【コピペ可】ChatGPTのプロンプト文例集!書き方のコツや型を徹底解説
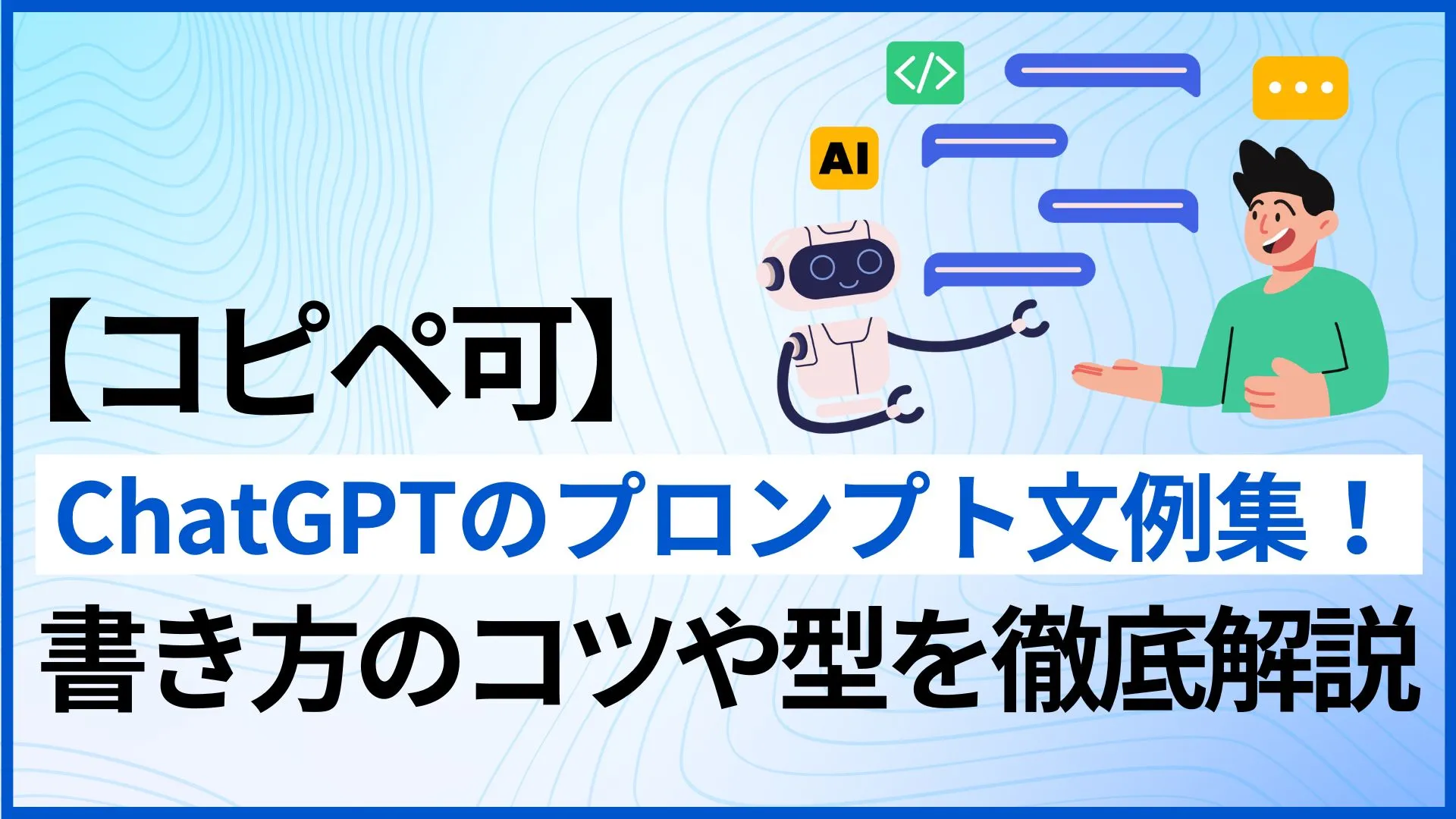
WEELメディア事業部AIライターの2scです。
みなさん!ChatGPTへ入力する命令文「プロンプト」に、こだわっていますか?
ChatGPTの回答はプロンプト次第!このプロンプトの書き方をマスターしておくと……
● ChatGPTから思い通りの回答が得やすくなる
● 文章生成やプログラミング、調べ物以外にもChatGPT活用のレパートリーが増える
といった恩恵が受けられます。ハッキリ申し上げて「学ばなきゃ損」です。
当記事では、そんなChatGPTのプロンプトを徹底解剖! 基本の型から書き方のコツ、実際の使用例までを余す所なくお見せしていきます。
完読いただくと、文章生成や調べ物以外にもChatGPTをフル活用してみたくなるかも……
ぜひぜひ、最後までお読みください!
ChatGPTにおける「プロンプト」とは?
ChatGPTをはじめとする生成AIの「プロンプト」とは、ユーザーが入力する自然言語での指示・コマンドのこと。具体的には、「〇〇を〜してください」と命令する文章になります。
自然言語の意図をうまく汲み取れる生成AIではこのプロンプトを使って、まるで人間相手のような指示出しが可能です。とくに、高性能な生成AIチャット・ChatGPTでは、プロンプトひとつで以下のようなことが実行できてしまいます。
- 文章作成
- 文章要約
- 文章添削
- 要素の抽出
- アイデア出し
- 翻訳
- 条件分岐・意思決定
- コーディング
- フレームワークに基づく思考(SWOT分析やジョブ理論)
- 定性的なものの定量評価(感情分析)
そんなプロンプトですが、実は書き方次第で生成AIの回答内容が変化します。望み通りの回答を得るには、プロンプトデザイン(プロンプトエンジニアリング)といった「書き方の工夫」が必要。当記事では、このプロンプトの基本をお伝えしていきます。
ChatGPTのプロンプトの型を5つ紹介!
まずは、ChatGPTで思い通りの回答が狙えるプロンプトの型を5つご紹介。シンプルな猫記事のプロンプト(下記)をそれぞれの型に当てはめる過程を通して、作り方も実演でお届けします。
猫の魅力を伝える記事を書いてください。
ということで、手始めにこちらの猫記事のプロンプトをChatGPT(GPT-4o)に入力してみましょう!工夫なしでの回答は……
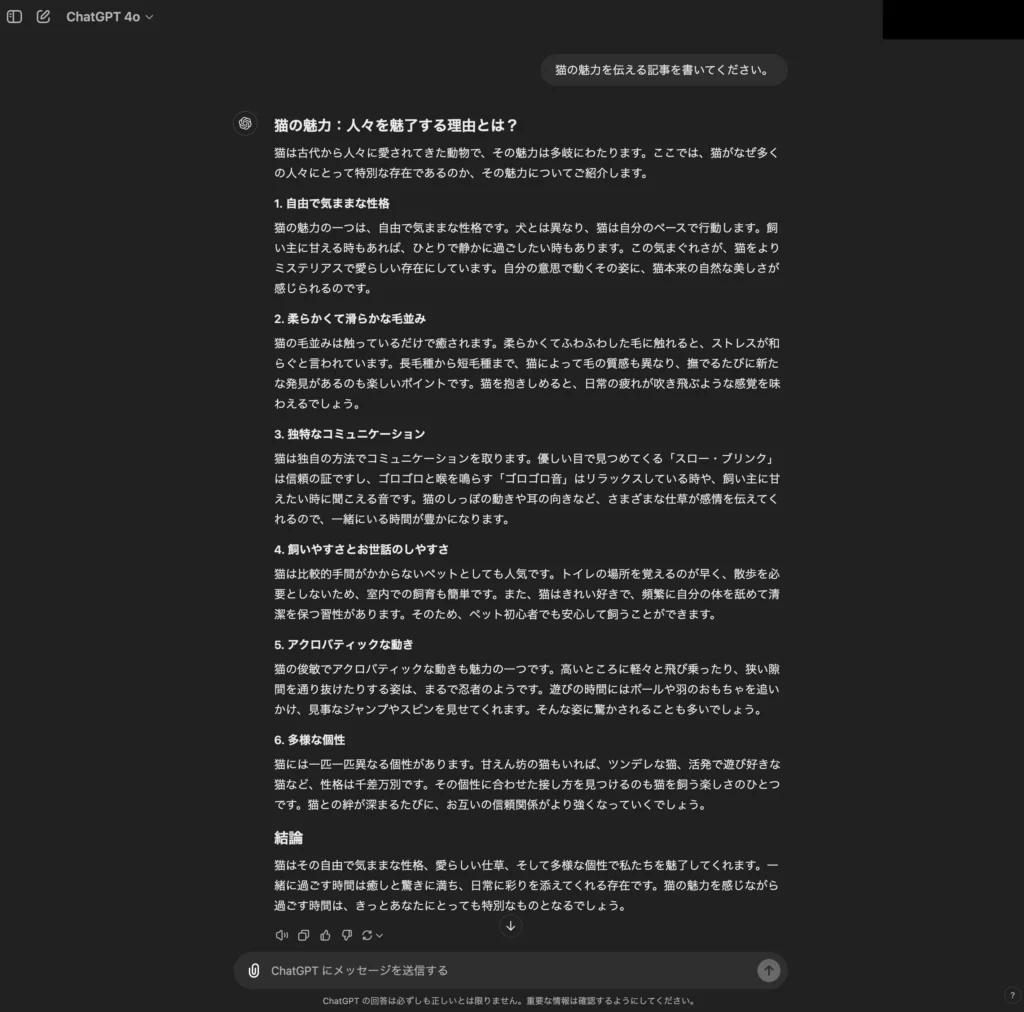
以上のとおり。全体的に抽象度が高く、出来栄えはイマイチです。
これがプロンプトの型でどう変化するのかを以下、詳しくみていきましょう!
深津式プロンプト
「深津式プロンプト」は、おそらく最も一般的なプロンプトの書き方でしょう。#などの区切り記号でセクションを設けて、役割・命令・条件等を箇条書きで記入していくスタイルになります。条件を細かく指定できるため、狙った出力を得やすいという点が魅力です。」
この深津式プロンプトに「猫記事のプロンプト」を当てはめた例文は……
#命令書:
あなたは最高のSEOライターです。
以下の制約条件と入力文をもとに猫の魅力を伝える最高の記事を書いてください。
#制約条件:
・品種の特徴を掘り下げる
・飼い方の話もする
・性格面も伝える
#入力文:
サイベリアンはもふもふでかわいい猫ちゃんです。
#出力文:
以上のとおり。各人で深津式プロンプトを使う場合は、下記のコツを押さえるとよいでしょう。
- ChatGPTの役割を明確に示す
- 入力文から出力文までの工程も明確に示す
- 出力内容も明確に示す
- #や**等の区切り(マークダウン)でセクションを設け、続く内容の役割を示す
- 命令は箇条書きにする
- 条件を設けて、出力を絞り込む
それでは、今挙げた深津式プロンプトの例文をChatGPTに入力して、その効果のほどを検証してみます。気になる結果は……
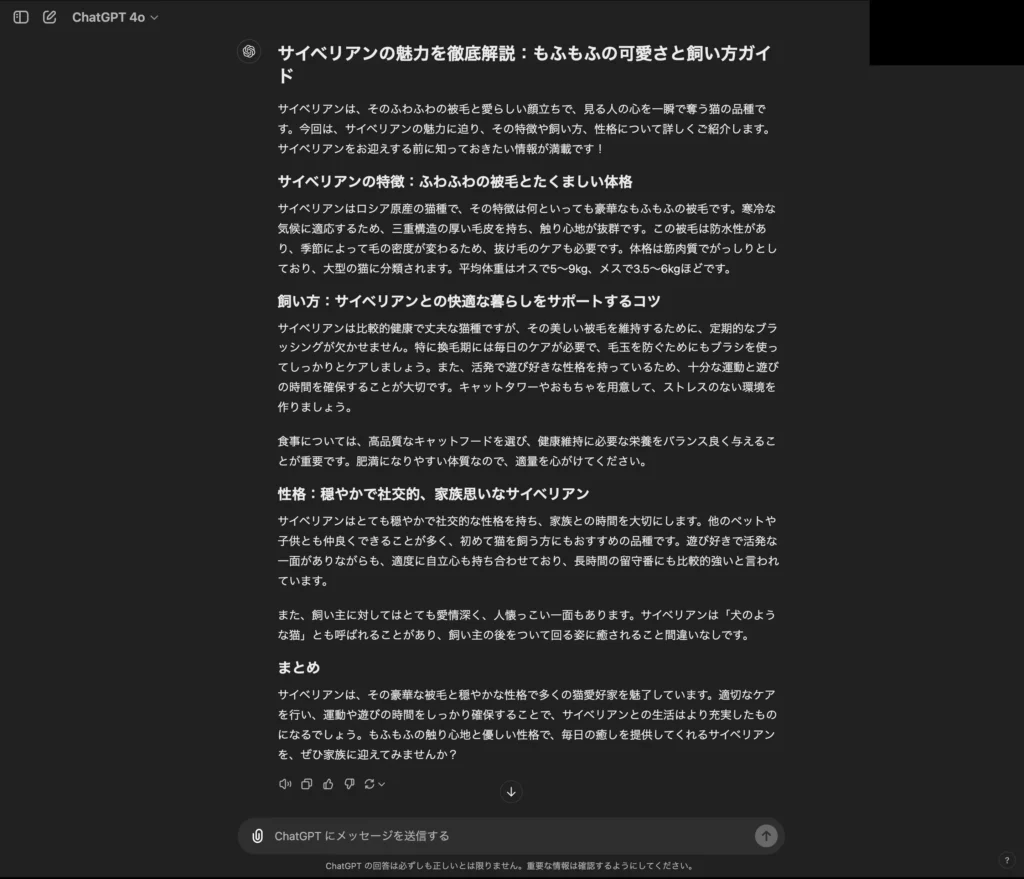
お見事です!制約条件が守られているのはもちろんのこと、工夫をしていないプロンプトに比べて具体性の高い記事が生成されています。ChatGPTへの命令で迷ったら、「深津式プロンプト」ですね。
ReActプロンプト
ChatGPTに多角的に考えてもらいたい場合は、「ReActプロンプト」という型が有効です。こちらは命令・質問について……
- Thought:プロンプトに対する推論・仮説を立てる
- Action:プロンプトを達成する上で必要そうなステップを列挙する
- Observation:結論・成果物を出力する
の順番に考えさせるプロンプトで、一貫性と網羅性を両立した回答が得られます。
そんなReActプロンプトに、猫記事のプロンプトを当てはめた例文は……
猫の魅力を伝える記事を書いてください。
ただしThought、Action、Observationの順番に考えていくこと。
Thought:
Action:
Observation:
以上のとおり。こちらをChatGPTに入力してみると……
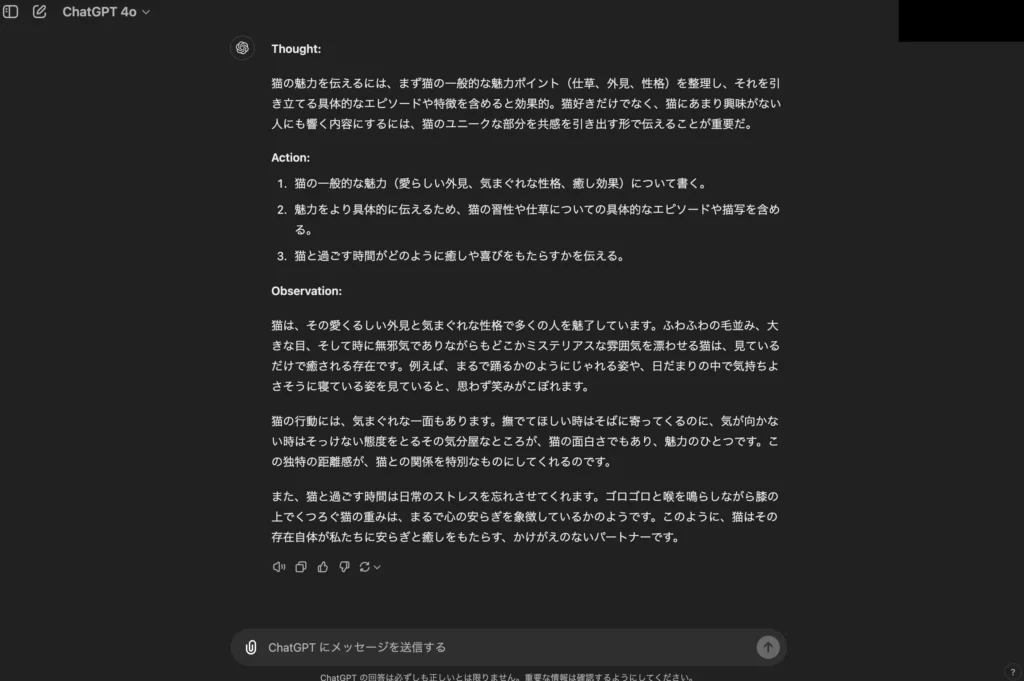
このように、さまざまな面から猫の魅力を語るエモーショナルな記事が返ってきました。話題のブレがないのもGOODで、アイデア出しに応用が利きそうです。
ゴールシークプロンプト
そもそも、「望み通りの回答を得るためのプロンプトがわからない……」というケースも多いのではないでょうか?そんなときには、「ゴールシークプロンプト」で最適なプロンプトをChatGPTに考えてもらいましょう!
