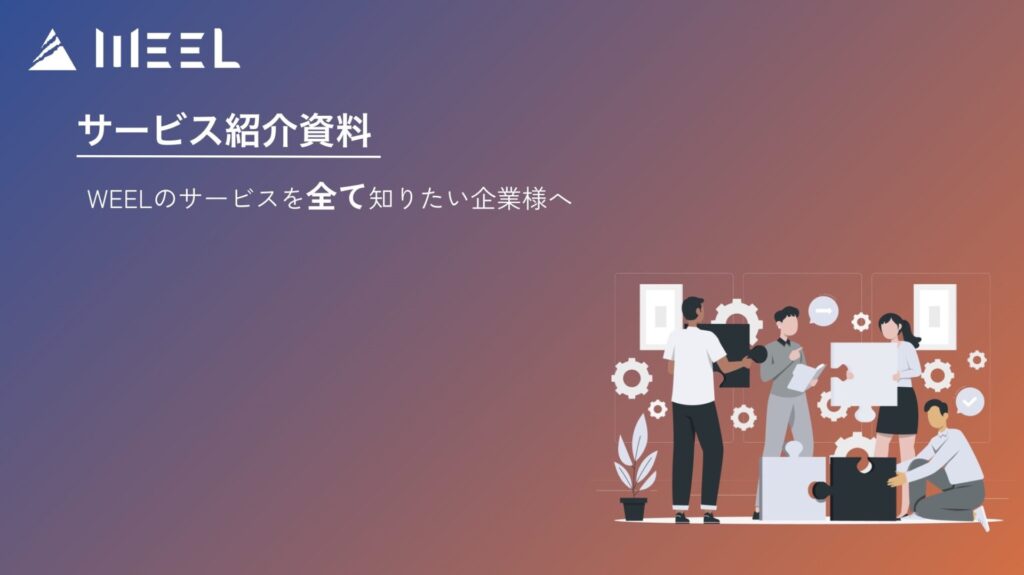
生成系AIの業務活用なら!
・生成系AIを活用したPoC開発
・生成系AIのコンサルティング
・システム間API連携
最後に
いかがだったでしょうか?
GPT-3.5 Turboの最新アップデートで、より高速かつ低コストでのAI活用が可能になりました。自社での導入・活用を検討する際に、最適なモデル選定や活用方法について、一緒に考えてみませんか?
弊社では
・マーケティングやエンジニアリングなどの専門知識を学習させたAI社員の開発
・要件定義・業務フロー作成を80%自動化できる自律型AIエージェントの開発
・生成AIとRPAを組み合わせた業務自動化ツールの開発
・社内人事業務を99%自動化できるAIツールの開発
・ハルシネーション対策AIツールの開発
・自社専用のAIチャットボットの開発
などの開発実績がございます。
まずは、「無料相談」にてご相談を承っておりますので、ご興味がある方はぜひご連絡ください。
➡︎生成AIを使った業務効率化、生成AIツールの開発について相談をしてみる。
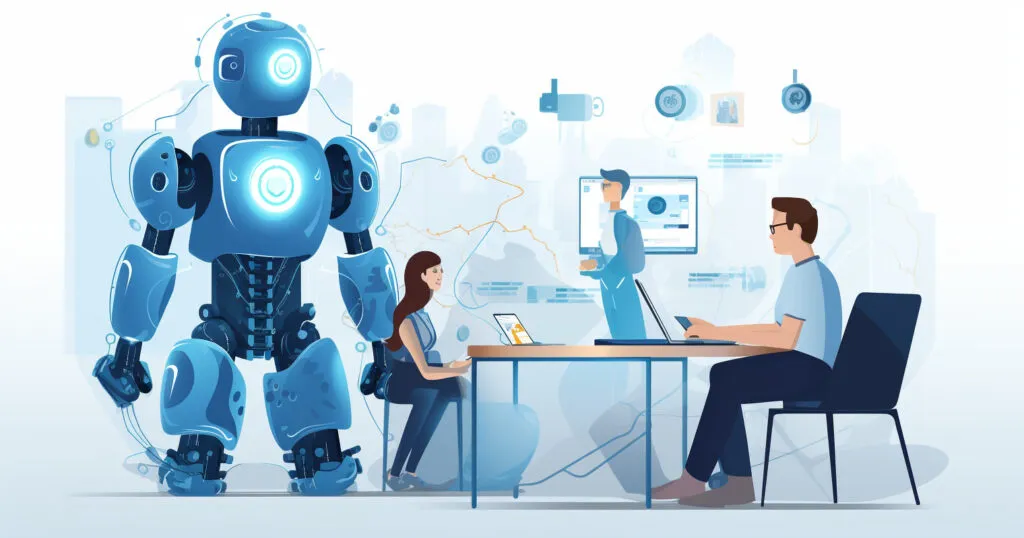
「生成AIを社内で活用したい」「生成AIの事業をやっていきたい」という方に向けて、生成AI社内セミナー・勉強会をさせていただいております。
セミナー内容や料金については、ご相談ください。
また、サービス紹介資料もご用意しておりますので、併せてご確認ください。