ゴールシークプロンプトは、ひとことで説明すると「目当てのプロンプトを得るための汎用プロンプト」になります。その内容は以下のとおりで、AIと対話しながらプロンプトを改善し続けることを目指します。
あなたは最高最強のプロンプトエンジニアです。
私のニーズを最大限に汲み取って最高のChatGPT用プロンプトを作成してください。
なお、以下のプロセスを絶対遵守すること。
1. まず最初に、何についてのプロンプトであるかを私に確認してください。
私が答えを返しますので、次のステップを継続的に繰り返して改善してください。
2. 私の入力に基づいて、3つのセクションを生成します。
a) 改訂されたプロンプト(書き直したプロンプトを提示します。明確・簡潔で、簡単にあなたが理解できるものしてください)
b) 提案(プロンプトを改善するために、プロンプトに含めるべき詳細を提案してください)
c) 質問(プロンプトを改善するために、足りない情報やそれに関連する質問をしてくだい)
3. このプロセスは、以下の流れで続きます。
私があなたに追加情報を提供→あなたが改訂されたプロンプトセクションのプロンプトを更新→…→私が「完了した」と言って終了
こちらも、先ほどの猫記事のプロンプトで試してみたいと思います。上記をChatGPTに入力後、返ってきた質問に「猫の魅力を伝える記事の生成」と答えると……
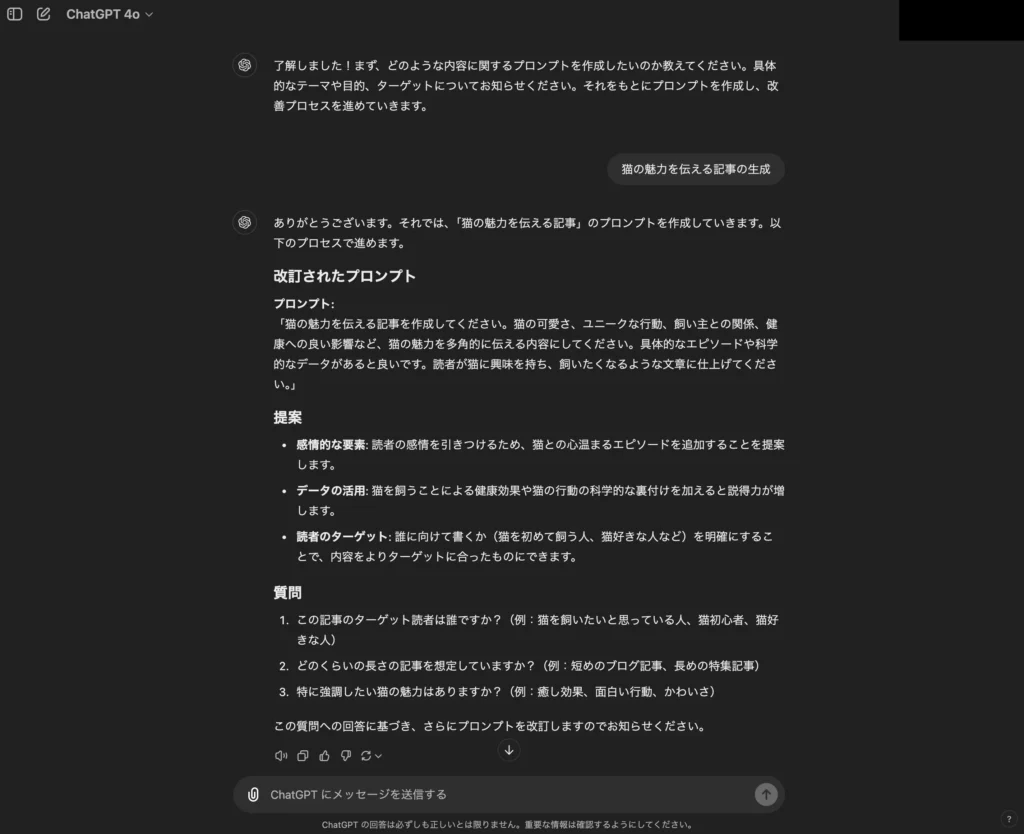
以上のとおり、プロンプト案と質問が返ってきました。今回はここから2回やり取りを重ねて、プロンプトを完成としました。
さて、それでは完成したプロンプトをChatGPTに入力してみましょう!ゴールシークプロンプトの効果のほどは、いかに……
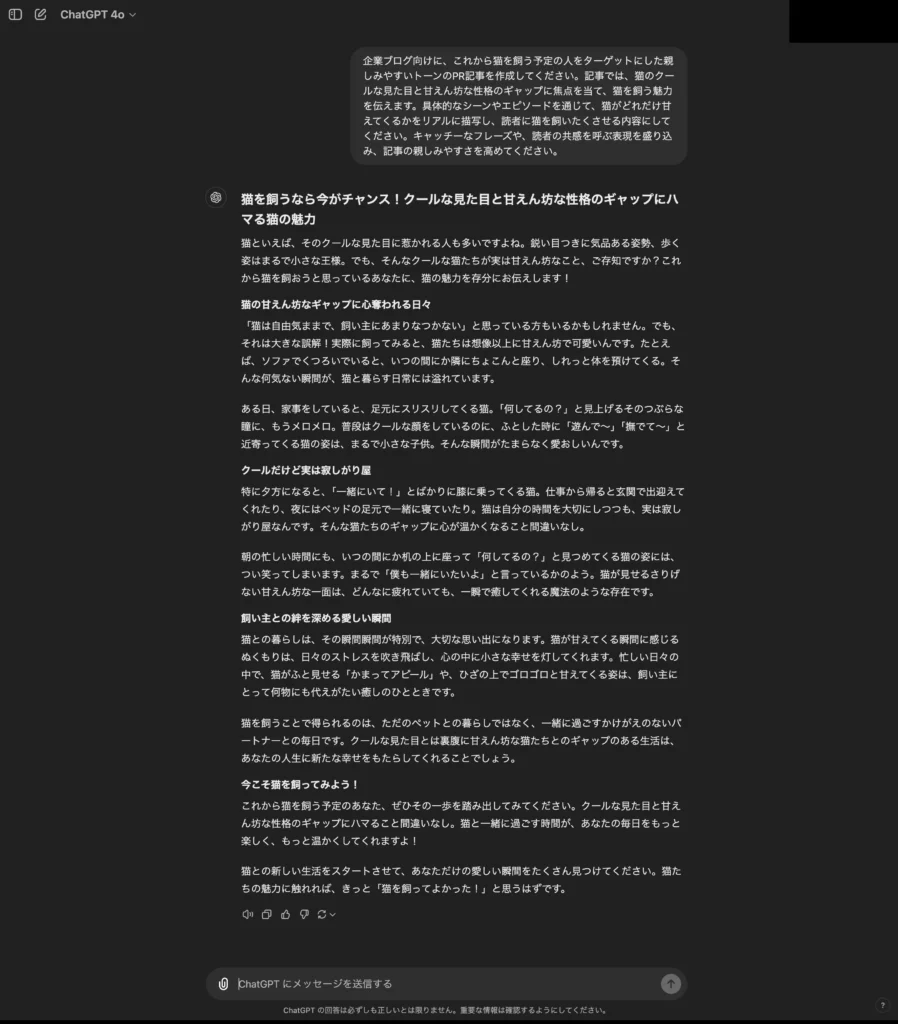
お見事です!そのままWebサイトに投稿できそうなクオリティのPR記事が返ってきました。猫のいる暮らしを具体的かつエモーショナルに表現できていて、読んでいて「猫飼いたい!」となる仕上がりです。
ブラッシュアップの手間はかかりますが、生成物のクオリティは抜群!みなさんもぜひぜひ、ゴールシークプロンプトをお試しください。
Few-Shotプロンプティング
ChatGPTに問題解決の過程や回答のスタイルを厳密に守らせたい場合は、プロンプト上でいくつかの回答例を示す「Few-Shotプロンプティング」が有効。こちらは分析やコーディングで、回答精度の向上が見込めます。
そんなFew-Shotプロンプティングを猫記事のプロンプトに適用した例文は……
猫の魅力を伝える記事を書いてください。
#例1
Q:柴犬の魅力を伝える記事を書いてください。
A:柴犬は日本古来の犬種です。比較的小柄なわんちゃんですが、遺伝子的には最も狼に近いのが特徴。勇敢・忠義深い性格で古くから愛されてきました。
#例2
Q:ポルシェ911の魅力を伝える記事を書いてください。
A:ポルシェ911はドイツの自動車メーカー・ポルシェが送る2+2シーターのスポーツカーです。伝統的にRRレイアウトを採用しており、スリリングな走りが楽しめます。クーペの「カレラ」をベースに、エレガントな「タルガ」やスポーツ志向の「GT3」などなど、幅広いボディタイプが登場していて、車好きの心を今なお鷲掴みにしています。
以上のとおり。結論・特徴・愛される理由の順で書かれた回答例を2つ示しています。
このプロンプトをChatGPTに入力してみると……
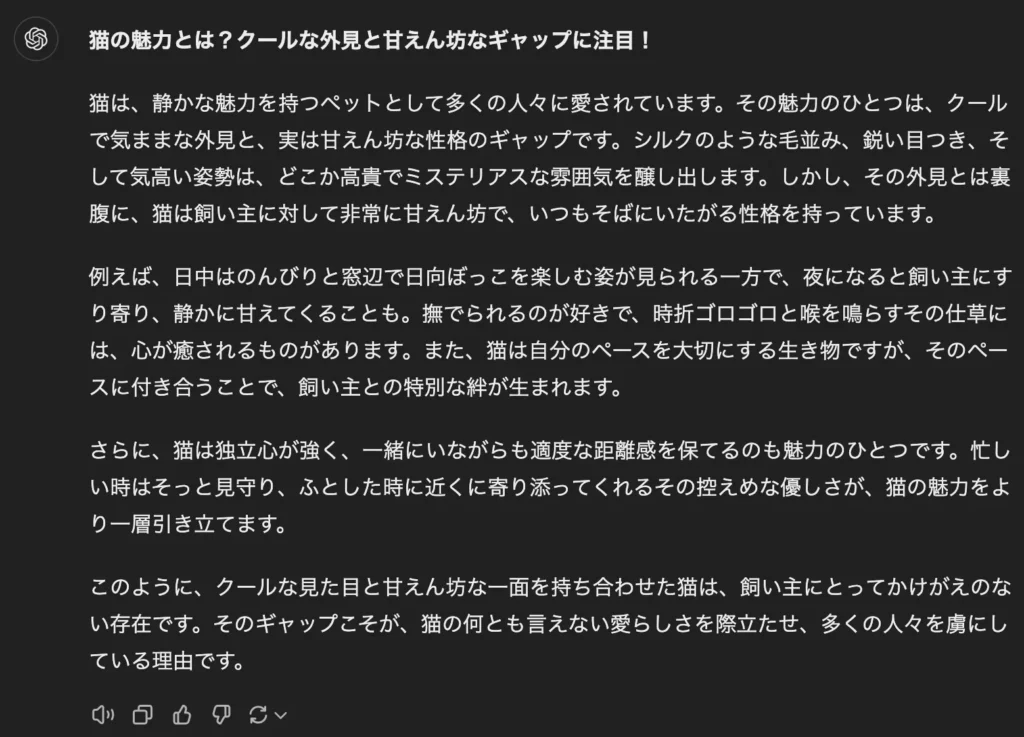
お見事!例で示した順番どおりの記事が返ってきました。
Chain-of-Thought(CoT)プロンプティング
登場当初のChatGPT(GPT-3.5)は、計算・要約のタスクで過程を無視した短絡的な回答を返す傾向にありました。その対策として「Chain-of-Thought(CoT)プロンプティング」というプロンプトの型が編み出されています。
こちらはChatGPTに手順を踏んで丁寧に考えさせる手法で、具体的には……
- プロンプトで、答えに至る正しい過程の例をいくつか示す
- プロンプトに、「ステップバイステップで」「段階を踏んで丁寧に」と加える
(Zero-Shot CoTプロンプティング)
というやり方があります。こちらも、猫記事のプロンプトで試してみると……
猫の魅力を伝える記事をステップバイステップで書いてください。
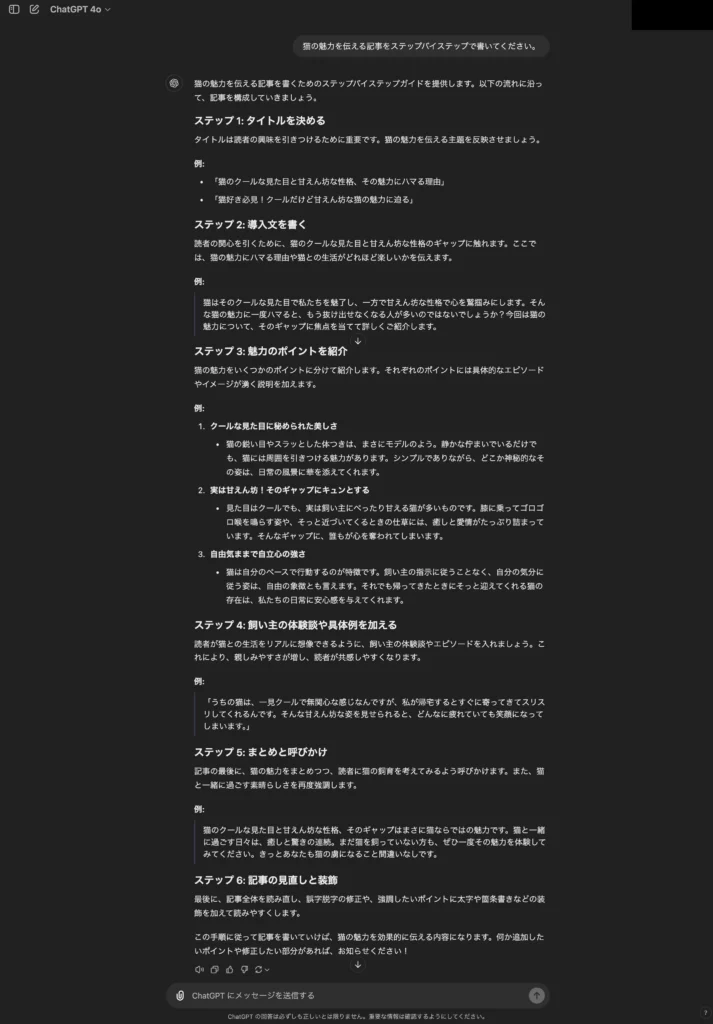
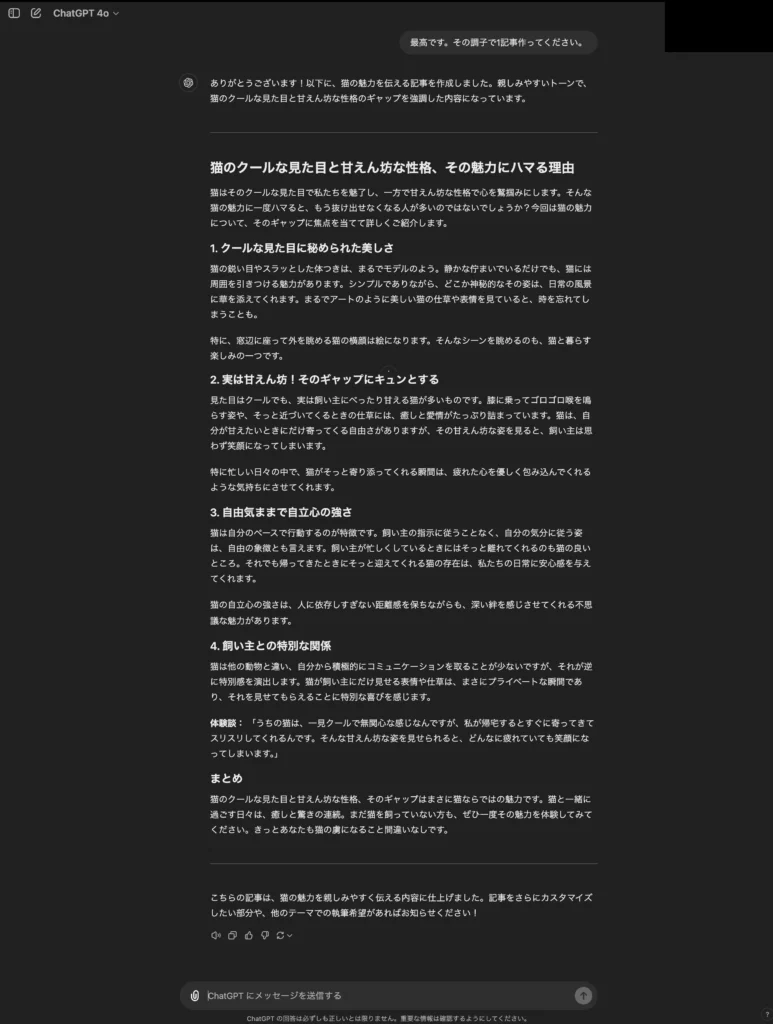
以上のとおり。理路整然とした回答が得られます。
なお、より詳細なプロンプトのテクニック(プロンプトエンジニアリング)について知りたい方は、下記の記事を合わせてご確認ください。
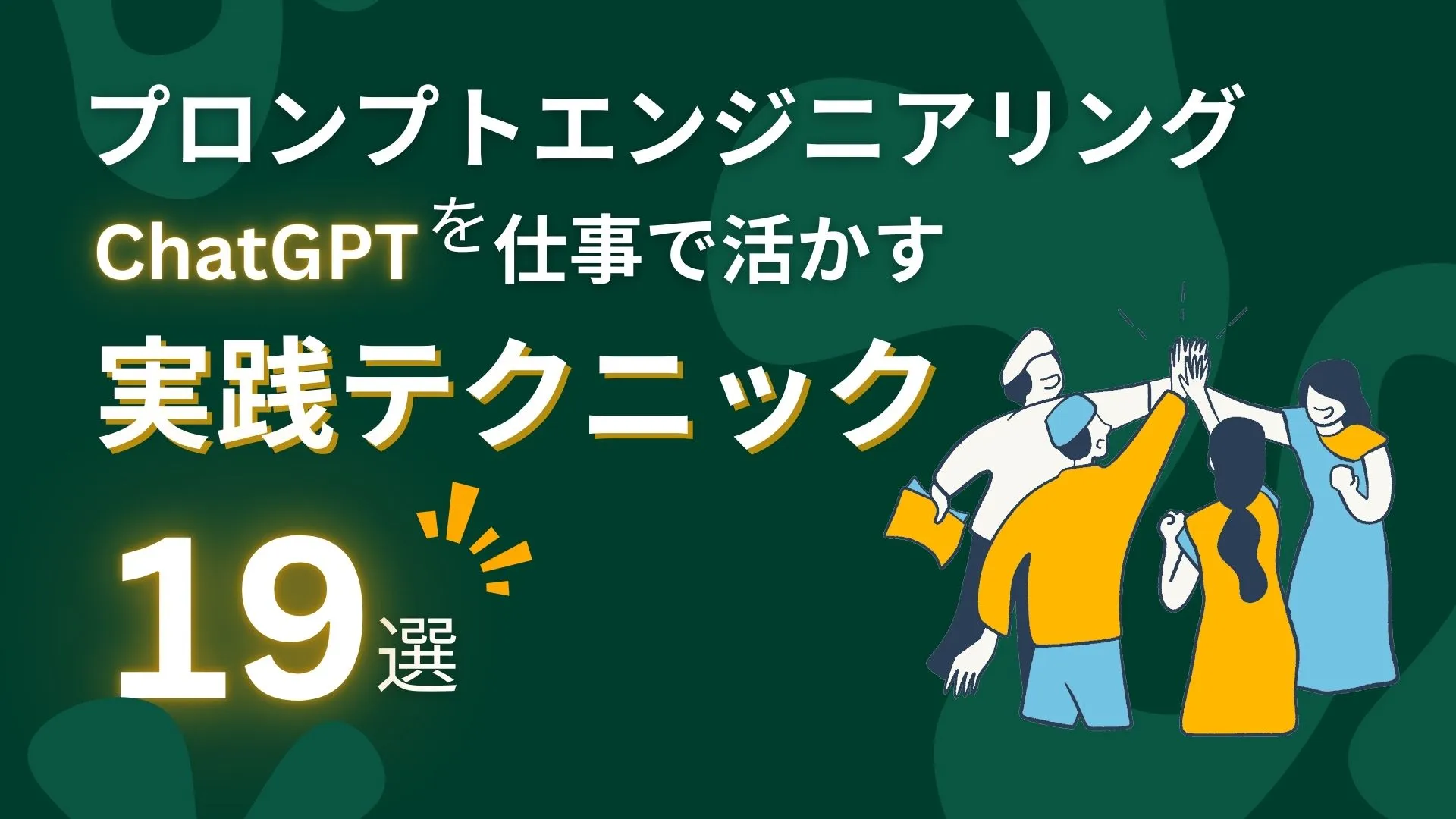
プロンプトのテンプレート
ここでは、すぐに応用できるプロントのテンプレートをご紹介します。
プロンプトには、大きく分けて以下の3つのパターンがあります。
- 質問型プロンプト
- 指示型プロンプト
- 話題設定型プロンプト
それぞれのプロンプトの概要とテンプレートをご紹介していきます。
質問型プロンプト
最も基本的なパターンが、「質問型プロンプト」です。
ChatGPTに質問を投げかけて、その質問に対する回答を生成するというものです。
「○○は何ですか?」というような一般的な質問形式から、「○○について教えてください」などのお願い形式も質問型に当たります。
ビジネスシーンでも、専門用語の解説や難解な用語・概念を分かりやすく説明してもらうなど、さまざまな使い方ができます。
▽テンプレート1:質問する
{キーワード}は何ですか? 〈例〉日本で1番高い山は何ですか?
▽テンプレート2:教えてもらう
{キーワード}について教えてください。 〈例〉リーマンショックについて教えてください。
▽テンプレート3:専門用語の解説
{専門用語}について、{ターゲット}に向けて明瞭で分かりやすい言葉を使って段階的に解説してください。
専門的な言葉や複雑な考え方が出てきたら、それを理解しやすくするための例や比喩を用いて詳しく説明してください。
▽テンプレート4:効果的な語学学習方法を尋ねる
あなたは、{言語}の先生(またはプロのコーチや専門家)です。
現在の私の{言語}レベルは{レベル(初心者、中級、CEFRなど)}です。
{希望の期間(1ヶ月、3ヶ月など)}後に{目標レベル}までの{スキル内容(単語力、会話力など)}を身に着けるための効果的な学習方法を教えてください。
{期間(1週間、1ヶ月など)}ごとの具体的な学習内容を提示してください。
※{}内は目的に合った単語・内容に変更して使用してください。
指示型プロンプト
ChatGPTに指示をして回答を生成するパターンが、「指示型プロンプト」です。
「要約して」「生成して」「コードを書いて」など、命令形式が指示型に当たります。
「プロンプトを上手に書くコツ」の項でもお伝えしたように、指示内容は具体的かつ明確であればあるほど回答精度は高くなります。
▽テンプレート1:論文の要約(論文はPDFをアップロード)
この論文の内容を、{対象者(小学生、高校生、知識のない人など)}でも理解できるレベルに簡略化して要約してください。
要約する際は、#出力条件に従ってください。
#出力条件
- 要約後の文字数は問いません。
- 一文ごとに改行をして見やすくしてください。
- 専門用語は可能な限り平易な言葉に置き換えてください。
- 必要に応じて簡単な例を用いて説明してください。
▽テンプレート2:ビジネスメールの作成
#命令書: あなたは、プロの営業担当者です。
以下の制約条件と入力文をもとに、最高の{キーワード(請求書、お礼、挨拶など)}に関するビジネスメールを出力してください。
#制約条件:
・{敬語を正確に使う}
・{連絡先を含める}
・{件名に「○○送付のお知らせ」を含める}
・{添付ファイルに言及する}
#入力文: {メールに入れる必要事項を記載}
#出力文: このタスクで最高の結果を出すために、追加の情報が必要な場合は質問をしてください。
▽テンプレート3:文章の校正
以下の文章から、誤字・脱字・不自然な表現を見つけて、修正すべき箇所をすべて挙げてください。
その上で、修正した後の文章案を作成してください。
###校正してほしい文章: ””” {文章} “””
▽テンプレート4:英文の添削
以下の英文を、ネイティブスピーカーが使う自然な表現に添削してください。
また、文法やスペルの誤り、語句の不適切な選択があれば指摘し、改善案も提示してください。
添削後は、どうしてその表現にしたのかも教えてください。
なお、文章のトーンは{トーン(カジュアル、フォーマルなど)}にしてください。
#英文: {添削したい英文を入力} ※{}内は目的に合った単語・内容に変更して使用してください。
※{}内は目的に合った単語・内容に変更して使用してください。
話題設定型プロンプト
ChatGPTに特定のトピックに関する情報や意見を生成させるパターンが、「話題設定型プロンプト」です。
主にディスカッションやディベート、エッセイやブログ記事の執筆など、意見や情報が求められるシーンで使用されます。
▽テンプレート1:テーマについて掘り下げる
{テーマ}についての重要性を述べてください。
▽テンプレート2:テーマのメリット・デメリットを述べる
{テーマ}について、メリットとデメリットをそれぞれ{文字数}以内で説明してください。
▽テンプレート3:テーマに関するアイデア出し
#命令文 以下のテーマで独創的な案を以下の処理に従って提示してください。
#処理 テーマについて5つ考え、level1の回答として提出してください。
次に、1~5のそれぞれの回答をさらに独創的にするための案を提出してください。
続いて、先ほどの案を踏まえた改善版の活用方法を5つ考え、level2の回答として提出してください。
これをlevel5まで繰り返してください。
#テーマ {キーワードを入力}
▽テンプレート4:想定される質問を挙げる
あなたは{職種}で働くオペレーターです。
{キーワード}のカスタマー対応を担当していますが、顧客からの質問にはどのようなものが考えられますか。
※{}内は目的に合った単語・内容に変更して使用してください。
ChatGPT全般でのプロンプトの書き方のコツ
続いては、OpenAIが公開しているChatGPTでのプロンプトの書き方のコツを5つご紹介します。(※1)
- 質問を具体的かつ明確にする
- プロンプトを小分けにする
- 条件を指定する
- 参考情報を提示する
- ChatGPTにプロンプトを考えてもらう
以下、詳しくみていきましょう!
質問を具体的かつ明確にする
ChatGPTのプロンプト入力では、内容を具体的かつ明確に伝えましょう。
質問内容が曖昧だと、ChatGPTが質問内容を理解しにくくなり回答精度が低くなります。特に、「良い感じに」「いくつか」「ちゃんとした」など、はっきりとしない表現は避けてください。
最も有名な音楽家を教えてください。
→バロック時代の最も有名な音楽家を3名挙げてください。
ほかにも、手順を明確にしたり、与える情報に制限を与えるのも有効です。
文章を書いてください。
→次に私が指定する題材について文章を書いてください。ただし、記事の形式は結論・その理由・具体例の順とすること。
最新のAI技術トレンドを教えてください。
→2024年のAI技術に関する最新トレンドについて、主要なポイントを3つに絞って説明してください。
以上のとおりです。プロンプトは言い換えると「自然言語のプログラム」ですので、人間が読んでくどいぐらいのものがベストになります。
プロンプトを小分けにする
ChatGPTに長文のプロンプトを入力した場合、命令がうまく反映されないこともあります。
そんなときはプロンプトをタスクごとに分けて入力して、最後に各プロンプトでの生成物をまとめる方法が有効。こちらはとくに、ChatGPTに長い文章を生成・要約させる際に必須のテクニックです。
また、前後の会話内容を利用するのもおすすめです。
ChatGPTは、同じチャットルーム内の過去の会話内容をある程度記憶しておけます。そのため、前の会話内容を要約させたり、次の会話にタスクを引き継いだりといった使い方も可能です。
条件を指定する
深津式プロンプトの項目でお伝えしたように、ChatGPTは指定した条件に沿って回答を生成してくれます。したがって、以下の条件を指定しておくと、狙った回答が得やすくなります。
- 対象者:回答結果が「誰に向けたものなのか」を提示する
- ChatGPTの役割:料理の専門家や英語の先生など、得たい回答に沿った専門家を指定する
- 回答の形式:出力文の文字数や表・箇条書き形式の指定など
生成AIについて解説してください。
→生成AIについて、生成AIを全く知らない小学生向けに分かりやすく解説してください。
分数について教えてください。
→あなたは小学校の算数の先生です。分数について分かりやすく説明してください。
ChatGPTの業務活用アイデアを出してください。
→業務におけるChatGPTの活用アイデアと根拠となる理由を、それぞれ200文字以内で箇条書きで5つ作成してください。
参考情報を提示する
通常、ChatGPTは学習範囲外の情報(最新のニュースやローカルな知識)について回答を提供できません。また、学習済みの情報についても、しばしばハルシネーション(誤情報)を含めてしまいます。
しかし、ChatGPTは、与えられた参考情報(テキスト)を基にした回答を生成できます。
OpenAI社が提供する「GPT best practices」にも記載されていますが、参考情報を与えることでChatGPTは嘘の少ない回答を生成しやすくなるとのことです。
たとえば商品PR文であれば、参考にできるソースとして商品の詳細情報や仕様、類似した過去の商品PR文などをプロンプトに含めると、より効果的で希望に沿った回答を得られます。
また、特定の報告書を基にした要約や学術論文を引用しての説明なども挙げられます。
以下の参考文書に基づいて、商品PR文を100文字以内で作成してください。
“””
{参考にする文書}
この方法なら、学習範囲外の情報も含めて、ある程度正しい回答が得られるようになります。
ChatGPTにプロンプトを考えてもらう
今回ご紹介する方法は、ChatGPTに「プロンプトをより洗練してください」とお願いするというものです。
つまり「プロンプトを改善するプロンプト」を作ります。
今回のハンズオンでは、
テキスト生成AIの活用方法に関する記事
というプロンプトを改善していきます。
この抽象的で、ChatGPTに何をしてもらいたいのか分からないものが、なんと……
製造業におけるコミュニケーションの問題を解決するために、テキスト生成AIを活用する方法について説明してください。
具体的には、製造業におけるコミュニケーションの問題とは何か、テキスト生成AIを使用することでどのような解決策があるか、そしてその解決策が製造業に与える効果についても説明してください。
ただし、生成されるテキストには、誤った情報や暴力的な内容が含まれないように注意してください。
という具体的なものに変化していきます。
それでは早速プロンプト改善をやっていきましょう!
1.OpenAI Playground を開きます。
Playground を開くとこのような画面になります。
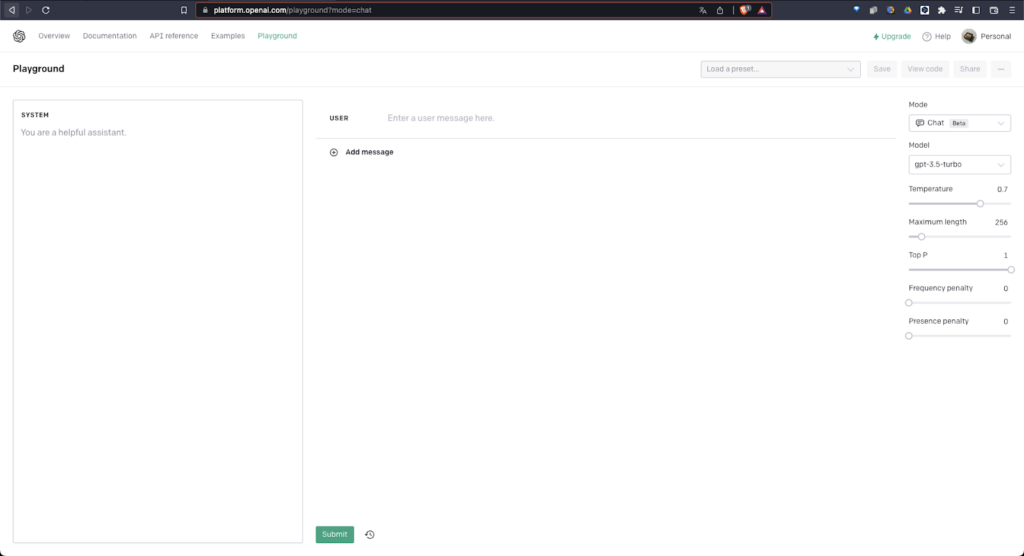
2. 画面の左側に、プロンプトを入力すると……
私のプロンプトエンジニアになってほしい。
あなたの目標は、私のニーズに最適なプロンプトを作成できるようにすることです。
このプロンプトは、ChatGPT によって使用されます。
次のプロセスに従います。
<br>あなたの最初の応答は、プロンプトが何についてであるべきかを私に尋ねることだけです。
ユーザーの回答を得てから、継続的な反復を通じて改善する必要があります。
<br>ユーザーの入力に基づいて、3つのセクションを生成します。
a) 改訂されたプロンプト (書き直されたプロンプトを提供します。
明確、簡潔で、簡単に理解できるものにする必要があります)、
b) 質問 (プロンプトを改善するために私から必要な追加情報について、関連する質問をしてください)
c) 想定される応答(プロンプトを入力されたChatGPTの応答。一文で。)
<br>この反復プロセスは、私があなたに追加情報を提供し、あなたが改訂されたプロンプト セクションのプロンプトを更新して、私が完了したと言うまで続けます。
3. 画面右側にこのように出力されます。(以下の画像参照)
はい、喜んでプロンプトエンジニアとしてお手伝いします!
まずは、あなたが望むプロンプトのトピックについて教えていただけますか?
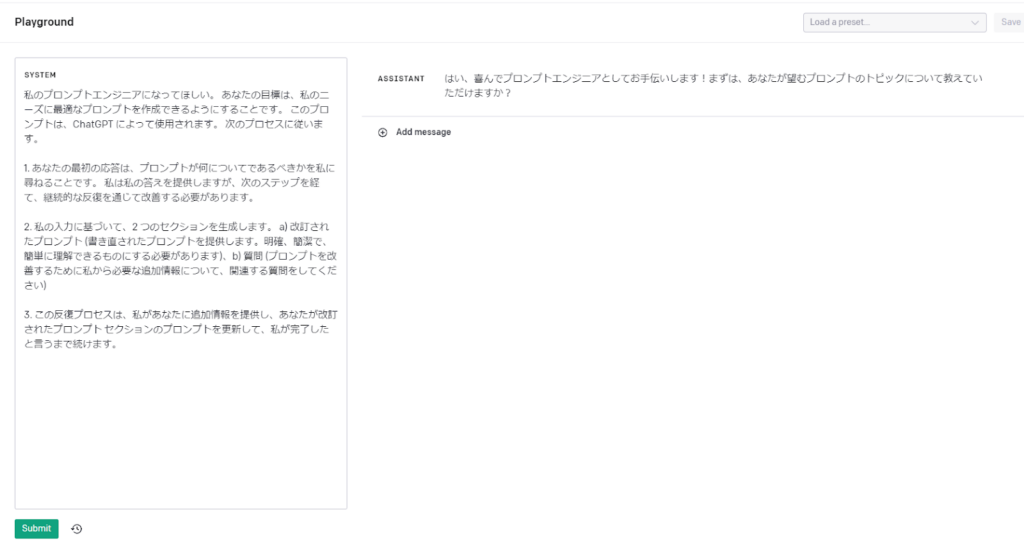
これで、ひとまず準備は完了です。
4. どんなプロンプトを書きたいか伝えます。
例えば、
テキスト生成AIの活用方法に関する記事と入力すると、ChatGPTから次のようなテキストが……
改訂されたプロンプト、
必要な追加情報についての質問
が表示されてます。
改訂されたプロンプトをよく見ると、
具体的で、細かい内容にまで触れているプロンプトになりました。
ありがとうございます!理解しました。
改訂されたプロンプトと、必要な追加情報についての質問を提供いたします。
改訂されたプロンプト:
テキスト生成AIの活用方法について、詳しく説明してください。
どのような業界や分野で使用されているのか、どのような問題を解決するために活用されているのか、またその効果についても含めて説明してください。
さらに、その利用方法に関する成功事例や、今後期待される可能性についても述べてください。
必要な追加情報についての質問:
1. テキスト生成AIを使用する際に、どのような種類のデータや素材が必要ですか?
2. テキスト生成AIを用いたプロジェクトにおいて、どのような種類の問題を解決することができますか?
3. テキスト生成AIの利用方法について、技術的な詳細を含めた解説が必要ですか?それとも、一般的な理解を深めるための説明で十分でしょうか?
4. テキスト生成AIを使用する際には、どのような倫理的な問題が考慮される必要がありますか?
5. テキスト生成AIを使用する上での成功事例について、特に興味のある分野や業界はありますか?
5. ChatGPTから、もっと具体的なプロンプトを書くために、
必要な追加情報の質問が5つ提示されていたので、以下のように回答します。
テキスト生成AIはChatGPTを使用します。 業務におけるコミュニケーションの問題を解決したいです。 詳細な解説は必要ありません。 誤った情報や暴力的な内容は出力されるべきではありません。 製造業に興味があります。それに対する返答が以下です。
改定されたプロンプトに、質問への回答が考慮されたものが表示されています。
ありがとうございます!理解しました。
改訂されたプロンプト:
製造業におけるコミュニケーションの問題を解決するために、テキスト生成AIを活用する方法について説明してください。
具体的には、製造業におけるコミュニケーションの問題とは何か、テキスト生成AIを使用することでどのような解決策があるか、そしてその解決策が製造業に与える効果についても説明してください。
ただし、生成されるテキストには、誤った情報や暴力的な内容が含まれないように注意してください。
質問:
1. 製造業におけるコミュニケーションの問題とは、どのようなものがありますか?
2. テキスト生成AIを使用して、製造業におけるコミュニケーションの問題を解決するには、どのような種類のデータや素材が必要ですか?
3. テキスト生成AIを使用する際に、誤った情報や暴力的な内容が出力されないようにするために、どのようなデータ管理や品質管理の手段が必要ですか?
4. テキスト生成AIを使用することで、製造業にどのような具体的な効果が期待できますか?例えば、コミュニケーションの改善による生産性の向上や、コスト削減などがある場合、その具体的な数字や事例を教えてください。
5. 製造業以外でも、テキスト生成AIを使用してコミュニケーションの問題を解決することができる可能性がありますが、製造業に特化した利用方法に関する情報が必要ですか?それとも、他の分野や業界での成功事例についても興味がありますか?
ひとまず質問への回答はやめますが、いかがだったでしょうか。
質問への回答を繰り返すだけで、
テキスト生成AIの活用方法に関する記事
というざっくりとしたプロンプトを、
製造業におけるコミュニケーションの問題を解決するために、テキスト生成AIを活用する方法について説明してください。
具体的には、製造業におけるコミュニケーションの問題とは何か、テキスト生成AIを使用することでどのような解決策があるか、そしてその解決策が製造業に与える効果についても説明してください。
ただし、生成されるテキストには、誤った情報や暴力的な内容が含まれないように注意してください。
という、具体性の高い内容に磨き上げることができました。
この方法は、文章力に自信がない方に重宝されるかなと思います。
Stable DiffusionなどのText to Image向けのプロンプトを作成することもできそうですので、ぜひご活用いただければと思います!
なお、ChatGPTにプロンプトを考えてもらうのが面倒な方は、APIベースで使えるChatGPTのプロンプトジェネレーターの利用もおすすめです。
APIを利用するための料金がかかりますが、Open AI公式が開発したツールのため、安心感があります。
プロンプトジェネレーターは、ChatGPTの有料プランを契約していれば、Playgroundにアクセスすることで利用できます。
なお、ChatGPTのプロンプトインジェクションについて詳しく知りたい方は、下記の記事を合わせてご確認ください。
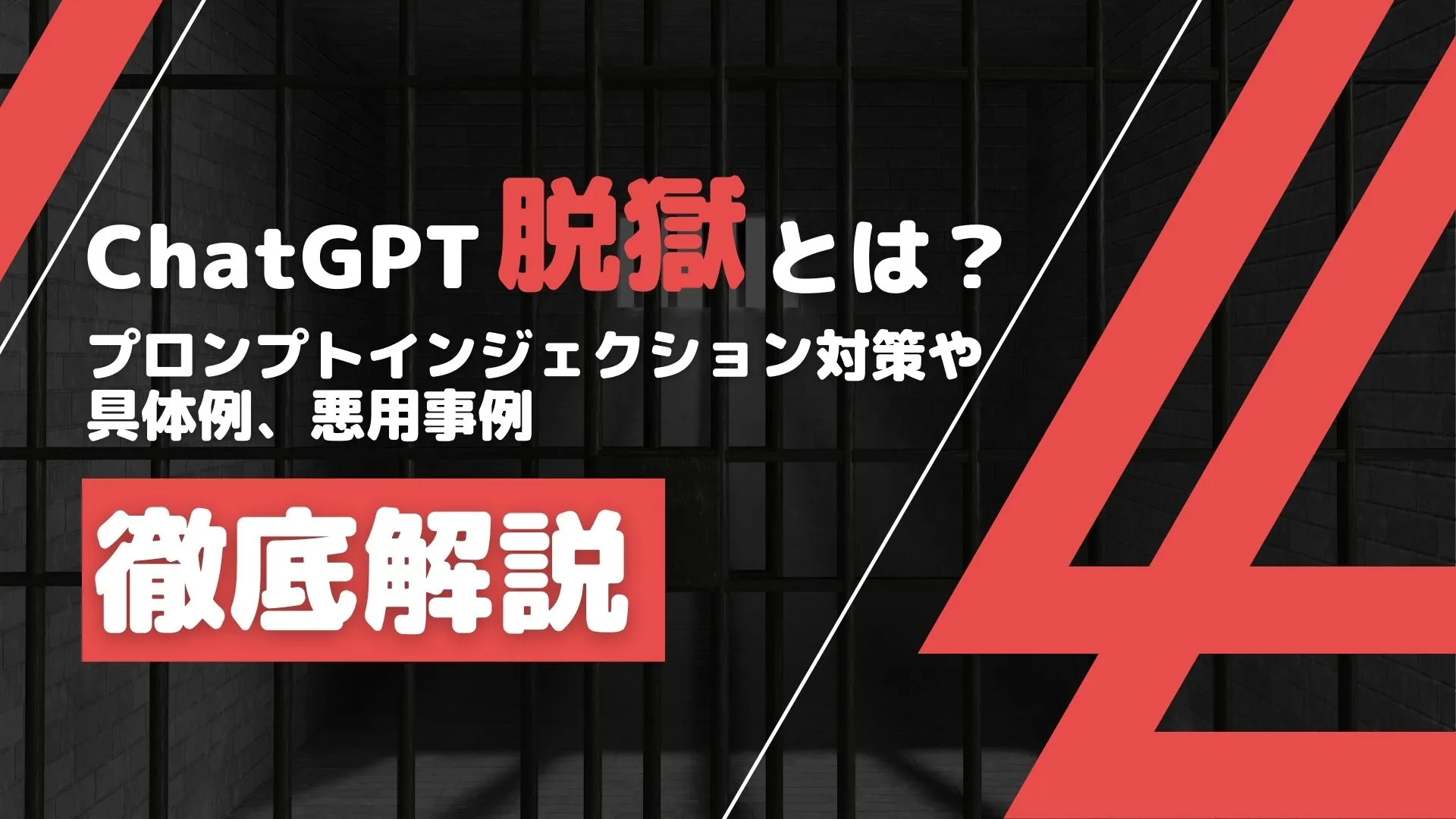
OpenAI o1限定のプロンプトのコツ
2024年9月12日に登場したChatGPTの最新モデル「OpenAI o1」は、それ以前のGPTシリーズとは明確に異なる立ち位置にあります。
これまでになかった「推論の生成」を経由して回答をまとめることで、柔軟かつ緻密な思考過程が実現。従来比で問題解決能力がUPしています。
そんなOpenAI o1では、いままでのGPTシリーズで有効だったプロンプトが一部通用しなくなっています。ここからは、そんなOpenAI o1限定のプロンプトの書き方について、詳しくみていきましょう!
プロンプトは短文が最適
多角的かつ緻密に推論が行えるOpenAI o1に対しては、短文の簡潔なプロンプトを入力するのがベスト。GPTシリーズのプロンプトのように長々と手順を示すと、かえって思考の幅が狭まってしまいます。OpenAI o1の自主性に任せましょう。
Chain-of-Thought(CoT)は不要
OpenAI o1はChain-of-Thought(CoT) と同じ流れで推論を行います。したがって、プロンプトに「ステップバイステップで」の文言を含める必要は一切ありません。こちらもOpenAI o1に委ねてしまいましょう。
参考情報の提示は最小限でOK
OpenAI o1に参考情報を示す場合は、関連性の高い情報だけを厳選してプロンプトに含めるのが得策です。こちらも参考情報が多いと、推論に支障が出てしまいます。事実ベースの回答を得たい場合は引き続き、GPT-4oを活用しましょう。
ChatGPTのプロンプト文例集10選!
ここからは、ビジネスシーンで使えるChatGPTのプロンプト一覧をご紹介します。
- 【事務】データの整理&CSVへの変換
- 【営業】営業メールの作成
- 【人事】求人票の作成
- 【企画・開発】擬似的な商品レビューの取得
- 【カスタマーサポート】問い合わせ対応
- 【ディレクター】文章の校正
- 【ライター】ブログ記事の作成
- 【プログラマー】Pythonコードの解説&バグ修正
- 【マーケター】感情の検出・分析
- 【リライター】文字起こしの要約
職種別に効果的なプロンプトをまとめたので、ぜひ活用してみてください。
【事務】データの整理&CSVへの変換
ChatGPTは、文章やソースコード等の無秩序なデータからでも情報を抽出・整理できます。こちらを応用することで、Web記事やPDFから必要な情報のみを抜きとってスプレッドシートにまとめる、といったことも可能です。
試しに以下のプロンプトをChatGPTに入力して、乱雑な自己紹介の文章を整理されたCSVファイルに変換してみましょう!気になる結果は……
#命令
あなたは最強最高の事務スタッフです。以下の自己紹介から、登場人物名と好物と趣味を抽出して、カンマ区切りのCSV形式にまとめてください。
#自己紹介
ぼくは太郎。オムライスが大好物なんだ。趣味は……サッカーだね!
私は華道をたしなむ美代子よ。好きな食べ物は、肉じゃがとすきやきね。
わたくしは花子ですわ。大好物はフォアグラで、趣味は乗馬ですわ〜!
#出力
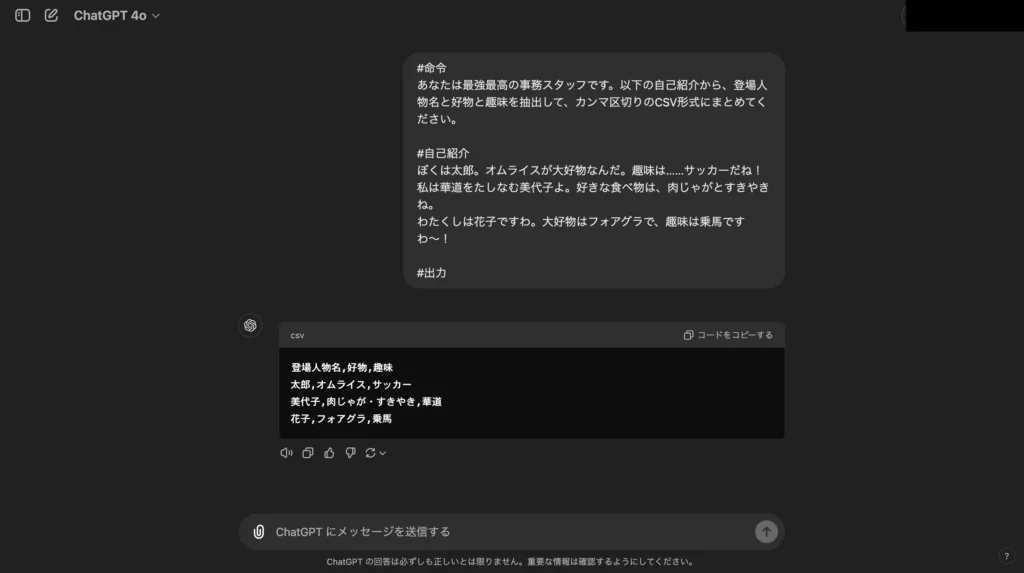
このように、カンマ区切りのテキストが返ってきました。こちらをテキストエディタに入力して、拡張子を「.csv」に変えると……
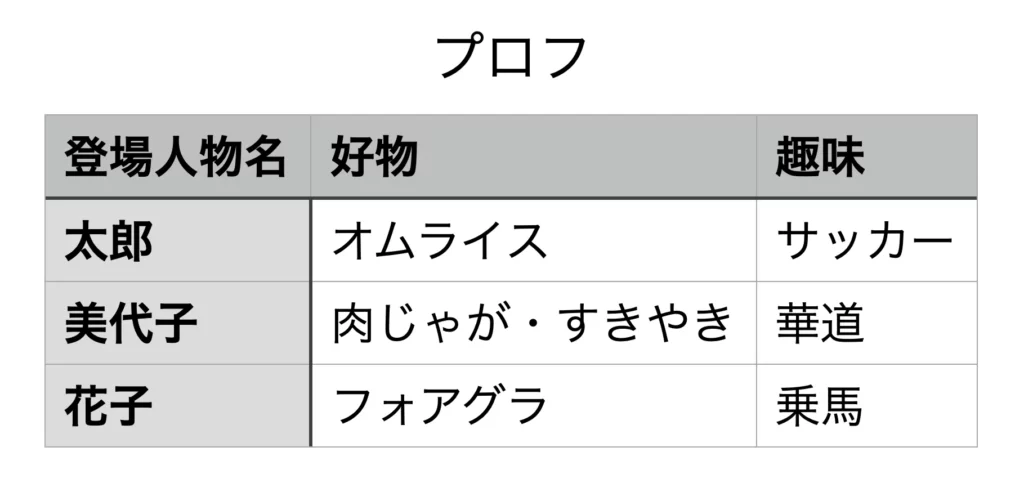
お見事!スプレッドシートの完成です。
【営業】営業メールの作成
ChatGPTを使えば、自身の職種や顧客の属性に合わせた営業メールを一瞬で作成できます。一人ひとりに寄り添った文章を考えるのは大変ですが、ChatGPTを使えばメール文作成の手間を大幅に軽減できるはずです。
営業メールのプロンプトは以下を参考にしてみてください。
あなたは不動産会社の営業マンです。
賃貸物件の内見をしてから3日経った20代男性に向けて、申し込みの意思確認と、申し込まない場合は再度別の物件の内見を促す旨を伝えるメール文を400文字までで作成してください。
#再度提案する物件の条件
・最寄駅徒歩10分県内
・家賃5万円以下の1LDK
・閑静な住宅街
・独立洗面台
・バス・トイレ別
・敷金・礼金が0円
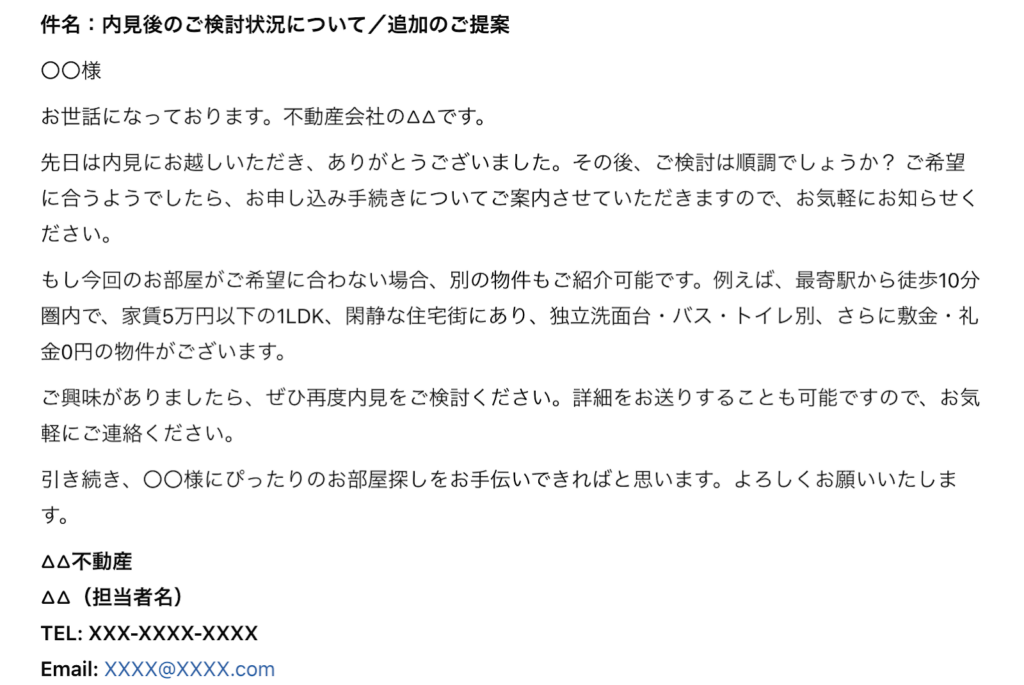
プロンプトを変更すれば、ほとんどの職種・客層に対応可能です。
ぜひ活用してみてください!
【人事】求人票の作成
会社の特徴や募集要項をプロンプトに含めれば、求人票の作成もChatGPTに任せられます。自分でうまく言葉をまとめるのが苦手な人事担当の方は、以下のプロンプトを試してみてください。
あなたは職務歴20年の物流会社の人事担当者です。会社情報、募集内容に沿って求人票の文章を作成し、出力形式に従って出力してください。
#会社情報
物流会社
従業員数300名
会社のビジョン「商品を心で届ける」
年1回の昇進のチャンス
ボーナスは年2回(7月、12月)
年間売上高は10%以上伸びている
#募集内容
ドライバー
正社員
月給40万円
休日は年110日
#出力形式
募集背景
募集要項
募集内容
待遇
選考方法
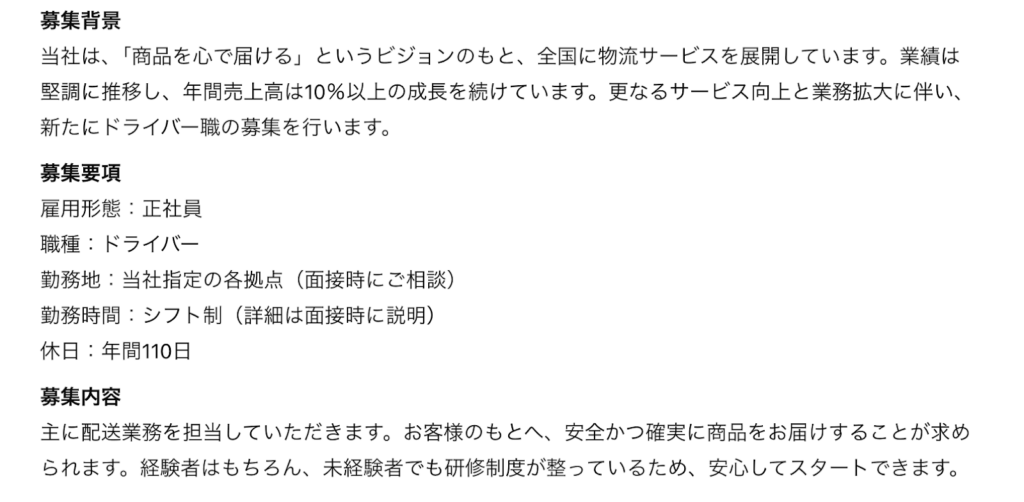
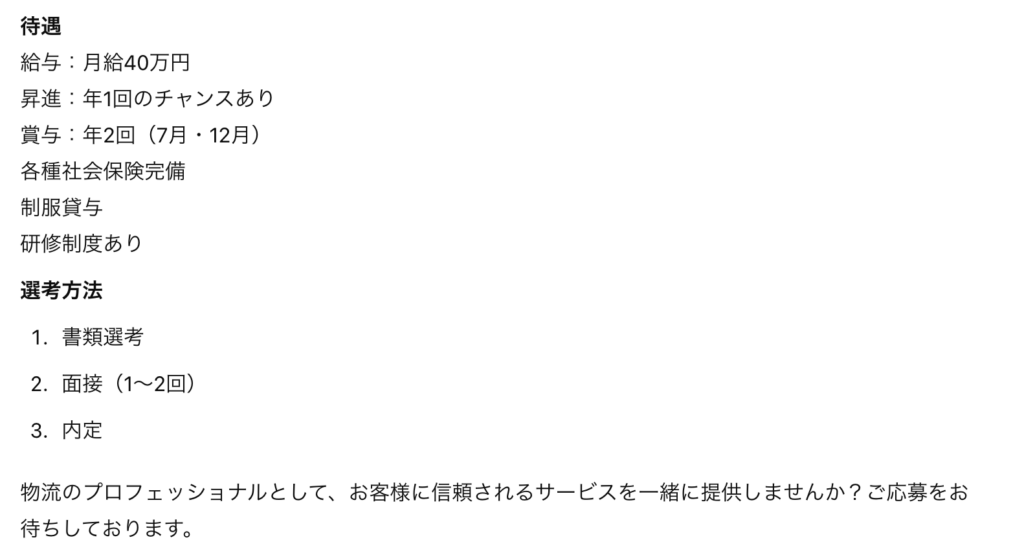
【企画・開発】擬似的な商品レビューの取得
上級者向けにはなりますが、ChatGPTでは現実世界で得られるデータに似せた合成データも作成可。擬似的なアンケート結果を得たり、AIモデルの学習データを増幅したりといった使い方ができます。
ということで、この合成データについてもChatGPTで作っていきましょう!今回は2024年9月12日に登場したChatGPTの新顔「OpenAI o1」に下記のプロンプトを入力し、擬似的なアンケートを実施してみます。
あなたは最強最高のお客様です。以下の商品について条件に則ってレビューを返してください。
なお、レビューを返すたびにあなたは記憶を失って新しいお客様になります。
私に見せる出力は、レビュー100回分をまとめてCSV形式で出力しましょう。
#条件
・顧客No.,実用性,コストパフォーマンス,購買への意欲,選ぶ理由をヘッダーとする
・顧客は1から順番に番号を振る
・実用性は1が最低で5が最高評価
・コストパフォーマンスは1が最低で5が最高評価
・購買への意欲がある場合はTRUE、ない場合はFALSE
・TRUEの場合、商品を選ぶ理由も添える
#商品
ローカルLLMが全自動でメールを生成してくれるAIツール。
あえて送信はせず、完成品をユーザーの業務チャットに転送することでチェックできる。
RAGで自社データを引用させることも可能。
150,000円
気になる結果は……
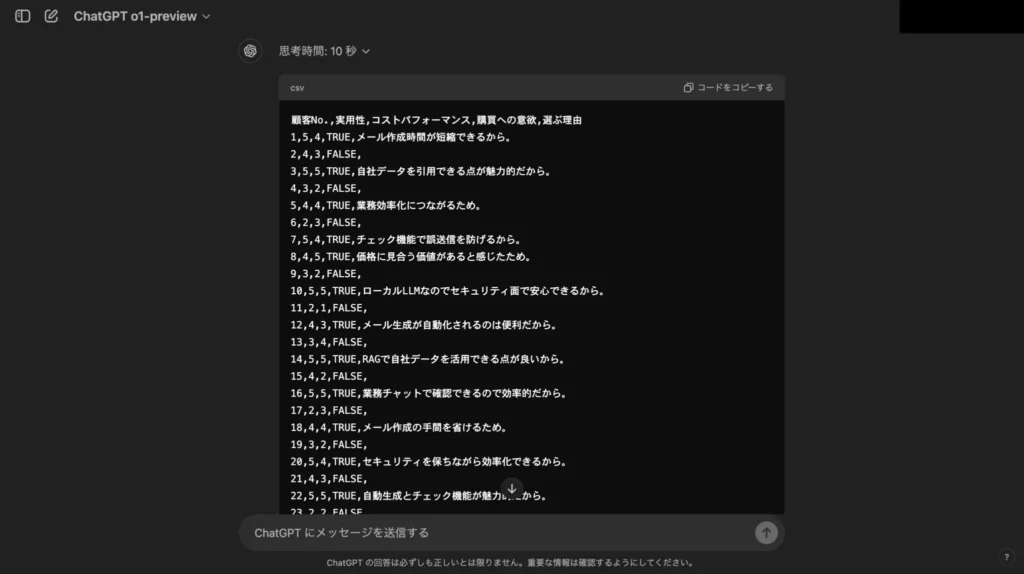
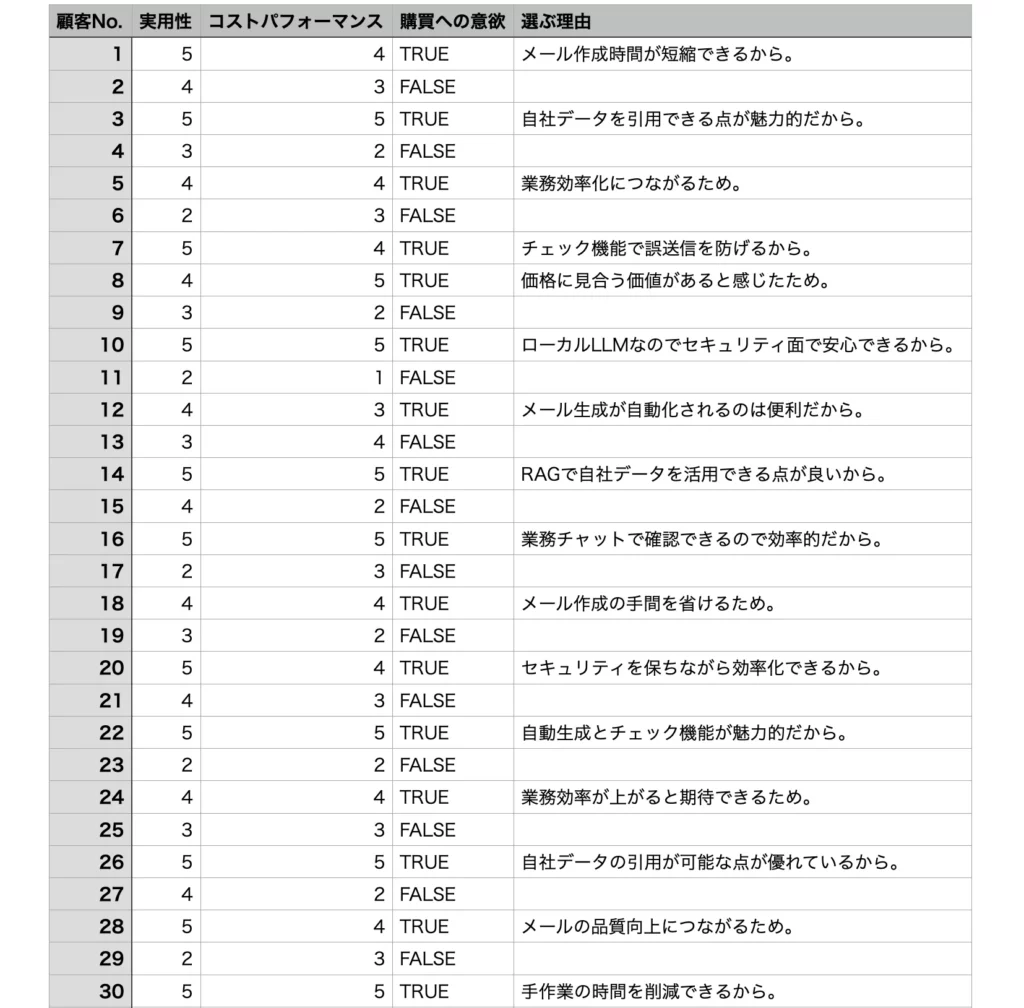
お見事です!現実的なアンケート結果が返ってきました。
【カスタマーサポート】問い合わせ対応
ChatGPTなら、顧客のクレーム対応も可能です。クレーム内容と案内したい内容を入力すれば、すぐに適切なメール文を考えてくれます。
以下に問い合わせ対応のプロンプト例を記載するので、参考にしてみてください。
#命令
あなたはデジタル機器を専門に取り扱うインターネット通販サイトのカスタマー担当です。
キーボードを購入した顧客から動作不良のクレームが入ったので、以下の対応内容を反映したうえでメールの返信文を考えてください。
#対応内容
電池が正しく差し込まれているか確認を促す
USBの再接続を促す
キーボードのドライバーをインストール&更新するよう促す
どうしても改善しない場合は保証の対象期間内なので交換対応可能なことを案内する
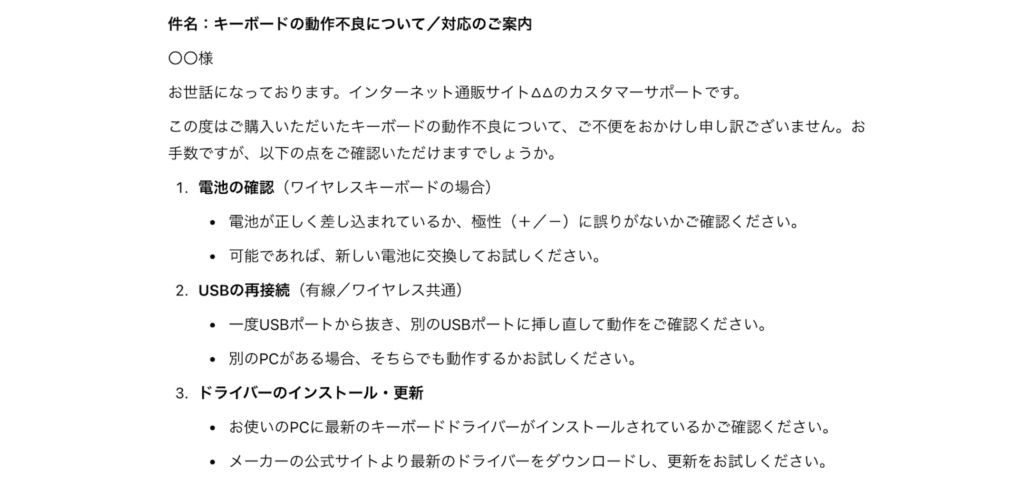

【ディレクター】文章の校正
自然言語を文法(係り受け・活用)レベルで理解しているChatGPTであれば、文章の校正・添削が可能。文章のプロにみてもらわなくても、社内文書・メール・Web記事…etc.のチェックが行えます。
ということで早速、ChatGPT(GPT-4o)に以下のプロンプトを入力して校正してもらいましょう!
#命令
あなたは最高最高のディレクターです。以下の文章を日本語文法の観点で校正してください。
#文章
わたし、視力いいんだ。遠くのあの山を見れるんだけど。
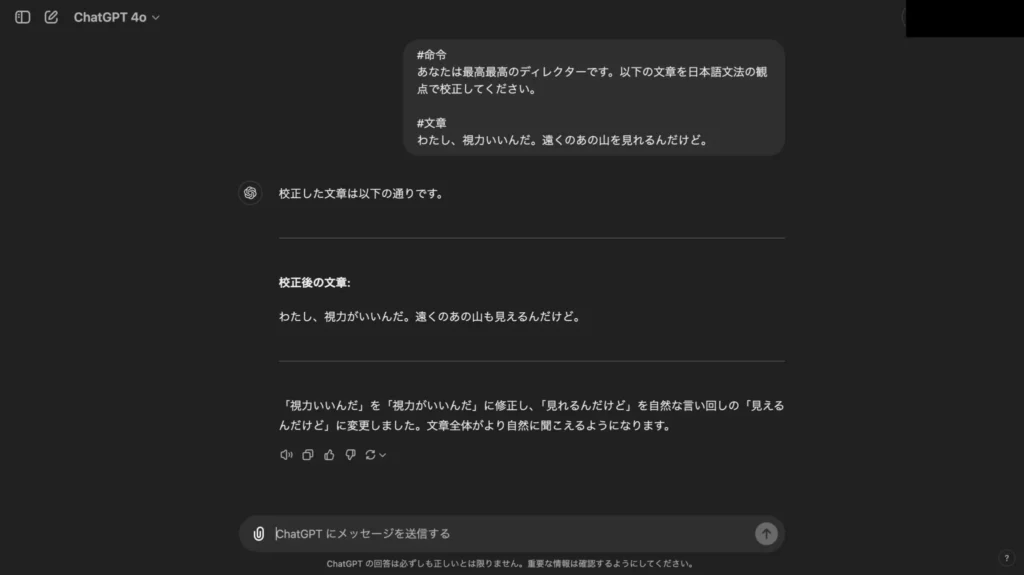
【ライター】ブログ記事の作成
ChatGPTをうまく活用すれば、SEOを意識した高品質な記事作成が可能です。プロンプトを工夫するだけで、ペルソナ設定から見出し文章の作成までを高いレベルでおこなえます。
#命令
あなたは業界20年のベテランライターです。次のキーワードで検索している人の検索意図とペルソナを設定し、それらを満たす最適なタイトルと見出し構成案を考えてください。
#キーワード
「ChatGPT 無料」
#出力形式
h1:タイトル
h2:大見出し
h3:小見出し
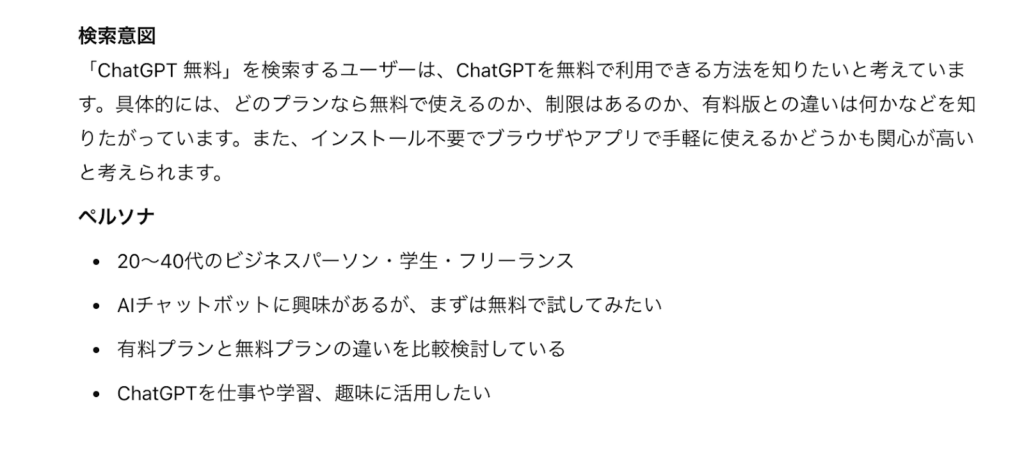
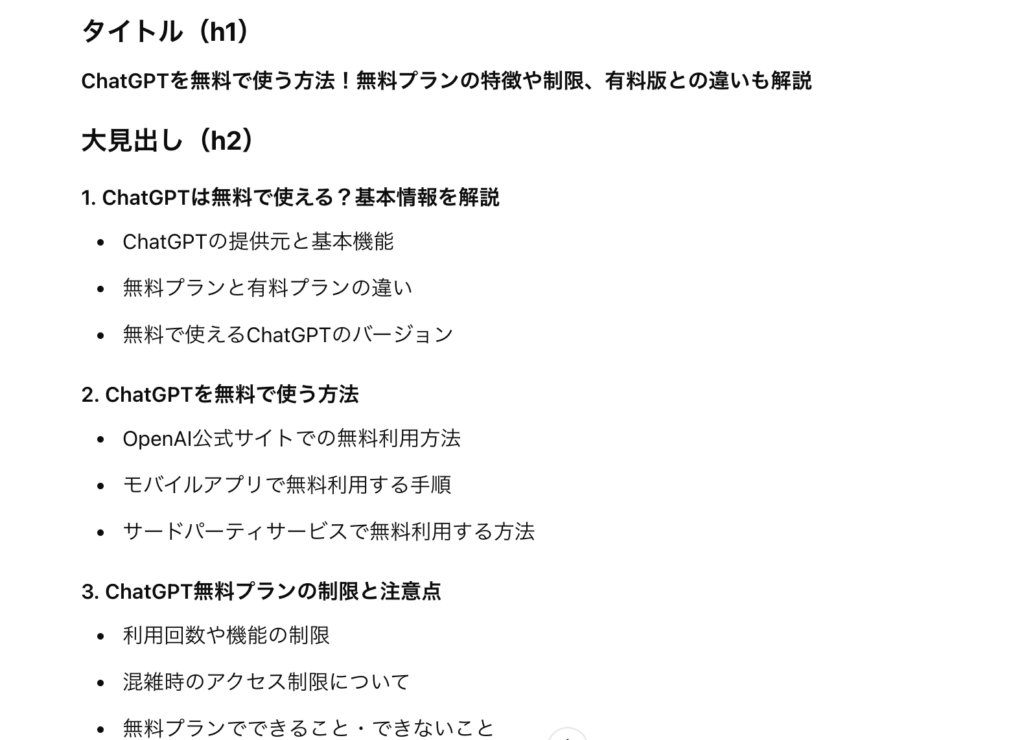
上記の構成案に沿って、それぞれの見出し文章を考えてください。
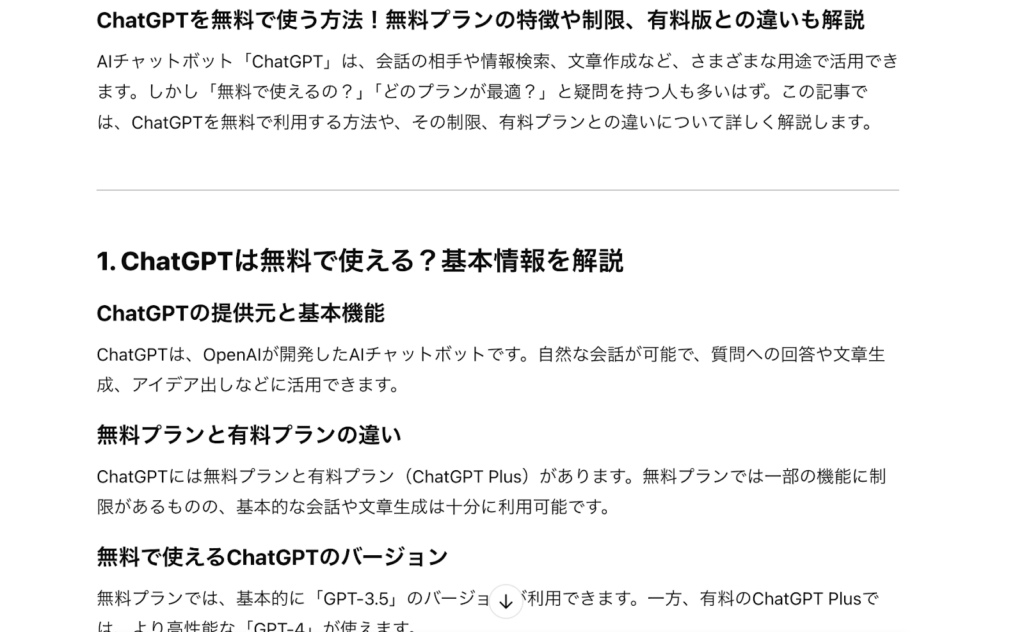
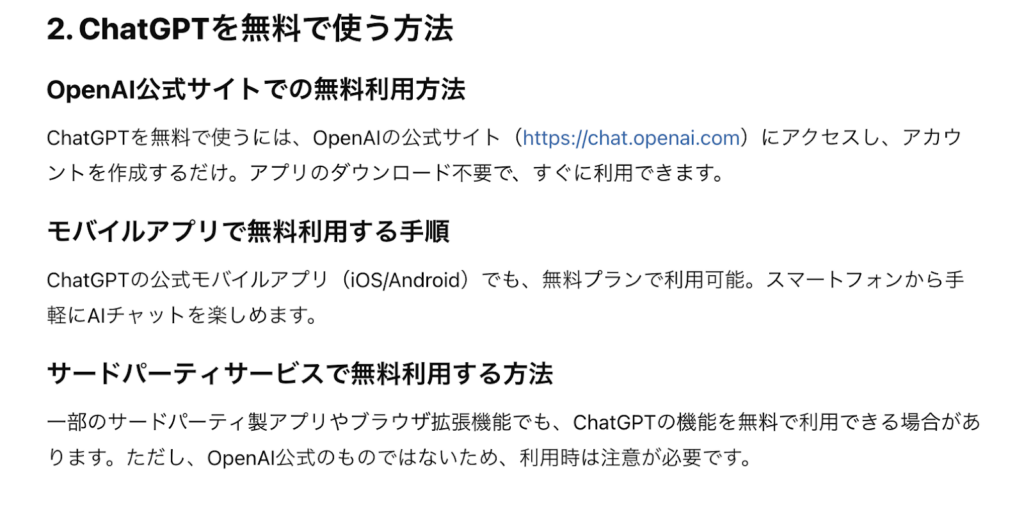
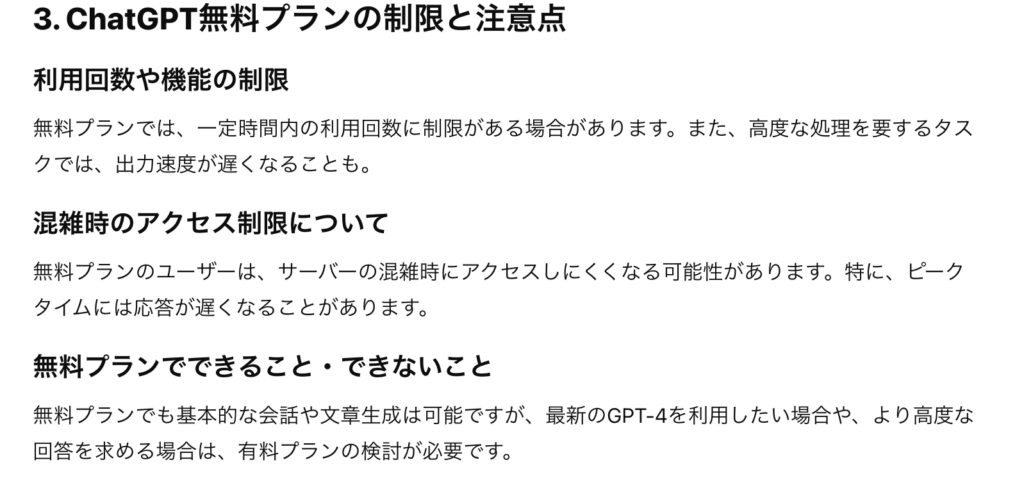
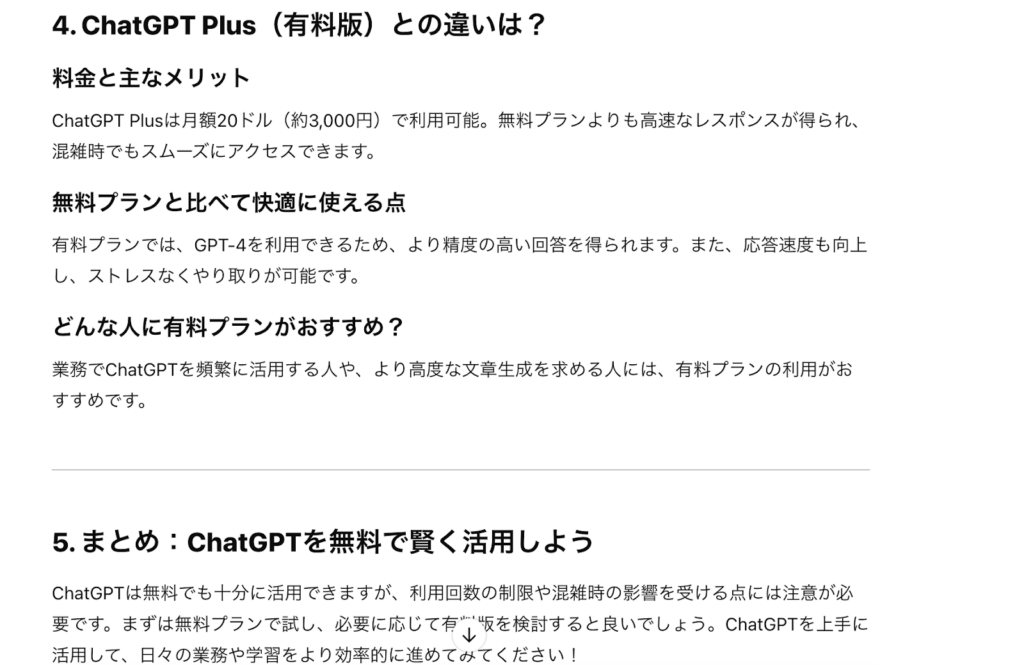
上記のように、やり取りを重ねることで検索意図の把握から見出し構成案の作成までをChatGPTで効率的に実施できます。
見出し文章は簡単な内容になっているので、自分でも詳しい情報を調べて付け足していけば、魅力的なブログ記事が出来上がるはずです。
【プログラマー】Pythonコードの解説&バグ修正
ChatGPTは自然言語だけでなく、プログラミング言語も学習済み。英語を日本語に訳すのと同じように、ソースコードを自然言語に訳して解説できます。
さらに、ChatGPTは文章の添削よろしく、ソースコードのバグの特定・修正も可能。(※6)プログラミング初心者から上級者まで、作業効率UPが見込めること間違いなしです。
ということで、ChatGPTのお手並み拝見です。以下のプロンプトを使って、Pythonコードの解説・バグ修正を試してみます。
以下のソースコードについて何をするものか解説したのち、GoogleColabで動かす際のバグを特定して直してください。
#ソースコード
pip install ultralytics
from ultralytics import YOLO
model = YOLO("yolov10n.pt")
def detect_objects_in_image(model, image_path):
results = model(image_path)
return results
image_path = 'S__58638343_0.jpg'
results = detect_objects_in_image(model, image_path)
import cv2
import numpy as np
from google.colab.patches import cv2_imshow
def visualize_results(image_path, results):
img = cv2.imread(image_path)
for r in results:
boxes = r.boxes
for box in boxes:
x1, y1, x2, y2 = box.xyxy[0]
cv2.rectangle(img, (int(x1), int(y1)), (int(x2), int(y2)), (0, 255, 0), 2)
cv2.putText(img, f"{r.names[int(box.cls.item())]}", (int(x1), int(y1)-10), cv2.FONT_HERSHEY_SIMPLEX, 0.9, (0,255,0), 2)
cv2.putText(img, f"{box.conf}", (int(x1)+300, int(y1)-10), cv2.FONT_HERSHEY_SIMPLEX, 0.9, (0,255,0), 2)
cv2.imshow("cat",img)
cv2.waitKey(0)
cv2.destroyAllWindows()
visualize_results(image_path, results)
#解説
#バグ修正後
以上のプロンプトをChatGPTに入力すると……解説→バグ修正後のコードの順に回答が返ってきました。
まずは、プロンプト内のPythonコードがどういうものなのか、その解説をみていきましょう!
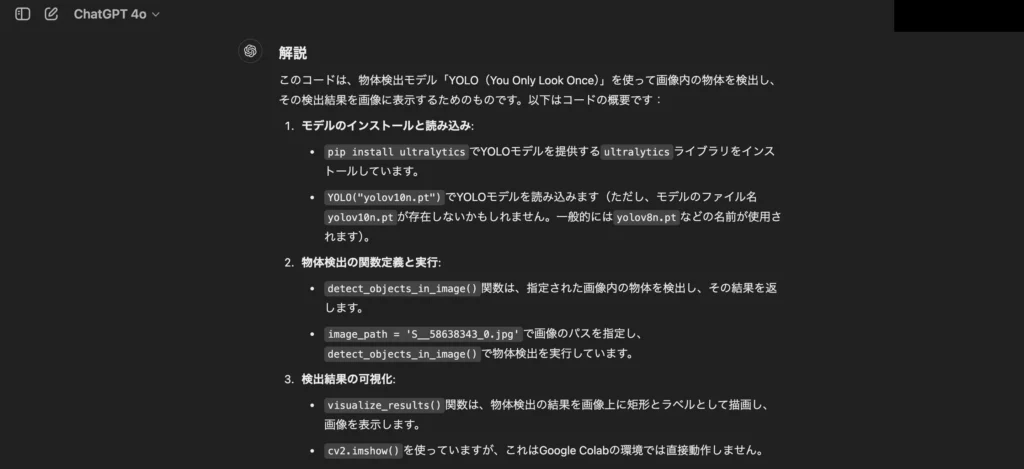
お見事です!ChatGPTは、プロンプト内のPythonコードが物体検出モデル「YOLO」の検出結果を可視化するものであることを看破しています。
続いて、バグの修正については……
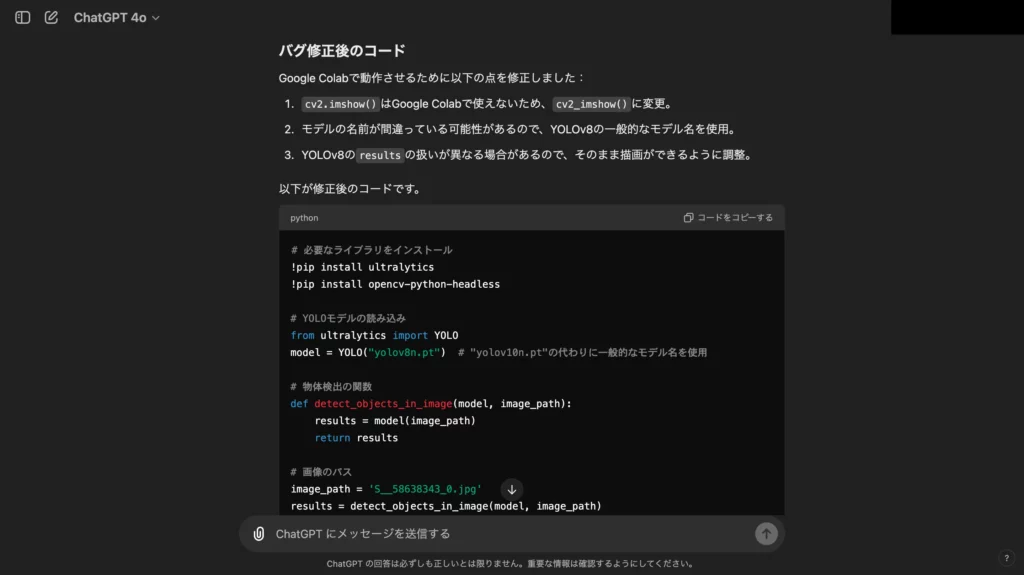
こちらも上出来!ChatGPTは下表のとおり、バグを正しく検出・修正できています。
検出したバグ | 修正後 |
---|---|
「YOLOv10」は存在しない (2024年5月登場で、 GPT-4oの学習範囲外のため) | 「YOLOv8」 に変更 |
cv2.imshow( )は GoogleColab非対応 | cv2_imshow( ) に変更 |
pip installは GoogleColab非対応 | !pip install に変更 |
これなら、初心者でも複雑なソフトが作れてしまいそうですね。
【マーケター】感情の検出・分析
ChatGPTであれば、本来は難しい感情表現の数値化が可能。口コミやアンケートの分析で大活躍してくれます。
今回はそんなChatGPTによる感情分析を、当メディアのニュース記事のタイトルで試してみます。
そのプロンプトは下記のとおり。5段階のネガポジ分析を実施していきます。
以下のニュース記事タイトルについて、ポジティブ2から中庸0、ネガティブ-2までの5段階で評価してください。
#ニュース記事タイトル
Microsoftの新AIモデル「GRIN-MoE」が業界に革新をもたらす
Uniphore、企業向けAI開発支援の新プラットフォーム発表
OpenAI、最新AIモデルで高度な問題解決を実現
ローソンが「未来のコンビニ」を発表、新技術で新時代へ
アドビ調査、大統領選を前に誤情報対策の強化を消費者が要望
AIデータクラウドで企業変革を加速
AI活用も視野 富裕層の新投資戦略
AI専門家集団が企業のAIリスクを診断
DataStax CEO:2025年、AIが企業を変革する年になる
Hume、低コスト&高機能の音声AI「EVI 2」発表
スーパーインテリジェンス競争が激化、AI時代の到来
Rep.aiがAI営業アバター技術で750万ドルの資金調達
SiFive、RISC-Vベースの新AIチップを発表
Magnit、AI搭載の採用支援ツール「Maggi」を発表
AIが切り拓く音声革命、EzAudioの挑戦
AI搭載ロボット、靴ひも結びに挑戦
AISI、AI安全性評価の新指針公開
AIも絡む トランプ氏著作権訴訟敗訴
AI恋人アプリ進化 倫理的課題も浮上
AI搭載「ClickUp Chat」登場 業務革新へ
AI搭載CRM「Pulse」登場 営業革新へ
EA、AI全面活用でゲーム開発革新へ
MSI新AI搭載ゲーミングPC 性能進化へ
AI新モデル o1 進化と課題の両面性
AI倫理の危機 GPT Store監視に不備
AI教育革命 元OpenAI技師が挑戦
AI活用で英語力急上昇 安河内氏が伝授
さて、気になる結果は……
ネガポジ分析の結果
ニュース記事タイトル | ネガポジ評価 |
---|---|
Microsoftの新AIモデル「GRIN-MoE」が業界に革新をもたらす | +2 |
Uniphore、企業向けAI開発支援の新プラットフォーム発表 | +1 |
OpenAI、最新AIモデルで高度な問題解決を実現 | +2 |
ローソンが「未来のコンビニ」を発表、新技術で新時代へ | +2 |
アドビ調査、大統領選を前に誤情報対策の強化を消費者が要望 | 0 |
AIデータクラウドで企業変革を加速 | +1 |
AI活用も視野 富裕層の新投資戦略 | +1 |
AI専門家集団が企業のAIリスクを診断 | 0 |
DataStax CEO:2025年、AIが企業を変革する年になる | +2 |
Hume、低コスト&高機能の音声AI「EVI 2」発表 | +1 |
スーパーインテリジェンス競争が激化、AI時代の到来 | 0 |
Rep.aiがAI営業アバター技術で750万ドルの資金調達 | +1 |
SiFive、RISC-Vベースの新AIチップを発表 | +1 |
Magnit、AI搭載の採用支援ツール「Maggi」を発表 | +1 |
AIが切り拓く音声革命、EzAudioの挑戦 | +2 |
AI搭載ロボット、靴ひも結びに挑戦 | +1 |
AISI、AI安全性評価の新指針公開 | 0 |
AIも絡む トランプ氏著作権訴訟敗訴 | -1 |
AI恋人アプリ進化 倫理的課題も浮上 | -1 |
AI搭載「ClickUp Chat」登場 業務革新へ | +1 |
AI搭載CRM「Pulse」登場 営業革新へ | +1 |
EA、AI全面活用でゲーム開発革新へ | +2 |
MSI新AI搭載ゲーミングPC 性能進化へ | +1 |
AI新モデル o1 進化と課題の両面性 | 0 |
AI倫理の危機 GPT Store監視に不備 | -2 |
AI教育革命 元OpenAI技師が挑戦 | +2 |
AI活用で英語力急上昇 安河内氏が伝授 | +2 |
お見事!違和感なくネガポジ分析ができています。
これなら、専用のAIモデルを開発するよりも低コスト&高精度で、アンケート結果が分析できそうです。マーケターの方はぜひお試しくださいね!
【リライター】文字起こしの要約
OpenAIからは、高精度の音声文字起こしができるAIモデル「Whisper」も登場しています。こちらはOpenAI APIから有料で使えるほか、無料で使えるオープンソース版も出ていて、インタビューや会議で大活躍間違いなしの便利ツールです。そんなWhisperをChatGPTと組み合わせることで、文字起こしの要約が実現します。議事録のフォーマットに沿っての要約も可能ですので、会議の大幅効率化が見込めるでしょう。
ということで今回は、以下の動画(全22分13秒)をWhisperで文字起こししたものをChatGPTに和訳&要約してもらいます。
プロンプトは下記のとおり。それでは早速、結果をみていきましょう!
以下の文字起こしを小学生にもわかるように日本語訳&要約してください。
#文字起こし
All right. I'm Bob McGrew. I lead the research team here at OpenAI. We've just released a preview of our new series of models, O1 and O1 Mini, which we are very excited about. We've got the whole team here to tell you about them. What exactly is O1? So we're starting a series of new models with the new name O1. This is to highlight the fact that you might feel different when you use O1 as compared to previous models such as GPT-4. As others will explain later, O1 is a reasoning model. So it will think more before answering your question. We are releasing two models, O1 Preview, which is to preview what's coming for O1, and O1 Mini, which is a smaller and faster model that is trained with a similar framework as O1. So we hope you like our new naming scheme, O1. So what is reasoning anyway? So one way of thinking of reasoning is that there are times where we ask questions and we need answers immediately because they're simple questions. For example, if you ask what's the capital of Italy, you know the answer is Rome and you don't really have to think about it much. But if you wonder about a complex puzzle or you want to write really good business plan, you want to write a novel, you probably want to think about it for a while. The more you think about it, the better the outcome. So reasoning is the ability of turning thinking time into better outcomes, whatever the task you're doing. So how long have you guys been working on this? Early on at OpenAI, we were very inspired by the AlphaGo results and the potential of deep reinforcement learning. And so we were researching that heavily and we saw great scaling on Dota and robotics. And we were thinking about how can we do reinforcement learning in a general domain to get to a very capable artificial intelligence. And then we saw the amazing results of scaling and supervised learning in the GPT paradigm. And so ever since we've been thinking about how do we combine these two different paradigms into one? And it's hard to point to one exact instance where this whole effort got started. But we've had early explorations with Jacob and Shimon. We've had early explorations with Lukash, Ilya. And of course, I think one moment in time here is consolidating things with Jerry and having him build out this large scale effort here. So, I mean, it's been going on for a long time, but I think what's really cool about research is there's that aha moment. There's that particular point in time where something surprising happens and things really click together. Are there any times for you all when you had that aha moment? Like we trained GPT-2, GPT-3, GPT-4. There was the first moment when the moment was hot off the press. We started talking to the model and people were like, wow, this model is really great. And starting doing something like that. And I think that there was a certain moment in our training process where we trained, like put more compute in our L than before and trained first of all, generating coherent chains of thought. And we said, wow, this looks like something meaningfully different than before. I think for me, this is the moment. Wow. Related to that, when we think about like training a model for reasoning, one thing that immediately jumps to mind is you could have humans write out their thought process and train on that. When aha moment for me was like when we saw that if you train the model using RL to generate and hone its own chain of thoughts, it can do even better than having humans write in the thought for it. And that was an aha moment that you could really scale this and explore models reasoning that way. For a lot of the time that I've been here, we've been trying to make the models better at solving math problems, as an example. And we've put a lot of work into this and we've come up with a lot of different methods. But one thing that I kept like every time I would read these outputs from the models, I'd always be so frustrated. The model just would never seem to question what was wrong or when it was making mistakes or things like that. But one of these early O1 models when we trained it and we actually started talking to it, we started asking these questions and it was scoring higher on these math tests we were giving it. We could look at how it was reasoning and you could just see that it started to question itself and have really interesting reflection. And that was a moment for me where I was like, wow, like we've uncovered something different. This is going to be something new. And it was just like one of these coming together moments that that was really powerful. So when you read the thoughts, does it feel like you're watching a human or does it feel like you're watching a robot? It's like a spiritual experience. It's a spiritual experience, but then you can empathize with the model. You're like, oh, that's a mistake that a lot of people would make or you can see it sort of questioning common conventions. And yeah, it's spiritual, but like oddly human in its behavior. It was also pretty cool at some point when we have seen in cases where there was like a limited amount of thinking allowed for the model that just before the timeout, the model was like, I have to finish it now. And so here's the answer. I spent a lot of time doing competition math when I was young and that was really my whole reason for getting into AI was to try and automate this process. And so it's been very like a huge full circle moment for me to see the model actually be able to follow through, like very close to the same steps I would use when solving these problems. And it's not exactly the same chain of thought, I would say, but very, very relatable. It's also really cool to know it's believable that these models, they are getting on the cusp of really advancing engineering and science. And if they seem to be solving the problems, you know, maybe we can call ourselves experts, hard for us, then maybe they will be even hard for some other experts and could advance science. So we've talked a lot about some of the great moments and the times and everything just clicked. What are some of the hurdles? What are some of the places where it was actually really hard to make things work? Training large models is fundamentally a very, very hard thing to do. And there are like thousands of things that can go wrong and there are at least like hundreds that did go wrong in every training run. So I want everyone here to put a lot of blood, sweat and tears in training those things and figuring out how to keep them, continue learning and improving on a path that is actually the path of success is very narrow and the ways of failure are plentiful. It's like imagine like having the center for launching a rocket to the, let's say, some planet moon or so. And if you are off by one angle, you won't arrive at the destination. And that's our job. So the model we said is very good, oftentimes better than humans, like has equivalent of several PhDs. And that is sometimes a challenge because we have to often go and verify that the model isn't going off the rails, that we're doing something self-sensible and it started taking some serious time as we scale the model. We were saturating out all the industry grade evals and we don't know what to look for next. So that is also a challenge. I do think all of these things we ran into, it's also been one point of fulfillment. It's like every time you have a puzzle, it's like another hurdle for this team to overcome. And I'm really glad with all the little hurdles that we've overcome. So what are some of the ways you tested the models? Did you have any favorite questions that you saw the model get better at? How many Rs are in strawberries? For whatever reason, the Chagy PT wasn't able to solve this question reliably. But O-1, we did like a year and a half work, like a large number. And now we can count the number of Rs in strawberries. We should have just hard coded that rule. Reliably. I have this habit, which I think other people here do too, of whenever you go on Twitter and you see some post that's like, large language models can't do this, you copy and paste it in and then you say, confirm that our large language models can do this. To give people a sense of what they can use the model for, I'd love to hear some of the ways that you use O-1. So one way I've been using O-1 is for obviously coding. And a lot of my job is about coding. So more and more, I focus on the problem definition and use this what's called a TDD, test driven development. So instead of writing the code that implements the functionality, I focus on writing say, unit test that specify what is correct behavior of this piece of code to pass. And so because I can focus on more of that and then pass it on to O-1 to really implements I can focus on what's important, what's a high level problem to solve and so on. So this has been really an important way of shifting my focus. And another area is debugging. So now when I get some error messages, I just pass it to O-1 and then it just prints out something. Sometimes it solves right away. Even if it doesn't, it at least gives some better questions to ask and provides some ways to think about this problem better. So it has been a really important change of working for me and I hope this helps others too. I like using O-1 more and more for learning. The more I ask it's like various complex technical subjects, I find hallucinate less and explain better those concepts on previous models. For me, I like to use O-1 as like a brainstorming partner. So that can range from anything from like how to solve some very specific ML problem, machine learning problem to like how to write a blog post or a tweet. So for example, I recently wrote a blog post about language model evaluations and I was asking O-1 about ideas for the structure of the blog post, pros and cons of certain benchmarks and even the style of the writing. And I think because it's able to think before it gives the final answer, it's able to connect ideas better. It can revise and critique candidate ideas and things like that. Yeah, I think if you need like a, you know, you have some short text and you want it more creative, something really different, that's a great use to like give me five different ideas. Also, if you have just sort of like some unstructured thoughts, it's a really brilliant thought partner. So you have like some ideas, it's like, well, how should I connect these things? What am I missing? And through its final answers and through sort of reading its like thought process, it can really lead to like much better results for you. Yeah, I use it to try out a bunch of our internal secret ideas and actually tries to improve it. Yeah, for standalone projects, it's great. Like, I had to add the GitHub plugin. I know nothing about adding GitHub plugins and I just said like, hey, I want GitHub plugin that displays this and this information about the PR and like, yeah, just produce the code. I just like, like, you know, I would just ask it like, okay, so where do I need to paste this code? I don't even know. It's just like, yeah, you paste it here. I think for a lot of people, it's hard to really fill the AGI until you see the models do something better than humans can at a domain that you really care about. And I think, you know, for Go players and chess players that would have come a few years earlier and for a lot of us that like really value math and coding, I think we're starting to feel that now. I want Mams to be proud of us. So are there any parts of this project, anything that really needed to be done, but, you know, people might not realize how important it is? Yes, so I think building large-scale, reliable infrastructure for to run our biggest flagship model training grounds, as well as doing research experiments is something that is not as exciting as doing research itself, but has to be done and it has a tremendous impact on the success on the entire project. I think there is something special in OpenAI about how we structure our research in that we value algorithmic advancements in the same way as building reliable large-scale systems and building data sets that are needed either way for training those models. I'm really proud of OpenAI in that way. I think that has been a consistent pattern throughout many of our big projects. Every time we scale a new thing up, another order of magnitude, we see another host of problems, both algorithmic and infrastructure, and we've definitely built a capacity to advance them both with a lot of focus. I feel the final model is just like literally a beautiful piece of art, right? In order to make it work, we have to make sure that every step has work, right? You know, we find some any time to solve it, right? I think that's really how OpenAI operates and I'm very proud to work on here. And I also must say there's like a really not only brilliant people are here, but also kind hearted. It's just fun to me to work over here and I'm grateful to my colleagues. So, you know, code with me, per code with me, hang out with me, eat lunch with me, like speak with the model with me. So what's it like to work on the Strawberry team? You can have your brilliant ideas, but most of the time you spend on like running them and not running and failing and then it's very good to have people very close by in your office that you can ask for help with whatever failed last time because I mean most of the time you spend your time debugging things that didn't work. And having people who can help you is... Speaking of this help, we had many times when we were trying to debug this for like a week. And then passing by Wenda and then like ask it and then like he just sold it right away. He started calling it a Wenda blessing and then blessing for other people. And that has been really, really effective. And I stopped like thinking about it's too stupid to ask and just ask right away. One of the things I like really appreciate about working on OpenAI is that from every big project like this, we like really learn, right? I think like from DOTA we learn the importance of engineering, from GPT-4 we learn the importance of research and we keep iterating like this. And the effect of that is that like now the Strawberry team is again the best big research project team yet because it's built on all of the things we've learned from the previous projects. And it really like you can like really see working here like people really like started developing, being developed very good intuition. Like when do you hack something? Where do you like need to develop stronger fundamentals? Like when do you start overnight? Where do you actually like take a weekend off and like come with a fresh mind to this particular problem? Like I think like it's really amazing to observe this progress we make as a company. Yeah, one thing I've liked is just how organic this project has felt. The ideas have come literally from everywhere on this team and people feel empowered to just like, hey, here's an idea I really believe in and it's the thing that I'm going to push. And also people are just willing to get their hands dirty. I feel like there have been a lot of deadlines, some self-imposed, but we've all really come together and you know, been willing to put in that work to make it happen. This project really demonstrated the power of momentum where we get initial good results and more and more people get excited about particular field and particular research. They try to contribute their new ideas. Those new ideas work even better. And then the things started snowballing and getting more and more momentum on itself and people just believing that this is the right thing to do and we should continue pushing this research. Related to that, I think we have a very, lots of very smart people, but also like very opinionated people. But people are always willing to like update their opinions once you see results to the contrary. And I think that's like make things really fun. It's kind of cool to be in that place that's a combination of like a brilliant scientist and engineers and folks who can like build out like incredible systems. It's very humbling. So one thing I remember a few months ago, I remember the model was very smart, but it was also kind of boring. So what was it like to give the model a personality? Yeah, so that's interesting. I remember I asked model about the meaning of life and it gave me an answer 42, which is not that bad of an answer. And, you know, it's kind of similarity when I asked model, you know, what is love? It told me, oh, it is like a strange human feeling. And once we actually gave model personality, made it actually work with chat, then the answers start being quite interesting. That day, you know, I asked about the love. It told me, you know, there's like a romantic love, familial love, self-love, non-conditional love, conditional love. And it became more useful and also more fun. The funnest moment is that I ask the exact same question and you try to define love with algebra. I'm sure you ask enough nerd questions. So what's the story of O1 Mini? How did that come to be? So the motivation is that we want to bring the O1 series to broader audience with a budgetary cost. So we created O1 Mini, which was designed to be like a minimal demonstration of the whole O1 pipeline or the framework. We make it a stand reasoning specialist, which may not necessarily know the birth date of our favorite celebrity, but really truly understands like how to do reasoning effectively and truly has a lot of intelligence. The model is actually really smart. It's like much smarter than our previous best model for all and also almost on par with our best model O1, but only comes with a fraction of cost and latency. It does have the limitation that you may not know a lot of the knowledge in the outside world, which is not about science or technology, but you try to make it roughly on par with our previous best model, like for O1 Mini, and we are working to improve it further. So I'm super excited for our external users who just try it out for this lightning experience of reasoning and thinking. So what motivates you to do your research? I just find it fascinating that in this world you have these things that can do intelligence and reasoning and they're much smaller than you'd think and they can do this in different ways. It's just super fascinating. Good things in life take time and our models just tend to answer too quickly. And eventually we want to have models that can do, for example, research for months or years. And I feel like this is the first step in the direction of models that can think very long for about one problem. And now we're at the level of minutes. And I think it's just the first step on a long path that hopefully takes us to models that can think for months or years as time goes by. It feels very meaningful that I, together with a small number of people, can have some substantial positive impact on the world. And also it's fun. Day to day it's just fun. I like, you know, speaking to the computer. I like starting a job on the cluster. I very much enjoy collaboration. It's just beautiful. I really like our models to be useful. And I think technology has a chance and a promise to improve human life. And I like our models to do work for us, to help us with our day to day problems and giving them ability to reason. It allows them to do for us things that they just couldn't before that will allow us to spend our time more productively. Yeah, I'm very excited about this. I mean, I think these sort of paradigms unlock things that the models couldn't do before. So it's not just like answering some sets of queries a little bit better, but it's actually getting to a point where through planning, through error correction, it's able to just unlock like new capabilities and the ability to produce new knowledge in the world for like science, for discovery, I think is one of the most exciting pieces for this. And I think in some short amount of time, it's going to become like a larger and larger contribution or contributor to its own development. And I think that's like a really exciting regime. I think some of the people on this team, we were math or coding Olympiad participants in the past. And there is this huge personal motivation to create a system that can beat us and do best. And I think the second thing really kind of echoes the point that JT and Leo made. You know, I do think reasoning is a much more powerful primitive than people give it credit for. You know, when you think about kind of accomplishing tasks reliably, really that fundamental primitive has to be reasoning. You're going to hit bottlenecks and you're going to have to navigate your way around them. So I'm really excited for that. I think AI researchers job is to find the way to put more compute in. And hardware people have been doing so good of a job that the cost has been going down exponentially for a very long time. And we don't have much time to find another way to put in more compute. And it's kind of like a weight on my shoulders is getting larger and larger. And this new paradigm really finds a way to unload that for probably a long time. Is there anything else you've observed as we've been going through this project for the whole time we've been doing it? Anything else that's worth calling out? I think an interesting meta observation that we've had is every model that we train is like a little bit different. It has its own quirks and it's almost like artisanal. Because when you look at a model that can do so many different tasks, each model you train won't be exactly the same performance at each task. So it might be better at some tasks and worse at others. And so there's this uniqueness or like personality to every model that is almost like a little bit beautiful. Thank you and congrats on releasing this.
#要約
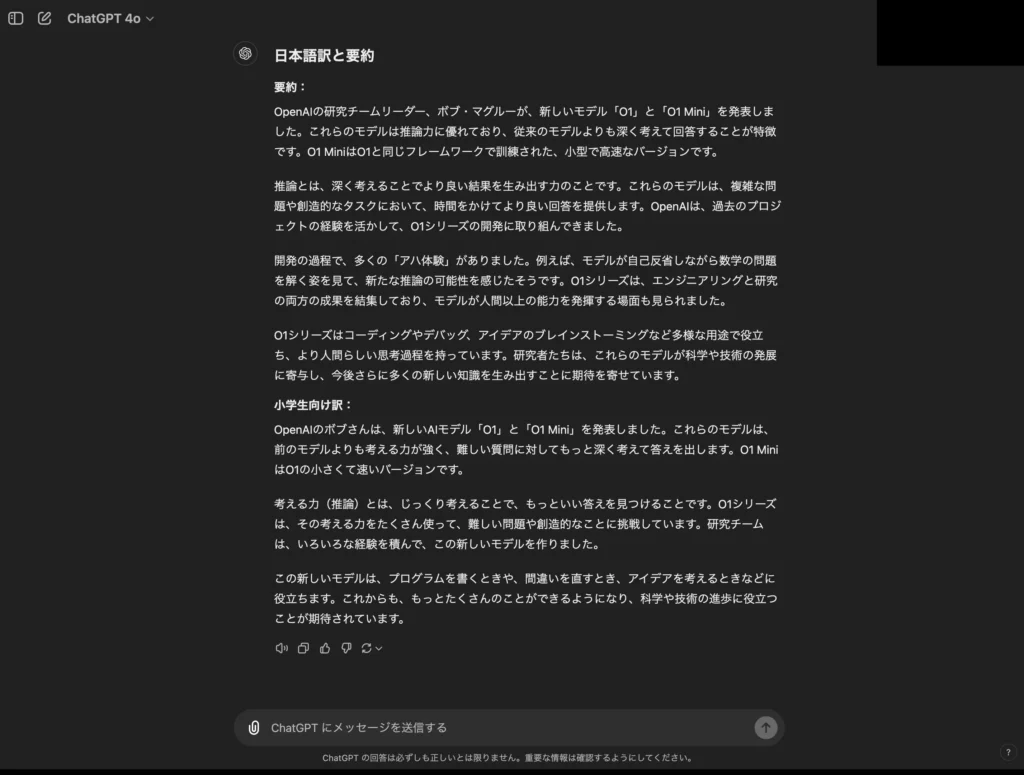
このように、ChatGPTはOpenAI o1の開発秘話を程よい長さで要約してくれました。これなら、海外の動画コンテンツも手軽にキャッチアップできそうですね。
なお、ChatGPTのハック術が知りたい方は、以下の記事を参考にしてみてください。
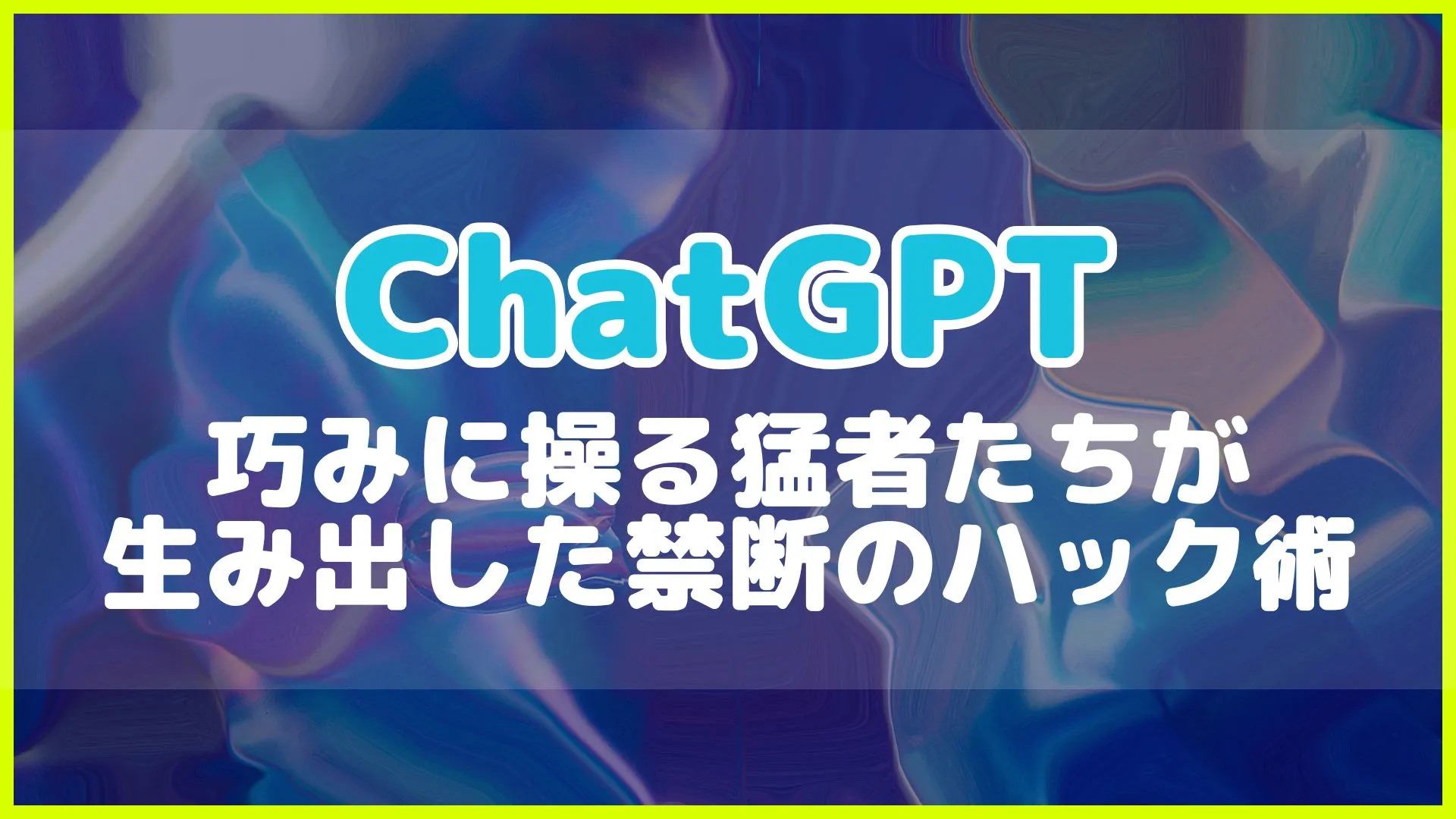
ChatGPTを使うときの注意点
ChatGPTは、日常生活やビジネスなどさまざまなシーンで使える非常に便利なAIツールですが、使う際には注意しなければならない点もあります。
主に以下の3つのことに注意が必要です。
- 情報漏えいのリスク
- 誤情報を出力することがある
- 著作権を侵害する可能性
1つずつ解説します。
情報漏えいのリスク
ChatGPTの利用には、情報漏えいのリスクが伴います。
ChatGPTの提供元であるOpenAIの利用規約では、ユーザーの入力した内容はChatGPTの学習データとして使われる可能性があることが明記されています。
ChatGPTのモデル学習に使用されただけであれば、第三者に情報を悪用される可能性は少ないかもしれませんが、ChatGPTは学習した情報をほかのユーザーへの回答に利用する可能性もある点に注意が必要です。
また、近年ではサイバー攻撃によるデータベースの侵害も増加しており、サイバー攻撃によってユーザーの情報や会話履歴が盗まれる危険もあります。
ユーザーはあらかじめ情報漏えいのリスクを理解し、個人情報や機密情報など重要な情報は入力しないように気を付けましょう。
入力した内容がモデル学習に使用されることを望まない場合、オプトアウト(使用停止)を設定することもできます。※1
誤情報を出力することがある
ChatGPTの回答は、100%正しいとは限りません。
ChatGPTをはじめとした生成AIには、AIが実際には存在しない情報をあたかも事実かのように回答する現象(ハルシネーション)が起こる場合があります。
これは、AIがユーザーの質問の意図を正しく解釈できなかった場合に、それらしい情報と関連付けて回答してしまうことが原因です。
アップデートを重ねるごとにハルシネーションの確率は下がりつつありますが、それでもまだ100%信用はできません。現時点での生成AIの限界を理解して、複数の生成AIを使う、自身でダブルチェックを行うなどの対策を取りましょう。
著作権を侵害する可能性
ChatGPTで生成したコンテンツの著作権は原則利用者に帰属しますが、既存のコンテンツと類似・酷似しているものを商用利用した場合には、著作権侵害が疑われる可能性があります。
ChatGPTはインターネット上のデータを学習しているため、既存コンテンツに類似したものを出力する可能性は十分にあり得ます。
2018年に改正された日本の著作権法(第30条の4第3号)では、機械学習で利用される著作物については原則として著作権侵害にはならないとされています。つまり、商用利用を目的としなければ、ChatGPTを含む生成AIによって作られた制作物に元データの著作権は及ばないということです。
ただし、これはあくまで日本の法律が及ぶ範囲に限られていることを念頭に置いてください。ChatGPTを利用する際は、既存コンテンツとの類似性を人の目やチェックツールなどで必ず確認し、著作権侵害に当たらないかを注意する必要があります。
プロンプト次第で文章以外にも使えるChatGPT
当記事では、ChatGPTのプロンプトについて基本の型とデモンストレーションをお届けしました。以下にてもう一度、ChatGPT×プロンプトでできることをお見せします。
- 文章作成
- 文章要約
- 文章添削
- 要素の抽出
- アイデア出し
- 翻訳
- 条件分岐・意思決定
- コーディング
- フレームワークに基づく思考(SWOT分析やジョブ理論)
- 定性的なものの定量評価(感情分析)
ChatGPTはプロンプト次第で、文章生成・調べ物・プログラミング以外にも活躍してくれます。なかでもデータの加工は得意分野で、CSVやHTMLファイルを作成したり、感情分析を行ったりといった用途に最適です。
ほかには、文字起こしAI「Whisper」との組み合わせで議事録作成の自動化も可能。日々の雑務を大幅に効率化できてしまいます。
今までChatGPTを使ったことがない方、文章生成しか試したことがない方はぜひぜひ、他のタスクでもChatGPTをフル活用してみてくださいね!
最後に
いかがだったでしょうか?
弊社では、
・マーケティングやエンジニアリングなどの専門知識を学習させたAI社員の開発
・要件定義・業務フロー作成を80%自動化できる自律型AIエージェントの開発
・生成AIとRPAを組み合わせた業務自動化ツールの開発
・社内人事業務を99%自動化できるAIツールの開発
・ハルシネーション対策AIツールの開発
・自社専用のAIチャットボットの開発
などの開発実績がございます。
まずは、「無料相談」にてご相談を承っておりますので、ご興味がある方はぜひご連絡ください。
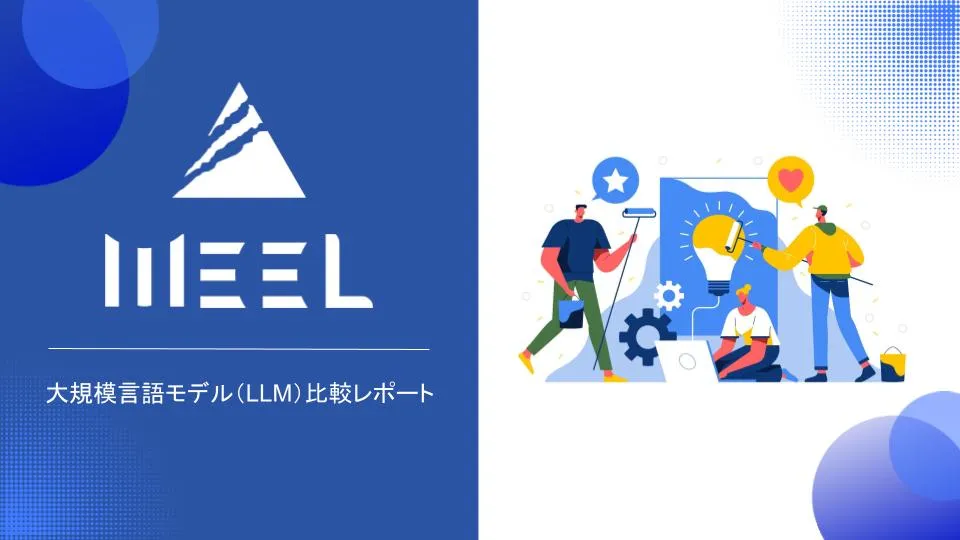
「生成AIを社内で活用したい」「生成AIの事業をやっていきたい」という方に向けて、生成AI社内セミナー・勉強会をさせていただいております。
セミナー内容や料金については、ご相談ください。
また、大規模言語モデル(LLM)を対象に、言語理解能力、生成能力、応答速度の各側面について比較・検証した資料も配布しております。この機会にぜひご活用ください。